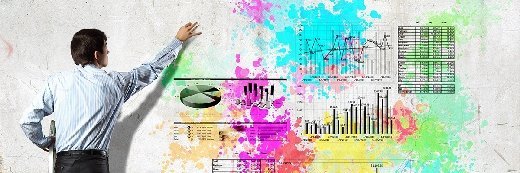
Sergey Nivens - Fotolia
IoT data quality determines project profitability
The insights drawn from IoT data analysis can only improve with better IoT data quality, which data scientists measure with metrics such as accuracy, timeliness and completeness.
IoT data quality matters, perhaps even more than the quantity of it, because the data generated by endpoint sensors drives decisions and determines actions.
Connected devices generate zettabytes of data each year, and the volume is set to triple in the upcoming few years. There will be 55.7 billion connected devices worldwide by 2025, with 75% of them connected to an IoT platform, according to research firm IDC. Those devices will generate an expected 73.1 zettabytes of data by 2025, up from 18.3 ZB in 2019.
The numbers are impressive, but how much of that data is any good? Each organization's answer will vary based on how they're using that data.
"You can't have good decisions with bad data. You can't have high-quality decisions without quality data, and you can't have good outcomes without high-quality decisions," said Shawn Chandler, CTO at GridCure and IEEE senior member.
IoT engineers and executives must ensure they develop a strong data governance program for their IoT deployments, a process that considers the necessary data quality measures and how to ensure those measures are met and maintained. Any decisions or predictions based on data that doesn't meet a determined standard will be flawed and can cost an organization when predictions skew in the wrong direction.
How do you measure data quality?
Data scientists can assess data quality by measuring objective features or dimensions, such as accuracy, record completeness, data set completeness, integrity, timeliness, uniqueness, consistency, precision, accessibility and existence. Subjective qualities might also include usability, believability, interpretability and objectivity.
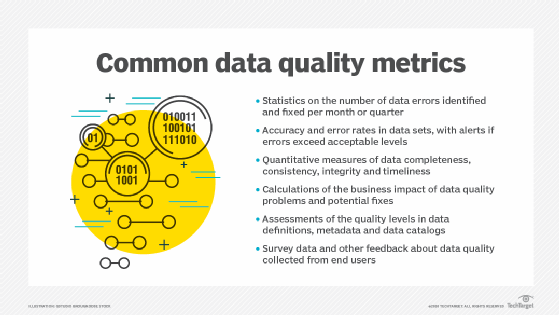
"Data should be determined to be 'fit for purpose' or not, and with a specified range or tolerance. That is, it's highly contextual. Just like, say, a diamond. We can measure its cut, color, carats and clarity. But these matter differently for a diamond engagement ring versus a diamond sawblade," said Doug Laney, data and analytics strategy innovation fellow at business and technology consulting firm West Monroe and author of the book Infonomics: How to Monetize, Manage, and Measure Information as an Asset for Competitive Advantage.
Each organization must evaluate its data and determine the level of quality it needs for each IoT use case. In other words, organizations won't find a one-size-fits-all rule to follow. The parameters of data quality dimensions vary from one organization to the next, based on each enterprise's needs and the decisions being made with IoT-generated data.
"Organizations should understand the data quality tolerances for accuracy, time and completeness needed for a particular application," said Mike Gualtieri, vice president and principal analyst at Forrester Research. "Accuracy for [a grocery store] freezer may not have to be as precise as say the temperature to keep an industrial chemical in gaseous state."
What can influence IoT data quality?
Engineers can alter the data quality at multiple points within the IoT data pipeline.
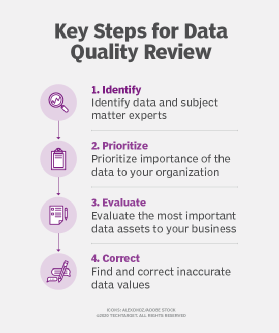
Endpoint devices might create issues themselves. If devices are not reliable or properly calibrated, the data they collect may not be an accurate or precise measure of reality.
"IoT devices are prone to miscalibration or communication issues that skew data or introduce availability and completeness issues," Laney explained, noting that such issues "are easy enough to test for and resolve."
Device deployment can also cause issues. For example, improperly placed or programmed endpoint devices may take in data that's not complete or relevant. A sensor intended to measure vibrations on a highway bridge that was programmed to only take readings at times when traffic flow tends to be low doesn't produce the full scope of data likely needed.
Problems along the IoT pipeline, such as security breaches or formatting changes, could also affect data quality.
Strong governance required
Organizations must create a data plan to address the quality dimensions needed.
A strong data quality governance program starts with teams identifying the data required based on organizational objectives and then determining what data quality dimensions to use. The intended use determines the parameters of those dimensions -- in other words, how accurate, complete and timely the data quality must be for the decisions being made.
"Data quality is a spectrum, and the methods and means used to collect, collate, store and analyze the data will vary across that spectrum. Understanding the nature of the data collected and the purpose for which it will be used will define the shape of the solution used to ensure the quality fits the purpose," said Simon Ratcliffe, principal consultant at the IT service management company Ensono.
For example, the data from endpoint devices that steer an autonomous vehicle has much higher data quality requirements than an IoT deployment being used to drive efficiencies in a building's heating system.
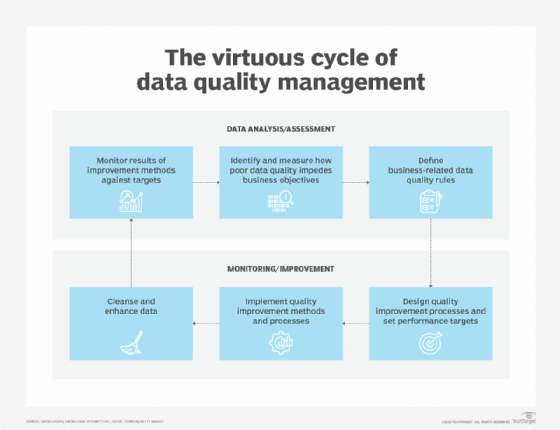
A governance program also must establish mechanisms to review the data and confirm that it continues to meet the dimension parameters required by the organization throughout the lifecycle of the deployment and accounts for the evolution of the IoT use cases.
"Data governance involves an entire operating model around the principles, guidelines, policies, practices, procedures, monitoring and enforcement of data's collection, generation, handling, usage and disposition," Laney added. "Ideally, data governance should be baked-into the design of systems that generate data, not be an afterthought."