Why AI for social good is a thing
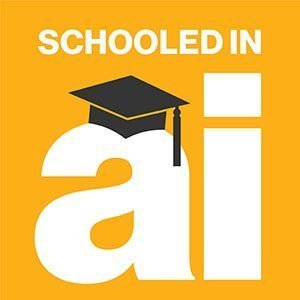
Tech companies love to promote their use of AI for social good. CMU's Fei Fang talks about why the ethics of AI is such a tricky topic in the final episode of 'Schooled in AI.'
CIOs don't have to look too hard to see examples of how technology vendors are taking on social causes.
Use cases that feature how AI technology is helping solve environmental and humanitarian causes have become a staple at data and artificial intelligence conferences. And headlines on, say, Microsoft's new AI for good program or the $25 million Google recently donated to "AI for social good" initiatives are now commonplace. Even Partnership on AI, an organization made up of researchers, academics and representatives from technology companies, includes AI for social good as one of its six pillars.
In the eighth and final episode of Schooled in AI, Fei Fang, assistant professor at the Institute for Software Research at Carnegie Mellon University, talks about the topic of AI for social good.
In this episode, you'll hear Fang talk about:
- The role of multi agent systems in AI
- Why teaching an AI for social good course is important
- How CIOs can tackle the tough topic of ethics in AI
To learn more about Fang's research on multi agent systems and AI for social good, listen to the podcast by clicking on the player above or read the full transcript below.
Hey I'm Nicole Laskowski, and this is Schooled in AI. Fei Fang is an expert in something called multi agent systems.
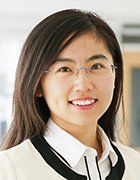
Fei Fang: A multi agent system is just a system with a lot of agents in the environment. So, what is an agent? An agent could be a human. It could be a software agent.
She went on to describe this to me in simple terms. Let's say you're selling something on eBay and several people place bids. All of those bidders? They're agents -- probably human, in this case. But take bidding for advertising space on, say, Google?
Fang: In those cases, it's usually not an actual human who is bidding, but instead it's a software agent bidding on behalf of a company.
Fang described agents, be they human or machine, as intelligent, as having their own objectives and preferences.
Fang: And when they interact with each other, there are all kinds of interesting things happening, and that's what multi agent systems are studying.
Fang is also an expert on something called AI for social good. In fact, she taught a course on the topic just last spring.
Fang: I am an assistant professor here in the Institute for Software Research in the School of Computer Science of Carnegie Mellon.
These two things -- multi agent systems and AI for social good -- are not mutually exclusive for Fang. In many of her examples of multi agent systems, the agents are potential criminals as well as those trying to prevent criminal activity from happening. And she uses machine learning and game theory to increase law enforcement's advantage.
Fang: Based on the research or on the study of multi agent systems, we can figure out what we can expect for systems and what strategies to use for law enforcement agencies, for example, to optimize their limited budgets and resources to combat illegal activities.
The objective is to uncover patterns that can help predict what's going to happen -- and sometimes where it's going to happen.In an antipoaching example she gave, that meant …
Fang: … trying to find out what kind of patrol routes the rangers should take so that we could reduce the overall level of poaching.
Her research depends on data. For antipoaching, that meant tapping into data from nongovernment agencies to build what she called poacher behavior models. But in some cases, useful data doesn't exist.
Fang: And in those cases, what we consider is what would be the worst case for law enforcement if they patrol or if they allocate their resources in this way.
When I initially reached out to Fang, it was to talk to her about AI for social good. I read that she taught a course on this at CMU and I'd begun to notice this pattern among vendors.They started showcasing examples of how their AI tech is helping farmers or how it's assisting in disaster relief efforts. And I wondered what CIOs were supposed to make of this.
Now, I'm sure there are lots of reasons why tech companies are presenting these socially good use cases. But what Fang and I talked about was how AI has a kind of dubious reputation right out of the gate. There's worry that AI will take jobs, will undermine personal privacy and wreak havoc on securing data and systems. Fang, though, brings a different perspective to the conversation.
Fang: There is the stigma, a feeling, that AI only leads to dangerous situations. But that's not the case.
She hopes that courses like AI for social good and the kind of work she's doing will show another side of the technology.
Fang: We can use AI to help the government agencies or the nongovernment agencies who are aiming to serve the people, and we try to help them improve efficiency in their daily operations or in their decision-making.
She does a part of that by teaching students how to apply AI to complex issues where the optimal solution may not be obvious.
Fang: When we try to use AI for some socially good problems, for example, how to more efficiently allocate social housing, then, inevitably, fairness and privacy and all kinds of ethical aspects come into play.
And she stresses to her students that debates on how to apply a technology so powerful it could change the course of someone's life should not be confined to coders.
Fang: AI researchers may not even have the expertise to judge what is ethical and what is not.
And for that very reason, she's welcomed other CMU professors into her classroom.
Fang: So, to give a concrete example, Professor Tuomas Sandholm at CMU has been working on a kidney exchange. And here, from the AI perspective, the research team would work on developing algorithms that can compute the best algorithm that matches donors to patients.
The problem is not black or white. Fang said it's important for her students to understand the objective Sandholm is after. So, is the objective to maximize the number of people who get kidney transplants or is it to maximize overall compatibility or the number of lives saved?
Fang: There needs to be a discussion with all kinds of experts to discuss what should be set as a goal.
Fang is hoping she's laying the groundwork for students to take that kind of thinking with them after they graduate.
Fang: Raising awareness among researchers and students is important because when they design their own AI algorithms, they will keep in mind that these are the things that they need to pay attention to.
It's a point that CIOs face, too. How do they know an AI algorithm is making fair and unbiased recommendations? Fang described this as a significant area of AI research, but she also said CIOs need to think through the inherent risks they open themselves up to when applying AI to a business problem.
Fang: But the problem is it might be hard for them to get an answer from the ones who developed the software. So, there might be, let's say, a third party or something that does this kind of investigation. This is my personal opinion.
She also suggested CIOs think about failure.
Fang: So, all these algorithms probably made some assumptions and it makes sense to ask in what circumstances these assumptions will fail and what would happen if these assumptions did fail.