AI, machine learning in cybersecurity focused on behavior
Artificial intelligence, and machine learning in particular, is being fruitfully employed in IT security tools. Learn where this advanced technology works best now.
AI and machine learning are at the top of the buzzword list security vendors are using today to differentiate their offerings. Those terms also represent truly viable technologies; artificial intelligence and machine learning in cybersecurity products are adding real value for the security teams looking for ways to identify attacks, malware and other threats.
The massive increases in the numbers of new attacks, instances of new malware and variants of old malware is reason enough to employ machine learning in cybersecurity. These tools possess the ability to process, detect, identify and remediate all kinds of threats in milliseconds. The legacy "send IT an alert and they can follow up" mentality of yesteryear just won't work.
But the value of AI and machine learning in cybersecurity is found in its ability to actually spot the "bad guy" -- in whatever form that may take -- as proactively as possible. With so many possible threat vectors, what should products be spending their AI and machine learning cycles on?
The overarching answer is simple: behavior.
From antiviral to behavioral security
The security industry started with the simplest behavior it could find: the execution of new malicious programs (read: viruses) onto a given endpoint. Look for that specific behavior (read: signature-based detection) and bam! -- the antivirus industry was established.
But, today, cybercriminals are diligently looking for ways to outsmart cybersecurity products, changing behavior -- even using evasive behaviors -- to avoid detection. So, the tools you employ need to have AI or machine learning watching for a number of specific behaviors (as is appropriate for a given product). Behaviors where the watchful eyes of AI and machine learning add value to security include the following:
- Endpoint behavior: To be effective, malware needs to run on an endpoint. That means files need to be written (with an exception in the case of direct memory injection), processes need to be launched and resources need to be accessed. And none of these look like the typical opening of Word or your web browser, which makes them stand out. In cases where fileless attacks occur and legitimate processes are compromised, the abnormal actions of those processes (e.g., Notepad launching a child process) will be obvious.
- Network behavior: Traffic on the wire is rather predictable. Specific endpoints generally interact with the same sites or systems, over the same ports, using the same instances of encryption (as is appropriate), sending the same amount of data. Attacks involve the use of command-and-control servers, abnormal uses of ports, unusual amounts of data and increasing uses of encryption.
- User behavior: Users are at work to do their jobs, which normally fall into a predictable and finite number of semi-repeating tasks. They log in mostly on the same days, at around the same time, use the same applications, access and interact with the same types and amounts of data, and communicate with the same people about the same things. Attacks involving the compromise of an endpoint and user credentials yield unusual logon times, multiple connections to systems, abnormal use of applications, mass copying of data to the cloud and more. Should an attacker "live off the land" and use applications native to the compromised endpoint, this, too, will be seen as unusual in most cases, helping to identify threatening behavior.
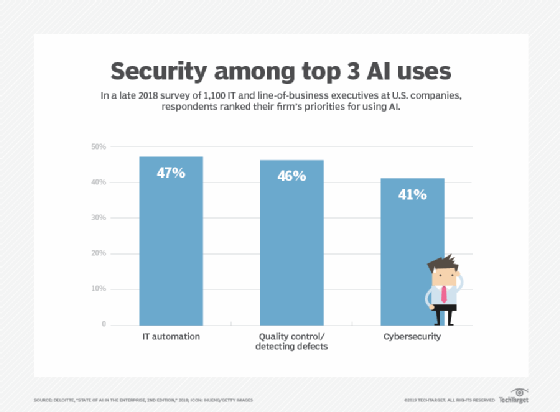
The point here is when you're looking at products that tout the use of AI and machine learning in cybersecurity, the question should be "What data are you looking at?" The answer needs to revolve around attack behaviors that indicate attempted or successful intrusion, discovery, compromise, movement, manipulation or exfiltration.
AI and machine learning tools are no longer just hype; they're very real technologies that spot abnormal behavior in far less time than any human. It will be interesting to see what the future holds for AI and machine learning in cybersecurity. As both sides of this battle evolve their efforts, will AI and machine learning spell the end for cyberattacks -- or at least some forms of them? Only time will tell. But, for now, behavior-focused AI and machine learning tools need to be a part of your security strategy if you're going to put up any kind of a fight.