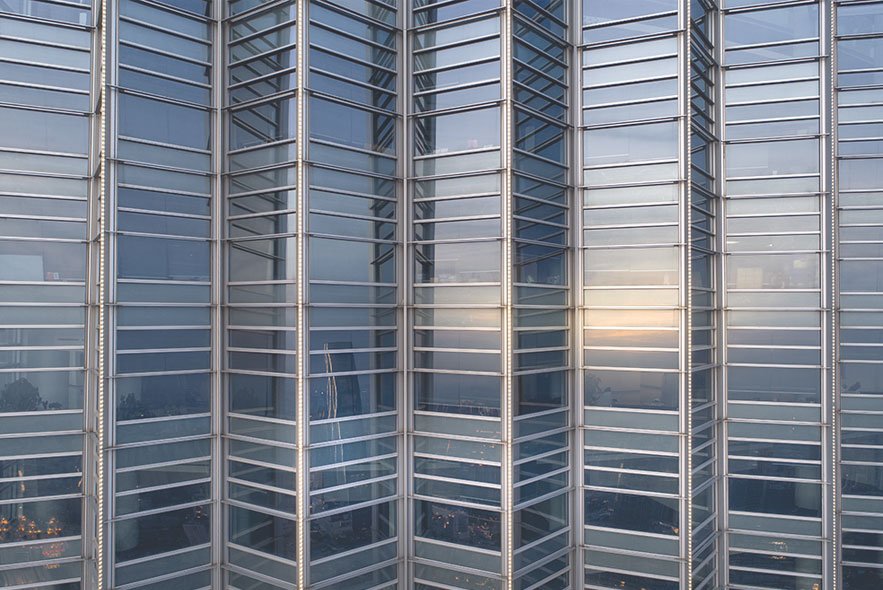
data pipeline
What is a data pipeline?
A data pipeline is a set of network connections and processing steps that moves data from a source system to a target location and transforms it for planned business uses. Data pipelines are commonly set up to deliver data to end users for analysis, but they can also feed data from one system to another as part of operational applications.
As more and more companies seek to integrate data and analytics into their business operations, the role and importance of data pipelines are equally growing. Organizations can have thousands of data pipelines that perform data movements from source systems to target systems and applications. With so many pipelines, it's important to simplify them as much as possible to reduce management complexity.
To effectively support data pipelines, organizations require the following components:
- A GUI-based specification and development environment that can be used to define, build and test data pipelines, with version control capabilities for maintaining a library of pipelines.
- A data pipeline monitoring application that helps users monitor, manage and troubleshoot pipelines.
- Data pipeline development, maintenance and management processes that treat pipelines as specialized software assets.
What is the purpose of a data pipeline?
The data pipeline is a key element in the overall data management process. Its purpose is to automate and scale repetitive data flows and associated data collection, transformation and integration tasks. A properly constructed data pipeline can accelerate the processing that's required as data is gathered, cleansed, filtered, enriched and moved to downstream systems and applications.
Well-designed pipelines also enable organizations to take advantage of big data assets that often include large amounts of structured, unstructured and semistructured data. In many cases, some of that is real-time data generated and updated on an ongoing basis. As the volume, variety and velocity of data continue to grow in big data systems, the need for data pipelines that can linearly scale -- whether in on-premises, cloud or hybrid cloud environments -- is becoming increasingly critical to analytics initiatives and business operations.
Who needs a data pipeline?
A data pipeline is needed for any analytics application or business process that requires regular aggregation, cleansing, transformation and distribution of data to downstream data consumers. Typical data pipeline users include the following:
- Data scientists and other members of data science teams.
- Business intelligence (BI) analysts and developers.
- Business analysts.
- Senior management and other business executives.
- Marketing and sales teams.
- Operational workers.
To make it easier for business users to access relevant data, pipelines can also be used to feed it into BI dashboards and reports, as well as operational monitoring and alerting systems.
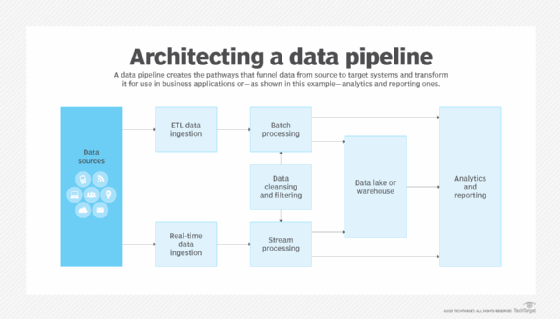
How does a data pipeline work?
The data pipeline development process starts by defining what, where and how data is generated or collected. That includes capturing source system characteristics, such as data formats, data structures, data schemas and data definitions -- information that's needed to plan and build a pipeline. Once it's in place, the data pipeline typically involves the following steps:
- Data ingestion. Raw data from one or more source systems is ingested into the data pipeline. Depending on the data set, data ingestion can be done in batch or real-time mode.
