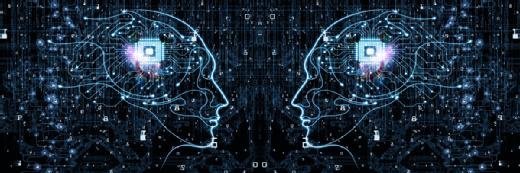
Getty Images/iStockphoto
How to overcome the limitations of AI
AI may not become the super-intelligent tool people envisioned, at least not in the near term. But that doesn't mean enterprises can't use AI in some valuable ways.
The 2010s gave rise to a number of tech bubbles, and the threat of those bubbles bursting in 2020 is resurfacing nightmares for some in the tech community of dot-com-era busts. One such bubble could be AI.
Yet, some of today's most successful tech companies -- Google chief among them -- grew out of the shattered landscape of the post-dot-com tech scene. The same pattern could play out in AI. Even if the current AI bubble does burst, there will most likely continue to be successful companies offering impactful tools.
Some experts claim we are in an "AI autumn," as the technology that once was feared for its potential to wipe out broad swaths of jobs has fallen short of its expected potential in many categories. Yet, underestimating the benefits of AI is a huge mistake, as various machine learning technologies are already providing value to businesses. But, given the limitations of AI, how can we get to a future where the technology has the world-changing impact that was previously expected?
AI's limitations start with intelligence
Google's AlphaGo Zero forced the current world champion of the game Go into early retirement. In Lee Se-dol's own words, AI is "an entity that cannot be defeated." Using reinforcement learning, the AI played millions of games against itself at superhuman speed -- a number humans can't match in a lifetime of gameplay. The hardware costs for AlphaGo Zero also go up to $25 million.
However, the new world champion would fall flat on its face with the tiniest change to the game's rules. It also can't use its knowledge to master any other game. Humans are superior at applying existing knowledge to new tasks with limited data. This is something most AI pioneers agree upon.
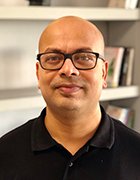
"Current AI algorithms have enormous data requirements to learn the simplest tasks, and that puts a strict restriction on where they can be applied," said Abhimanyu, co-founder and CEO of Agara, which analyzes voice with the aid of AI to augment customer support operators. "While neural networks show superhuman performance, their predictions are sometimes wildly incorrect, so much that a human would never make a similar mistake."
As Jerome Pesenti, Facebook's head of AI, put it: The AI field is about to hit the wall. This begs the question: How can we build smarter AI?
AI can't compete with the brain
Many experts believe improvements in hardware and algorithms are necessary to break that wall. Some even suggest that we need quantum computers.
Though deep learning and neural networks were developed to mimic how our neurons communicate, there is still much we don't know about the brain's inner workings, which outperforms thousands of CPUs and GPUs.
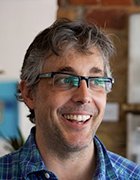
"Even our supercomputers are weaker than the human brain, which can run 1 exaflop calculations per second," Abhimanyu said. "However, since our algorithms have a long way to improve, it's hard to predict how much computation power we'd need."
More processing power doesn't generally equate to more intelligence. We can see this in the brainpower of various animals.
"As a simple proof point, there are animals with both much bigger brains and more neurons than humans have," said Alan Majer, CEO and founder of AI and robotics development company Good Robot. "So, if we wait for some kind of hardware tipping point, we're likely to be disappointed."
Applications of AI must account for its limitations
Recognizing the limitations of AI is the best thing we can do for the developing technology. While we are far off from human-level intelligence, companies are taking innovative approaches to overcome those constraints.
Explainable AI is one important approach.
AI has traditionally operated as a black box where the user feeds the questions and the algorithm throws out the answers. It was born from a need to program complex tasks, and no programmer was able to code all the logical decision variations. Thus, we let the AI learn by itself. However, this is about to change.
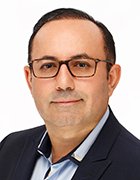
"Explainable, cognitive AI builds trust with people so humans and machines can work together in a collaborative, symbiotic way," said A.J. Abdallat, CEO of machine learning development company Beyond Limits. "Because explainable AI technologies are educated with knowledge, in addition to being trained with data, they understand how they solve the problem and the context that makes the information relevant."
The higher the potential stakes, the more important it is to know why AI arrived at a certain answer. "For example, NASA will not implement any system where you cannot explain how you got the answer and provide an audit trail," Abdallat explained.
Explainable AI gives us insight into the AI's decisions, improving the human-machine collaboration. However, this method does not work in all scenarios.
Consider self-driving cars, one of the benchmarks of our AI intelligence level. In fully autonomous vehicles, human operators are not enabled to aid the machine in split-second decisions. To solve this problem, experts adopt a hybrid approach.
"Waymo uses deep learning to detect pedestrians, but lidar and hardcoded programming add a safety net to prevent collisions," Abhimanyu explained. Developers use individual components that are not smart per se but can achieve smarter results when they are combined. By creating a smart design, developers challenge our understanding of the limitations of AI.
"The Google Duplex demo that amazed people is a really smart design coupled with state-of-the-art technology in speech-to-text and text-to-speech categories, which exploited what people look for in a smart human," Abhimanyu explained.
But these chatbots fail when it comes to natural conversations, which is still a challenging domain for AI. As an example, let's consider one of the major achievements in the past year, GPT-2, which stunned many with its content writing capabilities.
"GPT-2 can generate entire essays, but it is very hard to make it generate exactly what you want reliably and robustly in a live consumer setting," Abhimanyu shared. GPT-2 was trained on a huge library of quality documents from the internet, so it could predict what words should naturally follow a sentence or paragraph. But it had no idea what it was saying, nor could it be guided toward a certain direction. Experts believe being able to reliably and extensively control AI could mark the next step in our advancements.
The current AI algorithms were made possible on the back of big data -- that's why achieving this level of intelligence was not possible even with the best supercomputers decades ago. We are incrementally finding the next building blocks for smarter AI. Until we reach there, the most productive use of AI is on narrow domains where it outperforms humans.