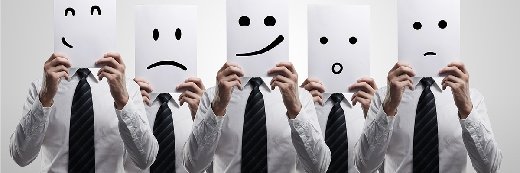
peshkova - Fotolia
Virtualization automation challenges underscore AI usefulness
Automation helps reduce the complexity of virtual systems, but admins might find that adoption challenges and temporary resolutions underscore its usefulness.
Over the course of the next decade, the field of IT will go through some significant changes and the role of IT administrators will evolve and adopt new responsibilities. Significant changes in the coming decade include utter reliance on virtualization automation and hybrid cloud platforms, which have already become standard operating procedure for service providers and large enterprises in modern IT.
It's not that admins should always complete IT tasks without automation, or that data centers should migrate entirely to the public cloud or stay completely on premises. Virtualization automation and hybrid cloud have already begun to affect the everyday lives of admins, and those admins must begin to focus on how to adopt these technologies to successfully modernize their systems. But admins must also understand that automation is not the end-all, be-all of system adoption and management.
The robots are here
One of the main benefits of virtualization automation is eliminating human intervention and hand-rolled scripts for each system. Instead, admins can centrally create automation policies and push them out to groups of devices. Even configuration management vendors, such as Puppet and Ansible, use automation techniques to complete many of their IT operations.
As a result, admins have collectively migrated much of the administration and automation operations of their networks to AI workers, which usually take the form of management applications. Some AI workers are cloud-based, such as Microsoft Intune, and others aren't, such as VMware's vSphere platform.
Even small business networks rely on some form of automation, though they generally use carefully curated collections of scripts assembled by admins. Most SMBs have yet to advance beyond Group Policy Objects (GPOs) enforced by Microsoft's Active Directory, and they often employ VMware's Distributed Resource Scheduler (DRS) to automate tasks.
In larger organizations, using GPOs and DRS usually doesn't cut it. Many opt to replace DRS with Turbonomic -- previously known as VMTurbo -- or one of the many competitors that provide improved resource scheduling as well as resource planning and overprovisioning analytics. But some endpoint management applications -- such as Intune -- augment GPOs, and endpoint protection applications provide a way to implement automation policies.
Automation policies lessen the load, but not the work
Template and profile-based policy management is the logical evolution of previous administrative approaches. It is a form of automation that reduces the amount of available jobs by enabling the existing number of admins to address the growing number of systems within their businesses.
Admins often use whatever they have available to accomplish tasks in the timeframe allotted. However, the approaches chosen based on tool availability are incredibly labor-intensive. Profile and template-based policy implementations free admins from the tedium of individually managing systems, although introduce tasks related to defining policies because of the increase in systems within organizations.
New types of automation can even take over policy definition from admins, freeing those admins to oversee other tasks.
Beyond the hype of AI and machine learning
AI and machine learning can lessen the burden of defining policy for many admins. Endpoint protection products increasingly employ AI and machine learning -- not only to aid in detection of security risks, but to also automatically determine what a policy should be.
Cloud-based AI workers can correlate telemetry data about policy implementation until the system has a new idea of what secure defaults should be. In addition, automated incident response helps not only in flagging potential security breaches, but in connecting security systems to infrastructure in order to automatically quarantine at-risk systems.
But adopting and implementing AI and machine learning technologies can cause problems for some admins. Just as policy enforcement automation came piecemeal, so, too, is the machine learning revolution -- now with additional cloud management necessities.
For example, Wi-Fi access points and associated management software are more difficult to implement than some admins might think. Counterintuitively, these systems require as little transmit power as possible. Otherwise, none of these devices can communicate with one another because every device in the surrounding area transmits its own signals.
Admins can face troubles with this, even with all the right tools. Wi-Fi environments are dynamic and can easily collapse if admins don't configure their access points correctly. Wi-Fi products constantly scan the radio environment for Wi-Fi and Bluetooth, and then optimize the access points to serve associated clients.
Individually, AI and machine learning aren't life-altering technologies. Each of them has its benefits and use cases, especially for larger organizations. But AI and machine learning present merely incremental responses to common pain points.
Bulk data computational analysis tools, whether machine learning or AI, are not new. However, machine learning and AI have pivoted from business analytics to enhancing the operational efficiency of IT. The hybrid cloud-based AI worker infiltration of data centers comes in response to organizations managing more systems with the same number of people.
In the coming decade, the tools admins use will continue to change. Eventually, more capable AI workers will manage lesser AI workers, and human admins will keep moving up the stack. Organizations will add increased layers of abstraction, each with its new levels of management, between admins and the workload. Some might consider this inefficient because this system devotes additional cycles, storage and network bandwidth to keep workloads running.
However, certain applications, such as Netflix, require these layers of abstraction and automation. At some point, human admins simply can't scale a system to the same degree as AI and machine learning.