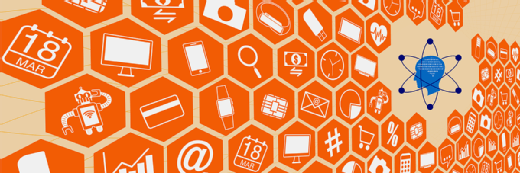
5 tips on how to pick the right AI use cases
Businesses may not be ready to jump all in to AI just yet, so starting with individual projects can be a good place to start. What should CIOs look for in early-stage AI projects?
Google was one of the pioneers in using AI, and the investments paid off with the company doubling its net income year over year after only a short period of time. Today, many companies seek to join the AI revolution. Genesys predicted that 60% of U.S. companies will be using AI by 2022, and the reason for this is simple: Not only have they seen the results, but the fear of losing the market to AI-driven competitors is a powerful source.
However, AI use cases must not be approached relentlessly. As Arijit Sengupta, CEO and founder of automated machine learning tool Aible and one of the original architects of Salesforce Einstein, explained: "It can be very hard to provide stats to prove the effectiveness of AI in business because the vast majority of AI projects are failing. In 2018, the industry research firm Gartner estimated that 85% of AI projects won't deliver for CIOs. AI has tremendous potential, but the way most AI is trained today is failing for business."
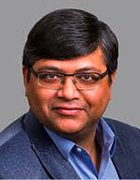
Below, we discuss the right ways CIOs should approach their AI use cases and strategy to be successful. We've interviewed dozens of AI experts and are sharing their hard-earned hands-on experiences, which you usually don't find online.
It's all about data
It's commonly known that AI feeds on data, yet the importance of data is often underestimated.
"The scope and scale of the data problem in AI is far larger than most people realize," explained Jen Snell, vice president of Verint, a chatbot development company. "So many organizations run into problems with their projects due to data -- from data quality to managing and wrangling data for meaningful insights to labeling and model building," she said. "In the beginning, it seems easy, but as you look to grow at scale, change models, manage and ensure control over the system, it gets tricky."
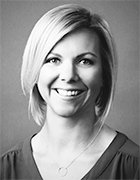
Snell shared a painful statistic: While 59% of executives believe AI could improve their use of big data, 85% of big data or AI projects fail. "We had a sense for that over 15 years ago, and it took a few years of working with customers and real-world data to know how widespread and systemic the problem is."
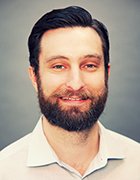
Thus, having reliable and clean data is imperative for AI transformation -- even more than the AI algorithm. "I learned that tweaking the algorithms and the math of a given model yields very little improvement. The biggest boost to accuracy you can get is from good, clean training data," said Aaron Edell, director of applied AI at Veritone, an AI technology and solutions provider. "Having a data acquisition strategy early is the key to success in machine learning -- something I wish I had known since the beginning."
How you obtain the data is also critical for AI use cases. While purchasing data from external sources can get you off the ground, it's not enough to keep your business running because AI does not understand what it does and it will only be as good as the data it is given. Edell discovered this the hard way when working on generically trained models. "A celebrity recognition model trained with 1 million 'celebrities' will perform poorly in real-life use cases because it wasn't trained on the data it is meant to be running on," he said. "If I'm trying to report how often and for how long Jennifer Aniston appears in every episode of Friends, applying a model that was trained on red carpet photos of her at the Oscars will not perform as well as a model trained on screen grabs from actual episodes of Friends."
Every company may need its own fine-tuned AI that is specific to its business. This will lead to more efficient algorithms in the long run that will bring higher revenues, reduced costs and happier clients.
Pick the right starting point
A successful AI transformation is an all-encompassing change. It's not just about the technological changes, but also the cultural changes and the resistance you face from the staff. Thus, CIOs must plan for success from the first brick.
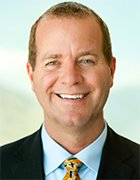
Methodically, when choosing AI use cases, it is best to "look first at the lowest-hanging fruit," as Lyle Ball, CEO of workspace automation company Avii, described it. "Find the highly repetitive tasks where people are involved, and have a system accomplish those functions instead."
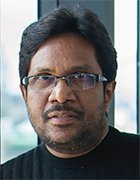
Processwise, though, it is best to start with anything consumer-facing first. "Customers expect a contextual and personalized service at a channel of their choice," explained Shridhar Marri, CEO of Senseforth, an AI-powered chatbot solutions provider. "The entire spectrum of acquiring, engaging and supporting customers is a key dimension that is being transformed by AI technologies."
Profit and satisfaction gains from improvements in your customer services also help you demonstrate the value of the AI transformation, particularly when facing resistance from colleagues and staff. "That helps to open the door for other uses," Ball summarized.
After the customer experience, Marri's suggestion is to go after increasing operational efficiency and reducing costs.
One must be careful not to confuse the AI transformation with digital transformation. Sometimes, even non-AI-based automation tools are enough for the job. Before rushing into AI, proper research must be made to see if an AI project is the best way forward. Jonathan Duarte, who built AI-based chatbots for the likes of Wells Fargo, shared his experience. "In one project where I came in to lead the development, the project plan was already in place, but there was little customer research done prior to the initiation of the project," he said. "In this case, by the time the chatbot was designed, the users had already figured out a workaround that, while not infinitely scalable, was equally as powerful as the AI tool."
Prepare for the cultural change
It's not enough to solely focus on the technological aspects of an AI transformation. Ironically, while AI seems to be about replacing humans, we've come to learn more about the value of the human workforce in recent years.
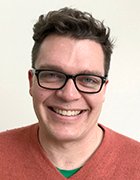
Chris Nicholson, CEO and co-founder of Skymind, which provides an AI infrastructure for enabling machine learning at scale, highlighted the importance of having the buy-in and cooperation of your colleagues. "Your team will probably be using the AI solution you plan to introduce, and if they don't accept it, then it won't be any good," he said.
Furthermore, the team will probably be involved in producing or vetting the data that you will need for the AI solution, and if they don't support your move to automation, you will not get good data. "It's hard to get started with AI, so there's no reason to invent additional difficulties," Nicholson concluded.
It's a journey
One mistake CIOs make when choosing AI use cases is forgetting the unique features of AI. AI is great because it learns, but it only knows what you have taught it. "Setting up an AI [use case] is a journey, not a destination," Marri explained. "You must constantly retrain your AI to keep it up to date and usable. A chatbot, for instance, needs to learn of your new product offerings or understand new and unexpected consumer requests to prove itself useful."
This strategy is used by many who combine AI with workforce: The humans take care of the edge cases where the AI has trouble, not only improving the UX, but also creating new data for the AI to learn from. At the same time, the AI helps to lift a large portion of the repetitive and manual work.
Know the limits
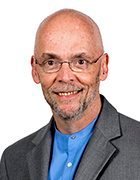
While we discussed how to get started with AI use cases and how to get it right, there are also pitfalls to be aware of to ensure your project succeeds and stays successful. Tom Austin, CEO and founder of The Analyst Syndicate, which is a community of senior independent analysts, pointed to a fatal example. "Don't automate away human judgement the way the designers of the MCAS [Maneuvering Characteristics Augmentation System] system on the Boeing 737 Max 8 did," he said. "AI isn't intelligent -- it's a robot that can do things that people can't, but it can't think."
While such examples may seem extreme, there is an important lesson to learn: "Don't assume you can get away with blaming the technology for making the mistake. Humans are and will remain at the end of the chain of responsibility," Austin said.