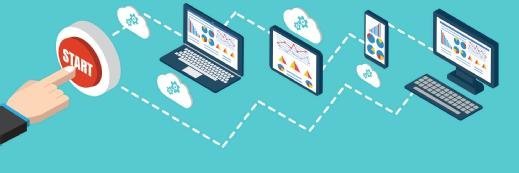
Seraphim Vector - Fotolia
Reach business objectives with the right IoT data pipeline
IoT technology creates value from its data. Organizations need the right IoT data pipeline to facilitate the processing that leads to actionable insights and informs business decisions.
Organizations must collect, move, analyze and store vast volumes of endpoint device data to achieve IoT business objectives, and the IoT data pipeline controls whether each step goes smoothly.
The IoT data pipeline is the technology stack that handles all data -- including data collection, aggregation and analysis -- while it moves from the connected endpoint device to centralized analytics or storage. The right components determine how efficiently the data reaches the desired results.
Every organization that collects data from connected devices has data pipelines, even if they don't use that specific terminology. The data pipeline is another label for the extract, transform and load process, said Robert Schmid, chief IoT technologist at Deloitte Digital. Still others refer to the IoT data pipeline as IoT data management services.
The IoT data pipeline complicates data processing compared to data pipelines from other sources because of IoT-specific challenges, such as interoperability, and the massive data volumes.
IoT data pipeline explained
A pipeline starts with captured data from the connected endpoint device, and the data moves through processing, transference, ingestion, validation, storage, analysis and learning, said Geoff Mulligan, an IEEE member and founder of the consultancy Skylight Digital.
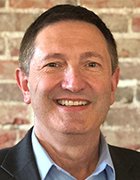
The configuration of those eight steps varies based on IoT uses and enterprise objectives.
"In many cases, what could be called the 'IoT data pipeline' is quite short with desired real-time and contextual insights, responses driving far-edge-based aggregation and compute without or with little data transmission to more centralized servers," said Chris Rommel, executive vice president of IoT and industry technology at VDC Research Group, a technology market intelligence and consulting firm.
An organization that captures video may analyze the images at the edge to avoid the cost and latency of moving data to the cloud. Only analyzed results move to central servers. Alternatively, an organization may move all data from endpoint thermometers to central servers if the organization needs a full record of temperature control adherence for safety reasons.
IoT data pipeline components
The data pipeline requires a collection of software and hardware to receive and deliver data. Pipeline components include the:
- data ingestion layer;
- data integration layer;
- data processing layer;
- data streaming or streaming analytics layer;
- data storage layer; and
- data visualization layer.
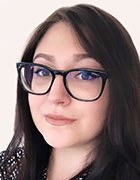
The full technology stack components can include hardware-agnostic SaaS or PaaS for analytics. Organizations also have a multitude of storage options, such as deep storage, in-memory data grid, in-memory database, data warehouses, data lakes and data rivers, said ABI Research analyst Kateryna Dubrova.
Organizations with more mature IoT deployments and strategies require a more sophisticated data pipeline to move and analyze data and to use the intelligence gained for real-time decision-making, Dubrova said. SMBs beginning their IoT journey still widely accept applied business intelligence models. Large manufacturing organizations, customer-centric firms and IoT-enabled enterprises require more sophisticated, augmented and constructive models to extract value from IoT data.
Organizations want to use data automation and real-time corrective action for data-driven decision-making that exceeds many existing data ingestion and analytics offerings. Some vendors have adapted their offerings to create seamless and automated data-processing mechanisms, Dubrova said.
"Alongside this, there is an emergence and democratization of [machine learning] algorithms and AI technologies, which vendors are offering as-a-service in the cloud space to consolidate data sources and enable automation of the data transformation process," Dubrova said.
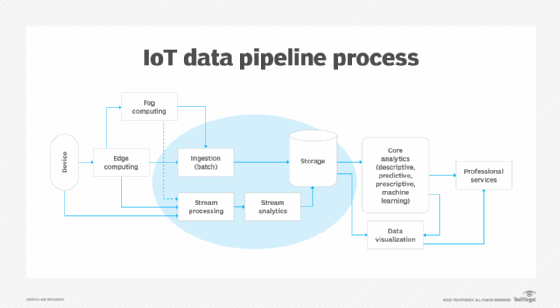
IoT-specific services from cloud providers include Azure's IoT Hub, AWS IoT Core and Google Cloud IoT Core. Other vendors provide various IoT data pipeline products and services, some open source, such as Apache, ClearBlade, IBM, KX Technologies, MongoDB, Oracle, Pandio, PostgreSQL, StreamSets, Striim, ScaleOut Software and Tibco.
"Despite deconstruction of the stream processing and streaming analytics functionalities within the value chain, most vendors view them as a bundled offer and do not offer separate services for processing streams and streaming analytics or for ingestion, integration and batch processing capabilities," Dubrova said.
A strategic start to build an IoT data pipeline
Organizations with effective data pipelines build them to align with strategic IoT objectives. Deployed endpoint devices collect data that the organization uses for their specified goals.
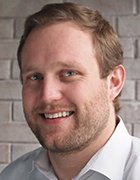
When CIOs examine their data pipelines, they must identify their IoT use cases and what they want to achieve.
"Before undertaking any IoT deployments, organizations must develop a firm understanding of their business goals. IoT goals could be for operations optimization, service delivery, client value-add or for future product development insights," Rommel said.
The business goal informs the pipeline design, including:
- which endpoint devices to use;
- what data to collect;
- where to analyze the data;
- what actions analysis drives; and
- how much generated data will be stored, where and for how long.
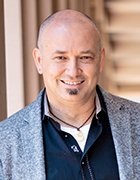
This work requires a multidisciplinary team, including IT, a security person, someone who really understands data storage and data processing, and someone who knows AI and machine learning, Schmid said.
CIOs must carefully weigh their choices and consider whether to use cloud-native tools, Mulligan said.
"There's a quick rush to use cloud-native tools, but if, somewhere down the line, the company decides they want to move from, for example, AWS to Azure … suddenly their entire data pipeline needs to be rewritten," Mulligan said.
Organizations must pursue cloud-agnostic architecture and use open standards and APIs where possible, as well as Agile development principles to better prepare for future industry changes.