Evaluate cloud data warehouses based on data, outcomes
Organizations must focus on data and desired outcomes -- and question their assumptions -- when evaluating cloud data warehouse needs, according to industry expert Dr. Barry Devlin.
Organizations are at a crossroads as they evaluate their cloud or on-premises data warehouse needs. To make the right decisions, they must maintain focus on their desired data goals and outcomes without relying on outdated assumptions.
Industry expert Dr. Barry Devlin sat down to discuss the shift of on-premises data warehouses to the cloud, the factors to consider when evaluating cloud and the importance of asking the question, "Why do you need this?" He has more than 30 years of IT experience, defined the first data warehouse architecture in 1985, and is founder and principal of 9sight Consulting.
Devlin covers the keys principles of cloud data warehousing, the meaning of data and how to foster an environment of insight-driven decision-making in his book,Cloud Data Warehousing Volume I: Architecting Data Warehouse, Lakehouse, Mesh, and Fabric.
He will dive deeper into the architectures of data warehouses, lakes, fabrics and mesh in Volume II, which is expected to be published in early 2024.
Editor's note: The following interview was edited for length and clarity.
What has been the biggest influence on the shift from traditional data storage methods to cloud data warehouses?
Dr. Barry Devlin: I find this a really difficult question to answer other than to note that here's a huge marketing push driven by vendors who have things to sell … and we seem to be besotted with the biggest, best and latest technology, rather than really thinking about what's underneath the covers. This is the reason why I started my new book with what I mean by data warehousing: as a means of getting quality data and obtaining the best possible value from it for making decisions and taking action. At the end of the day, it doesn't matter if it's on the cloud or on premises or wherever it is. More important is the way you think about information and data. Underpinning all this is, of course, technology and whether the data is all in one place or is it distributed over multiple places. That, of course, does influence architectural and design decisions.
Are there advantages to keeping on-premises data warehouses, or is cloud always the best option?
Devlin: It's about outcomes. It's about what I want to achieve for my organization. What information do I need to help make those decisions. It shouldn't really matter where that info lies from a business point of view. Each has its strengths and weaknesses. There's pros and cons to having information on the cloud vs. on premises. In particular, look to sourcing -- where does your key data or your biggest data originate -- and how do your users need to access it?
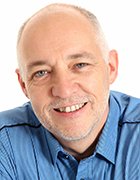
When you discuss the seven deadly sins of data warehousing in Chapter 3, are those in a state of flux and must be constantly monitored for the next potential "sin?"
Devlin: They do carry forward, and they also morph a little bit as we go. The point for taking this approach was to really emphasize to people that we make a lot of assumptions when we decide something and design a system. For example, sin number two here is that operational and informational systems are separate. That's an assumption in the data warehousing world we have carried around for many years. The reasons we came to that conclusion are, in large part, no longer true. Therefore, these days, it's a deadly sin, but 30 years ago, it was the right thing to do. These assumptions come out of what technology can do and what businesses want at a particular time.
Today's businesspeople are keen on having real-time data. Every time I have a discussion with a business about information, this is one of my first questions: Why do you need it so fast? Sometimes, they think well, actually, I don't need it so fast. But, when they do, the technology assumption that operational and informational systems should be or even can be separate breaks down. You haven't got time overnight to do the transfers and reconciliations from all the different systems.
So, these deadly sins really are assumptions you're making based on your history. Each of them is a consideration you want to go back to in the cloud data warehousing era and say, 'Is this true? What does it look like in my org, in my era, with my business folks and with the tech skills I have?'
Generative AI is the hot topic currently. What do you see as the biggest benefits it can bring to cloud data warehouses?
Devlin: I think it's a topic I need to take on two levels. At the highest level, I am very interested in information and the management of information and how we ensure information has got high quality. Generative AI is based upon a huge corpus of information, i.e., the internet. I have strong doubts about the quality of info on the internet. Generative AI is based upon a very dirty set of data -- data that is highly biased, that has been collected by organizations like Facebook and Google to drive their advertising business and monetize all information. … Generative AI is going to change an awful lot of things, but I fear, in many cases, it's not going to be for the better.
In data warehousing, I think [generative AI] will help in the design of lakehouse, mesh and fabric because there's probably enough good metadata about data modeling and data pipelines out there already to build on. In that sense, [it] improves productivity, although the social implications of lots of people losing their jobs is another concern.
Any final comments you'd like to make?
Devlin: We've had a very philosophical discussion about many different aspects of the world and IT. But there is a very practical purpose behind this book and Volume II. I want to step back from these three patterns -- data lakehouse, fabric and mesh -- and offer a useful and usable basis on which to understand and compare them -- an independent view of what these things do, as opposed to selling any one of them over another. I want to help readers see what is underneath the covers of each of them, beyond the marketing hype. In Volume II, out hopefully early in the new year, I'll be digging even deeper into the architectural design patterns and technologies underpinning each.