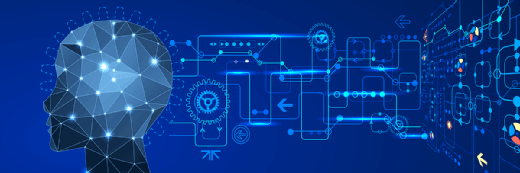
Davenport: AI-based projects should be focused in scope
New AI projects in enterprises should focus on achievable goals rather than try to reinvent business processes or products and services, Tom Davenport says.
Tom Davenport was an early proponent of big data and analytics. The Babson professor and consultant literally wrote the book on how businesses should use data. His popular 2007 volume, Competing on Analytics, brought the idea of data as a competitive advantage to many business leaders for the time.
So, you might expect Davenport to be optimistic about the latest trend in analytics, AI. However, this time around, he's recommending a more measured approach. In his new book, The AI Advantage, Davenport recommended enterprises avoid AI moonshots and instead look to advance AI more incrementally. While he sees AI as a powerful technology for enterprises, he doesn't think it's likely to have the immediately profound impact some predict.
In this Q&A, we talked with Davenport about how he defines AI technology and how enterprises should select the best new AI-based projects for their needs.
For the purposes of your book and when you talk about AI, what specifically are you thinking about?
Tom Davenport: Well, I'm pretty inclusive, and I believe that the simplest forms of machine learning are basically predictive analytics. But I think it's very difficult to draw the line and say, 'Well, if it's logistic regression, it's analytics, and if it's gradient-boosted analysis, then it's AI.' So, I put machine learning in the category of AI, and I put in all the kind of language-oriented things: natural language understanding, which most people believe is AI, natural language generation, [which] some people say is not AI. But I do include it because I think it's hard to draw the line. And I also put in robotic process automation [RPA], which I think is not that intelligent on its own but more and more people are adding machine learning to it. And it is about automation, so I just throw it in one big bucket.
How important do you think it is to get to some sort of definition? It sounds like you have a pretty broad notion of what you would include as AI. Do you think there's any real value in setting out some sort of firm definition of the term?
Davenport: I've been around for a while, and I did knowledge management for a decade or so, and people really wanted to talk about definitions -- when is it knowledge? when is it information for data? -- and it just seemed like medieval philosophy to me to talk about when something becomes knowledge, so I kind of feel the same way about AI. If you want to call RPA something different than AI, then that's fine with me, but I don't think it does harm to put it in the AI category. As I always used to say with knowledge management, you're trying to add more value to it. I think in AI you're trying to add more intelligence to it to make it more useful and smart, but the exact point at which it becomes intelligent enough to call it AI I think is pretty academic, and I'm an academic.
In your book, you argue that enterprises should be looking at more modest AI-based projects rather than the big moonshot initiatives. Why?
Davenport: I have seen a number of these moonshots fail, and I started out talking about that MD Anderson failure with Watson. And then another Watson failure at DBS Bank in Singapore. And then I talk about Amazon. The drone delivery project is a moonshot, and you could argue that Amazon Go, their convenience store, is too. Those, I think, are moonshots, but [Amazon founder and CEO Jeff] Bezos said [in his 2017 letter to shareholders] the great bulk of what they do is -- I think his term was "quietly but meaningfully improving core operations." And even Google, by 2016, had 2,700 projects with AI being a component of them, and obviously, the vast majority of those are pretty incremental kinds of changes, at least as far as they're visible to an outside observer.
So, I just think projects are far more likely to succeed if they're less ambitious, and AI tends to be well-suited to less ambitious projects in a way because it really only supports individual tasks. And to really have a dramatic impact, you'd have to do something for an entire process or at least a whole job, and that's not often the case with AI. You'd have to stream together multiple projects to do it. For the vast majority of organizations that aren't as technologically astute as Google or Amazon, it probably makes sense to do zero moonshots.
Is that primarily because, as you were just saying, the more typical enterprise doesn't have access to the type of skills that more advanced companies like Google have, or is it that the technology itself is not quite mature enough? Why specifically do you think these bigger moonshots in AI-based projects aren't really paying off?
Davenport: I think it's a combination of the fact that the technologies aren't fully mature. Certainly, that's what Dave Gledhill, the CIO and head of operations at DBS Bank, said about Watson. It was early days, and even still, I think it's incredibly hard to do these big things like cure cancer or figure out what's the ideal stock for a customer to recommend, which is what they were trying to do at DBS -- or have autonomous drones deliver packages. So, unless you're willing to take a lot of risk, I think it's probably not a good idea. The systems are quite narrow, and they only do a relatively narrow task, and moonshots are by definition somewhat broader.
And we don't have a great history with high expectations about AI. We've gone through several AI winters, I think, as a result of expectations being dashed a bit. So, better to be a little bit more conservative and not set ourselves back with big failures.
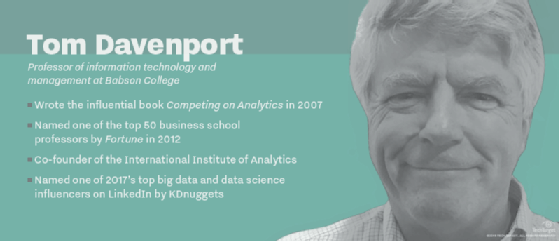
At the same time, though, do you think there is any risk to having a scaled-down mindset and missing out on the next big innovation in your AI-based projects? Is there any risk to taking too small of an approach to AI right now and missing a chance to gain a competitive advantage with something more transformative?
Davenport: I do think that, if you want to really accomplish something dramatic, then fine -- undertake a bunch of related projects at a particular area of your business. And I think you might have some success with that. But each individual project would be relatively small. If you want to be really ambitious, I don't think there's any great risk in dividing up AI into a set of smaller projects. It's kind of like autonomous vehicles. I was talking to a woman who used to be head of machine learning at Carnegie Mellon, and she just went to JPMorgan Chase, Manuela Veloso, and she said, when she came to CMU in the mid-80s, everybody was saying: 'Autonomous vehicles are just around the corner. We have prototypes in the lab, etc.' That was 30 years ago, and now we're still just around the corner with it.
So, there are a lot of different components that have to work together, and it's hard, like with autonomous vehicles, to get the existing ways of doing work out of the way immediately. You have to put in these things incrementally. So, I think the biggest risk is that people may see that some of the hype is not justified.
Editor's note: This interview has been edited for conciseness and clarity.