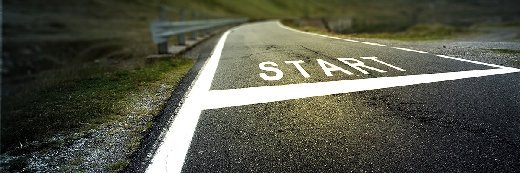
lassedesignen - Fotolia
Ten steps to start using predictive analytics algorithms effectively
A successful predictive analytics program involves more than deploying software and running algorithms to analyze data. This set of steps can help you put a solid analytics foundation in place.
There's a growing trend among businesses to use predictive analytics algorithms to boost their bottom line. Compared to descriptive analytics, which provides a perspective on what has already happened, predictive analytics attempts to discern future events -- for example, identifying people who may be receptive to marketing offers.
But to take full advantage of predictive models, analytics teams need to balance the adoption of advanced analytics tools and techniques with the establishment of new or modified business processes and other organizational changes. Pure and simple: Analytical findings that don't influence decision-making and corporate behavior in a productive way won't add any business value.
What kind of value can predictive analytics algorithms provide? In addition to better targeting marketing campaigns and online advertising, retailers can use predictive modeling to guide inventory management and drive recommendation engines that suggest more purchases to customers. Manufacturers can run predictive maintenance applications to detect impending problems in plant-floor equipment and products in the field. Predictive analytics can also aid in risk assessment and management -- for example, in evaluating insurance risks and spotting financial transactions that may be fraudulent. And it can help healthcare providers identify symptoms that point to particular diseases or medical conditions.
That's just a sample of possible predictive analytics uses. It isn't merely a matter of choosing and running an algorithm, though. An effective predictive analytics program requires engagement and support from business users across the data analysis lifecycle. Consider taking the following 10 steps to help foster that and put other foundational elements in place to set your analytics initiative up for success.
- Find a corporate champion. It's wise to start by getting the backing of a senior leader who's willing to invest both resources and personal goodwill in ensuring that the organization supports the predictive analytics process and the operational improvements it helps drive. For example, a chief marketing officer might be a good candidate to sponsor a customer analytics program because the CMO can provide the necessary funding and direct marketing teams to use the results generated by the predictive analytics algorithms in planning campaigns and promotions.
- Understand the company's key business objectives. The best predictive models are ones that share two important features: accuracy on predictions and relevance to the business. You can improve the probability of creating business value when the data scientists and analysts doing the analysis work are well aware of the organization's business goals and concentrate on building models that can have the maximum impact in meeting those objectives.
- Evaluate how business processes could be affected. Predictive models help identify business opportunities, but even the best-designed ones will be ineffective if the results of analytics applications aren't used to guide the actions of business managers and operational workers. Identify business processes that can be improved based on the findings of predictive analytics algorithms, and get department heads on board with preparing their workers to put those findings into action.
- Define performance metrics. Your predictive models shouldn't just influence changes in business processes -- they should also have a measurable impact on business performance. Devise a plan to create metrics that can be used to quantify the value of employing the models.
- Hire a competent team. Your analytics team should be composed of individuals with a range of skills, potentially including statisticians, data scientists, data analysts, business analysts, data engineers and change management professionals.
- Define your model development methodology. One of the impediments to analytics success is when the time it takes to develop the predictive models exceeds the window of business opportunity. Some organizations adopt Agile development techniques to speed the model-creation process, with iterative refinements to improve the models as they're being deployed. Faster delivery of incremental value is much better than unending delays in delivering the perfect predictive model.
- Make sure the right data is available -- and accessible. Big data is a mixed blessing. Although your organization might have the capacity to collect and store massive amounts of data, the analysts still need to know what data sets are available and how to access the ones that are relevant to them. In addition to creating an inventory of data sets, maintain sufficient technical and business metadata in a data catalog to ensure particular predictive analytics applications have access to the right data.
- Institute a solid data governance program. The corporate data environment is constantly changing, and new data sets are continuously being captured and ingested from both internal and external sources. Implement data governance practices to help guarantee that the quality and consistency of the data in your analytics systems remain at an acceptable level, with associated data usage policies to prevent different interpretations that lead to conflicting analytics results.
- Plan to fail fast when things don't go right. There are going to be many opportunities to build analytical models, but not all will succeed in delivering useful information. Establish the principle of failing fast: If a predictive model isn't producing anything of value to the business, move on to the next opportunity.
- Choose the right tools for the job at hand. There's a healthy market for predictive analytics tools, including open source and commercial options that support various types of analytics -- from data mining to machine learning and deep learning that's based on the use of advanced neural networks. Look for tools that provide a core set of capabilities matching your application needs. A big issue, of course, is support for the particular predictive analytics algorithms you'll be using -- linear regression, logistic regression, time series, decision tree, etc. Other factors to consider include interoperability with a variety of conventional and big data platforms, the ability to handle structured and unstructured data, and integration with data visualization and presentation front-end tools.
These 10 steps aren't an exhaustive list of what's involved in setting up a successful predictive analytics program. But they provide a foundation for gaining sustainable support internally, framing the planned predictive models in the context of business value, engaging business users, managing change and giving analysts access to relevant and trustworthy data. Done properly, they'll help feed repeatable processes for developing, testing and deploying predictive models that can indeed benefit the business.