7 SOC automation use cases to augment security operations
Implementing SOC automation can have far-reaching benefits for an organization's infosec program and security culture. Learn how by exploring these seven use cases of AI in SOCs.
Even before COVID-19 radically impacted business operations, the proliferation of systems, data, cloud technologies, apps, devices and distributed endpoints was already expanding cybersecurity risks. Not only are the challenges facing a security operations center, or SOC -- the centralized "heart" of an organization's cybersecurity detection, analysis and prevention -- constantly changing, tool sets are rapidly evolving as well.
Among cybersecurity technologies, AI has significant potential to reshape how businesses approach, resource for and protect against cybersecurity threats. Specifically, software offerings are using machine learning, deep learning, computer vision and a host of related techniques to review massive amounts of data quickly to detect potential malicious behavior at scale.
Research has identified the following seven unique use cases for SOC automation.
1. Incident analysis
Various AI techniques are used to mine data on security incidents, parse them based on parameters, cluster them for commonalities and assign risk scores. The core role of SOC analysts is to monitor for threats, but historically, this has required tedious and repetitive triage for them. This could result in misidentified threats, inefficient use of highly skilled analysts, staff burnout and turnover. AI helps scale analysis efficiently by casting a wide net -- a net that continues to grow wider and wider.
2. Landscape analysis
AI is harnessed to defend widening topologies. Companies are digitizing more and more of their operations. This includes updating old and developing new internal -- often hybrid -- platforms and networks. As more employees use cloud apps and mobile devices for work -- not to mention increase IoT configurations -- the enterprise security perimeter spans far beyond the organization's traditional "four walls." Extensive network and endpoint security resources are required to manage all communications, transactions, connections, applications and policies. These resources are often disconnected, thus limiting visibility and details of the risk profile. AI can support, reach and scale across these heterogeneous topologies, while correlating threats and assessing how one threat may impact another resource.
3. Incident detection
This SOC automation use case helps differentiate and prioritize different classes of threats and distributes notifications or prevention activities accordingly. This could take many forms, from automating ticket creation and adding pertinent remediation information to detecting the presence of malware before malicious files are opened. AI-powered incident detection is obviously crucial for preventing attacks as it reduces dwell time and accelerates time to repair, but it is also enables preemptive and proactive measures.
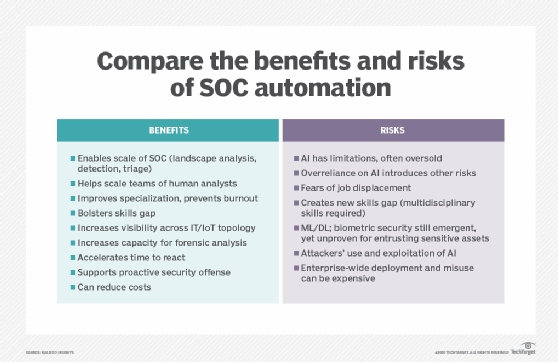
4. Incident response
AI is used to preempt malicious attacks by automating containment actions; orchestration of software, devices or networks; or the deployment of other specific safeguards. Incorporating AI's predictive capabilities helps complete the shift from reactive cybersecurity mitigation to a proactive cybersecurity strategy in an enterprise's fight against hackers. The use of AI-powered incident response applications in organizations today remains incremental, but a proactive approach to the never-ending cybersecurity storm is critical for enterprise security.
5. Emergent threat mitigation
SOC automation is used to learn about novel threats by recognizing patterns or clusters and then providing feedback. Some companies are training machine learning algorithms to recognize attacks perpetrated by other machine learning algorithms, such as smart malware or artificial hackers and bots that personalize attacks tailored to specific victims.
These emerging, AI-based threat mitigation techniques will prove useful as attack tactics, such as malware, botnets and ransomware, continue to mutate along with the pernicious ways AI is used to target and manipulate user and business vulnerabilities.
6. Gamification of security training
AI can also be used to simulate diverse types of attacks and make the education process more fun, engaging and competitive for security analysts. Microsoft's Into the Breach exercise is one example in which the company divided SOC analysts into different teams. The teams were challenged to defend against AI-generated threats, which were developed based on data and techniques derived from real-world attacks.
While nascent, this SOC automation application has the potential to scale a culture of security far beyond the SOC. Not only will it make security training more accessible, personalized and fun, but it can also arm the front line of cybersecurity with essential awareness and security workflow best practices.
7. Human SOC analyst augmentation
This is the AI market's parlance for how AI is used to optimize humans' threat intelligence and mitigating actions. Although automated techniques are better at managing the volume of potential threat vectors, AI is not equipped for complex problem-solving. Human analysts remain the essential arbiters to develop controls, explain threat techniques and uncover attackers' motives. Tests show that the highest performance and accuracy of machine learning are often achieved due to a combination of human and AI intelligence. Thus, AI is unlikely to completely displace SOC analysts but rather supplement the team's efforts and talents.
Across all the above SOC automation use cases, the greatest value AI brings to SOCs comes from combining the virtues of both human and machine learning. AI's efficiency at scale across massive data sets and security topologies enables analysts to focus only on mission-critical decisions and analysis.
Human experience, grasp of nuance, and cultural or linguistic sensitivities are increasingly important as attackers' tools and techniques evolve. Because AI learns through interactions, supervision and validation, human analysts offer a crucial nexus for training and optimizing the AI feedback loop. With proper governance, augmented security tools will help scale and optimize an organization's SOCs by enabling analysts to stay focused on the why when it comes to cybersecurity incidents.