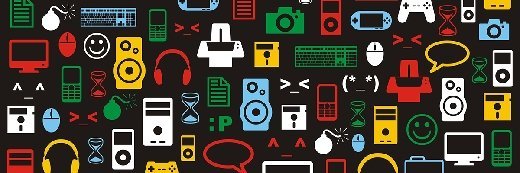
Kit Wai Chan - Fotolia
Diversified data sets for analytics deliver top results
Analytics teams should focus on data diversity to ensure that their projects deliver the most meaningful insights -- but they must be wary of some stumbling blocks.
After Toyota launched its Prius V in November 2011, drivers soon noticed a funny sound when braking. Prius owners started calling customer support centers in April 2012, and the company subsequently worked out a fix for the brake problem based on their feedback.
But the fix could have come sooner. A review of social media posts showed that drivers began posting videos to document the sounds their cars were making in January 2012, three months before the problem became apparent through the customer service calls.
Jayadev Gopinath, who heads analytics and data management operations at Toyota Motor North America Inc., said this story shows the power of diversified data sets for analytics. The engineering team at Toyota told him that it could have worked out a solution to the problem earlier if it had access to information about the social media posts. But, at the time, the company wasn't systematically analyzing social media data.
Finding value in multiple sets of data
Lesson learned, according to Gopinath. "There's data everywhere," he said in a presentation at the Gartner Data & Analytics Summit 2018 in Grapevine, Texas. "It's not that we don't have data, but it's typically organized in siloes. The real value is if you can link it up."
Today, Toyota is analyzing a range of data sources in a more systematic way. The company uses Power BI and Tableau to access a data lake that ingests data from a range of traditional sources, including customer data, vehicle data and manufacturing data. The data lake also has data from outside sources, including JD Power, Experian and social media sites.
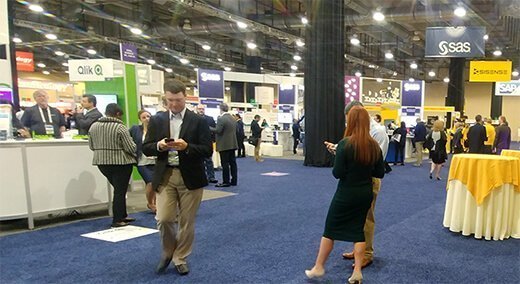
This has enabled Toyota to do more tailored marketing campaigns and monitor the operating conditions in its manufacturing plants; in the future, it also plans to monitor vehicle health remotely through connected car devices. "The key to all this is data," said Gopinath, whose full title is general manager of advanced technology, platforms, innovation, data and analytics.
A diversified data set can enhance an analytics project, but bringing together multiple sources isn't without risk. There are some considerations to keep in mind when trying to build the broadest data set possible.
Establish trust in data via governance
The biggest issue when diversifying data sets for analytics is ensuring trust in that data, said Gartner analyst Kurt Schlegel. He said that people today have a tendency to act as they did in an earlier era of business intelligence during which analysts pulled data from a system of record -- typically, the data warehouse -- that contained verified data.
But that can never be the case when line-of-business users are given self-service data preparation and exploration tools that enable them to combine and analyze data from essentially any source, including data lakes that aggregate data from disparate sources.
To solve this problem, Schlegel said data governance policies should operate like Wikipedia: Enable users to build their own data sets and do their own analyses, but also use metadata to tag where individual pieces of information came from or where an analytics output might be weak.
"The data lake does not replace the data warehouse," Schlegel said. "But we can make it possible to establish trust."
Educate users in a diversified data world
Another way to address the complexity that comes with an environment of diversified data sets for analytics is educating users on how to take advantage of them. Gartner analyst Rita Sallam said data literacy is a key component of a self-service environment in which users have access to a range of data sources.
Sallam, who also spoke at the Gartner conference, acknowledged that enterprises have a lot to gain from putting data in the hands of front-line workers who can most directly operationalize data-driven insights. But simply giving data and BI tools to such users is not enough, she said. To make self-service BI most impactful, analytics capabilities need to be paired with strong training programs that instruct users on data quality best practices, according to Sallam.
"The self-service mantra, while necessary, is starting to show its limitations, particularly as complexity grows," she said. "Developing literacy in data and analytics programs is a key challenge."