Gartner's AI 'value chain' offers schema for assessing an AI project
Analyst Bern Elliot encourages CIOs to approach AI the way a financial advisor approaches an investment portfolio -- with a mix of high-risk and low-risk projects.
Gartner analyst Bern Elliot said enterprise AI projects should start with objectives and use cases, but they should also start with a good dose of reality.
For any AI project, CIOs will need to:
- Map out the underlying technologies needed to produce a return on investment.
- Assess the quality of their data and determine if it's clean enough to produce a functional model.
- Determine if their organizations have the technical skills needed to get an AI project started.
That's not all. Elliot suggested that unless the company is ready to move quickly on AI, CIOs need to think like a financial advisor. Instead of betting it all on one AI project, CIOs should invest in a portfolio of projects to reap the benefits of AI -- one that mixes low-risk, low-reward projects with those that are high risk, high reward.
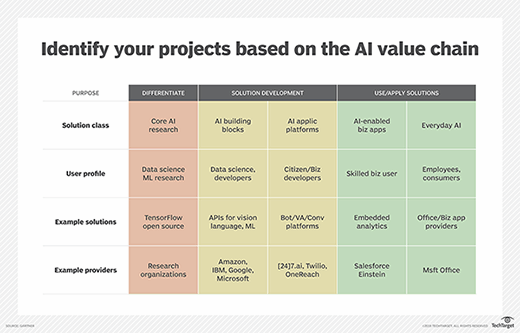
The AI value chain
Not all AI projects are created equal. Some can provide incremental improvements such as using a virtual assistant to help with customer service. Applications like these don't provide a competitive differentiation, but "are good places to start on your AI project," Elliot said during a recent Gartner webinar, "A Framework for Applying AI in the Enterprise."
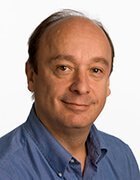
Other AI projects can be more strategic in nature, where the enterprise seeks out a competitive advantage. "It often involves specific data that you have access to and that is unique to your operation," he said. A strategic AI project such as a healthcare organization deploying conversational patient assistants to improve care will require more time, more skills and more experimentation than incremental improvement AI projects.
Elliot said one way to visualize an AI project's level of difficulty is with Gartner's AI "value chain," a chart that lays out the purpose of an AI project against its complexity, needed expertise, project examples and technologies.
On one side of the value chain is a category called "core AI research," which requires data science and machine learning expertise and is often carried out at research institutions. On the other side is deploying packaged AI solutions that are embedded into enterprise applications such as Salesforce Einstein or Microsoft Office.
In between are additional categories that include solution development, which Elliot said required a good amount of technology expertise and significant resources. There are two types of solution development. The more complex of the two is often characterized by large organizations using APIs from companies such as Google or Microsoft to explore how specific algorithms such as computer vision, which enables a machine to see, might be used.
The less complex of the two is to utilize AI application platforms, which provide "drag and drop" environments to enable enterprise architects to put together, say, a chatbot or a virtual assistant, which can understand language and help users complete tasks. "This is the most active market we're seeing right now -- companies offering platforms to let enterprise developers build their solutions," he said.
As CIOs move across the chart from left to right, the risks, the time, the costs and the necessary skills needed to get the project off the ground to achieve an objective decrease -- as does the potential for strategic differentiation, according to Elliot.
Think portfolio
Gartner does not recommend pulling the trigger on just one kind of AI project. Instead, CIOs should think about AI projects the way they think about an investment portfolio.
"If you're going to be investing a million dollars in the stock market, you wouldn't put it all on one long-sure bet -- or at least you probably wouldn't," Elliot said. "What you'd want to do is start out with a more balanced approach and, in fact, start out with investments that may have less potential for growth but also have less downside for risk."
In the beginning, CIOs should invest more on the right side of the chart. As they gain experience and expertise, the investment will shift to the higher risk projects found on the left side of the chart.
"If you start with some projects that are further to the right and are easier to execute, then you get the experience, you develop your corporate skills overall, and you develop credibility so that you can get funding more easily," he said.
Key questions to ask
Elliot said two key questions can help CIOs determine where the project falls on the AI value chain. First, is there a sufficient amount of clean data? "It's good data if it's sufficient to develop a model that is accurate in predicting what you would like to predict," he said.
Second, are there off-the-shelf toolkits available? "If the answer to both of these questions is yes for that particular use case, then that would be a low-lying fruit, a green project," he said. "It would be a better project, a safer project for you to start out on."
If an off-the-shelf tool isn't available but the data is, CIOs should think of the project as intermediate in complexity, perhaps as a project that can help the organization develop application expertise in-house. If the data isn't good, CIOs should consider the project risky and worth waiting on while the skills catch up to the use cases.
For companies that have a modest adoption profile, they'll start with the easiest projects and move forward over the next couple of years. Companies with a more advanced adoption profile should establish a roadmap and move more aggressively from simple to complex projects over the next year or two, according to Elliot.