- Data integration. If multiple data sets are being pulled into the pipeline for use in analytics or operational applications, they need to be combined through data integration processes.
- Data cleansing. For most applications, data quality management measures are applied to the raw data in the pipeline to ensure that it's clean, accurate and consistent.
- Data filtering. Data sets are commonly filtered to remove data that isn't needed for the particular applications the pipeline was built to support.
- Data transformation. The data is modified as needed for the planned applications. Examples of data transformation methods include aggregation, generalization, reduction and smoothing.
- Data enrichment. In some cases, data sets are augmented and enriched as part of the pipeline through the addition of more data elements required for applications.
- Data validation. The finalized data is checked to confirm that it is valid and fully meets the application requirements.
- Data loading. For BI and analytics applications, the data is loaded into a data store so it can be accessed by users. Typically, that's a data warehouse, a data lake or a data lakehouse, which combines elements of the other two platforms.
Many data pipelines also apply machine learning and neural network algorithms to create more advanced data transformations and enrichments. This includes segmentation, regression analysis, clustering and the creation of advanced indices and propensity scores.
In addition, logic and algorithms can be built into a data pipeline to add intelligence.
As machine learning -- and, especially, automated machine learning (AutoML) -- processes become more prevalent, data pipelines likely will become increasingly intelligent. With these processes, intelligent data pipelines could continuously learn and adapt based on the characteristics of source systems, required data transformations and enrichments, and evolving business and application requirements.
What are the different types of data pipeline architectures?
These are the primary operating modes for a data pipeline architecture:
- Batch. Batch processing in a data pipeline is most useful when an organization wants to move large volumes of data at a regularly scheduled interval and immediate delivery to end users or business applications isn't required. For example, a batch architecture might be useful for integrating marketing data into a data lake for analysis by data scientists.
- Real time or streaming. Real-time or streaming data processing is useful when data is being collected from a streaming source, such as financial markets or internet of things (IoT) devices. A data pipeline built for real-time processing captures data from the source systems and quickly transforms it as needed before sending the data to downstream users or applications.
- Lambda architecture. This type of architecture combines batch and real-time processing in a single data pipeline. While more complicated to design and implement, it can be particularly useful in big data environments that include different kinds of analytics applications.
Event-driven processing can also be useful in a data pipeline when a predetermined event occurs on the source system that triggers an urgent action, such as a fraud detection alert at a credit card company. When the predetermined event occurs, the data pipeline extracts the required data and transfers it to designated users or another system.
Key technologies used in data pipelines
Data pipelines commonly require the following technologies:
- Extract, transform and load (ETL) is the batch process of copying data from one or more source systems into a target system. ETL software can be used to integrate multiple data sets and perform rudimentary data transformations, such as filtering, aggregations, sampling and calculations of averages.
- Other types of data integration tools are also often used to extract, consolidate and transform data. That includes extract, load and transform (ELT) tools, which reverse the second and third steps of the ETL process, and change data capture software that supports real-time integration. All of these integration tools can be used together with related data management, data governance and data quality tools.
- Data streaming platforms support real-time data ingestion and processing operations, often involving large amounts of data.
- SQL is a domain-specific programming language that's often used in data pipelines. It's primarily designed for managing data stored in relational databases or stream processing applications involving relational data.
- Scripting languages are also used to automate the execution of tasks in data pipelines.
Open source tools are becoming more prevalent in data pipelines. They're most useful when an organization needs a low-cost alternative to a commercial product. Open source software can also be beneficial when an organization has the specialized expertise to develop or extend the tool for its processing purposes.
What is the difference between an ETL pipeline and a data pipeline?
An ETL pipeline refers to a set of integration-related batch processes that run on a scheduled basis. ETL jobs extract data from one or more systems, do basic data transformations and load the data into a repository for analytics or operational uses.
A data pipeline, on the other hand, involves a more advanced set of data processing activities for filtering, transforming and enriching data to meet user needs. As mentioned above, a data pipeline can handle batch processing but also run in real-time mode, either with streaming data or triggered by a predetermined rule or set of conditions. As a result, an ETL pipeline can be seen as one form of a data pipeline.
Data pipeline best practices
Many data pipelines are built by data engineers or big data engineers. To create effective pipelines, it's critical that they develop their soft skills -- meaning their interpersonal and communication skills. This will help them collaborate with data scientists, other analysts and business stakeholders to identify user requirements and the data that's needed to meet them before launching a data pipeline development project. Such skills are also necessary for ongoing conversations to prioritize new development plans and manage existing data pipelines.
Other best practices on data pipelines include the following:
- Manage the development of a data pipeline as a project, with defined goals and delivery dates.
- Document data lineage information so the history, technical attributes and business meaning of data can be understood.
- Ensure that the proper context of data is maintained as it's transformed in a pipeline.
- Create reusable processes or templates for data pipeline steps to streamline development.
- Avoid scope creep that can complicate pipeline projects and create unrealistic expectations among users.