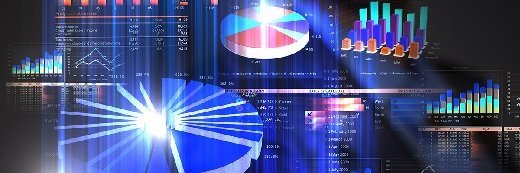
Sergey Nivens - Fotolia
Align business and IT drivers through data quality best practices
Information management consultants talk about the importance of aligning business and IT drivers for a holistic architectural approach to ensuring strong data quality.
Data quality issues extend across -- and often beyond -- an organization. Combining people, process and technology for a holistic architectural approach can help address these issues. But managing data quality has become more complicated due to changes in how data is produced, processed and used.
Managing director Donna Burbank and principal information management consultant Nigel Turner from Global Data Strategy Ltd., an international information management consulting company that specializes in the alignment of business drivers with data-centric technology, talked about data quality best practices in a webinar hosted by Dataversity. They discussed how to achieve good quality data that is what Turner called "demonstrably fit for purpose," meaning it meets defined business needs for accuracy, completeness, reliability, accessibility and timeliness.
The issue of data quality has changed significantly over the past couple of decades, according to Turner. For example, now, it is more real-time, automated and business-driven than in the past, when batch processing and manual data cleansing were prevalent and IT typically drove things. He said data quality also now requires more of an enterprise-wide view than a platform-specific one, due partly to an increased focus on ensuring data is accurate for reporting and analytics uses.
As a result, traditional data profiling and cleansing measures aren't enough anymore, Turner said. Data quality best practices need to be more proactive now, with a focus on validating data as it's created instead of waiting to find and fix errors later on.
"You've got to get it right in the first place," he said.
In addition, organizations need to address data quality issues in different systems in a consistent way, Burbank said. Otherwise, data fixes in one system might be undone by bad data coming from another one.
"You can clean up the pollution in your pond, but if the pollution is coming in from streams feeding that pond, it's still going to get dirty," she said.
The continuing business impact of poor data quality persists, exacerbated by the increasing complexity and volatility of data. According to an estimate by IBM, which Turner cited, the U.S. economy loses $3.1 trillion a year because of poor data quality. Consultant Thomas Redman estimated in the MIT Sloan Management Review that, on average, poor data quality costs companies between 15% to 25% of revenue.
"You can lose a lot of revenue if your data isn't fit for purpose," Turner said.
Poor data quality can have an impact on an organization's brands, reputation and customer loyalty, as well as its legal and regulatory compliance, thus affecting not only revenue, but also costs and profits.
As a case in point, Turner pointed to Amazon, which made the apparent error of pricing a $13,000 camera lens and other high-cost photography products at $94.48 each during its Amazon Prime Day sale in July 2019. The low prices, amounting to discounts of up to 99%, were adjusted after being spotted by shoppers and publicized online. But Amazon honored them for at least some customers who placed orders before the prices were fixed.
Turner emphasized data quality best practices are never absolute in organizations -- and often don't need to be. For instance, if a monthly finance report consists of data that is two weeks out of date, maintaining data accuracy in that window is good enough. But an online purchasing system requires data to be up to date and sometimes real-time for it to work within the context of the business process.
"The world changes, and data models the world," Turner said. "If you don't take proactive steps in the organization to capture those changes and model them in your data, your data inevitably and inherently gets out of date the day after you collect it, in many cases."
Turner also emphasized the importance of recognizing poor data quality as a business problem, not just an IT problem. The key is getting those two teams working together, creating a triangle of people, process and technology.
Turner also said to account for the inevitable human error that affects poor data quality. In many organizations, nobody is formally responsible for data and its improvement, so bad data never gets systematically fixed.
"Sometimes, it's the human interface with the technology that has the poor design," Turner said. "Sometimes, it's the business process itself."
In applying structure to a data governance framework and data quality initiatives, Turner said to get down to the basics and make sure you have a repeatable process for creating high-quality data.
"The more complex [a data environment] gets, the more simple it needs to be," he said.
The traditional approaches to data quality will continue to have great value, though combining them with new approaches for data quality in organizations will yield the greatest benefit.
In the new age of data quality, the focus is on real-time data validation on the front end, rather than cleansing and enhancing at the back end.
The concept of intelligence at the edge is now being accompanied by something Turner called data quality at the edge. For example, they're building basic data quality checking into smart meter devices, which is beneficial when the data is sure to be correct by the time it hits the system. Turner's golden rule of IT: If you prevent a problem, you fix it a lot more easily than if you wait for the problem to occur.
The new approaches include giving business users more control over the creation and management of business rules, ending user self-service data quality functionality, and obtaining a tool set that supports a wider variety of platforms and data types.
A holistic approach encompasses finding the balance between the older and newer approaches. This consists of a mix of human and automated, in the middle of reactive and proactive. Fixing things is the goal, but also looking ahead and proactively understanding and making conscious decisions.
"Sometimes, we know there are issues, but we cannot fix everything," Turner said. "So, it's very valuable to pick your battles."
Both he and Burbank also emphasized the importance of demonstrating the business benefits that data quality projects will provide upfront.
"Unless you have some really hard [ROI] targets and a defined plan, you're going to struggle to succeed," Turner said. "Don't start any data quality initiative until you have a business case for it."
The holistic approach to good data quality combines people, process and technology to enhance data quality and identify organizational and technological issues. Data governance is valuable in coordinating the people, processes and organizational structure, and data architecture provides business and technical alignment to implement data quality business rules into core systems.