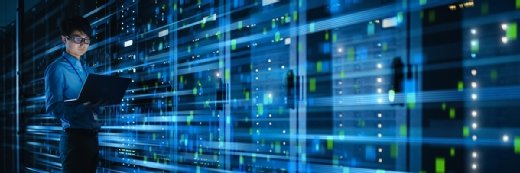
Gorodenkoff - stock.adobe.com
AMD Instinct MI300 AI accelerator takes aim at Nvidia GPUs
Data center-grade GPUs and accelerators for enterprise customers and cloud vendors are the new battleground for AI hardware. AMD and Google advance the race with new chips.
Both AMD and Google released AI accelerators today: AMD Instinct MI300 and Google TPU v5e. Both are data center-grade processors that speed AI tasks, such as training large language models.
AMD is playing catch-up to Nvidia, which has parleyed its gaming tech expertise into an AI processing superpower. AI typically runs on chips adjacent to CPUs; AMD's accelerator is a GPU, while Google's is a proprietary tensor processing unit (TPU) that powers AI in the Google Cloud.
What do the 153 billion transistors in AMD's MI300 accelerator -- and its claimed 17TB/second bandwidth -- get enterprise IT buyers? The Instinct MI300 chips run AI operations much faster, AMD CEO Lisa Su said at a launch event.
AMD customers and partners there, including Dell, HPE, Microsoft, Meta, Oracle, Databricks and others, said they had the chips either running in their products and services, are testing them, or plan to use them soon. Not only are the chips faster than their predecessors, but they can be combined to further improve performance.
"Generative AI is the most demanding data center workload ever," Su said. "It requires tens of thousands of accelerators to train and refine models with billions of parameters. And that same infrastructure is also needed to answer the millions of queries from everyone around the world.

"It's very simple: The more compute you have, the more capable the model, the faster the answers are generated. And the GPU is at the center of this generative AI world," she said.
The hardware upon which AI accelerators run has become a key feature of AI accelerators, said Daniel Newman, Futurum Research founder. It's not just speeds and feeds anymore but open source platforms that let developers build software and connect their large language models to the hardware.
"Today is all about AMD entering with valid, competitive capabilities and products using open source in the era of an incredibly strong or even dominant Nvidia in the AI training [chip] and overall AI chip," said Daniel Newman, Futurum Research founder. "It isn't just about performance. It is also about availability, viability, capability, and the world understanding that open-source collaborative ecosystems for AI are important."
Enterprise AI buyers, take note
Many companies still field their own GPUs in their data centers or colocations -- even in the cloud-first era -- Gartner analyst Chirag Dekate said. Data privacy regulations or the need for intellectual property protection force companies to take a hybrid approach that mixes their own data centers and public clouds such as Google, AWS and Microsoft.
In some cases, an enterprise might run its proprietary LLM in its own data center to keep it off a public cloud.
The AMD GPU accelerators will be adopted not only by large public clouds but also by individual enterprise customers, Dekate predicted. The combination of hardware, software and partnerships will help those customers set up their AI operations faster.
"What AMD is announcing today is not just a GPU that can be deployed in the data center," Dekate said. "They're also announcing cloud partnerships. They're announcing platforms and software stacks. [Together they will] enable enterprises to hit the ground running with an AMD-native strategy."
Google delivers new AI accelerators
Amid its Gemini general AI model release and unveiling of plans to be the first manufacturer to put generative AI on smartphones, Google also released the TPU v5e, its latest AI accelerator. TPUs power Google's own AI in apps such as Maps, YouTube and Gmail, and it hopes Google Cloud Platform customers will follow suit.
In the future, it's likely that enterprise cloud services buyers will have different AI services powered by different manufacturers' chips, Dekate said. Some enterprise applications and operations will work best -- or cheapest -- on one chipmaker's array compared to the others. It will depend on the scale and bandwidth required for a job, such as training a large enterprise language model.
Competition will be the key to keeping AI chips viable and to keep advancements moving in the AI hardware race as each manufacturer tries to outdo the others, Newman said.
"Ultimately we need a highly competitive marketplace for AI infrastructure, chipsets, software, and more," Newman said. "[Generative AI represents] the biggest transformation our world has seen technologically, and a healthy, vibrant, competitive ecosystem is critical."
Don Fluckinger covers digital experience management, end-user computing, CPUs and assorted other topics for TechTarget Editorial. Got a tip? Email him here.