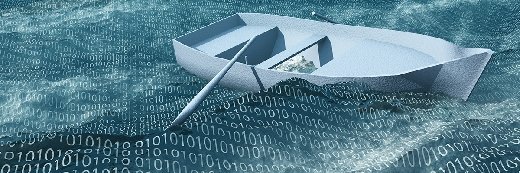
rolffimages - Fotolia
Advice on enterprise data cleansing from an SAP VP
SAP's Kristin McMahon details data cleansing best practices and explains why a good data cleanse needs continual communication, collaboration and oversight.
The enterprise data cleansing process is far from new, but it is an aspect of data management that is essential and can easily be overlooked, according to Kristin McMahon, vice president at the platform and technologies group at SAP.
Data cleanses are key in combatting dirty data -- data that contains errors such as duplicate records and incomplete or outdated information. Dirty data costs the U.S. economy $3 trillion annually, according to a 2016 estimate from IBM. It also costs the average business 15% to 25% of revenue, according to MIT Sloan Management Review.
McMahon, a 12-year SAP veteran, said in this interview, while it can seem tedious and daunting, the data cleansing process is even more necessary today given the huge amount of data that enterprises collect. With more money at risk, it is also increasingly important that data professionals get the enterprise data cleansing process right, she added.
Here, McMahon gives her definition of a data cleanse, explains how it saves time and resources, details the process step by step and advises what to avoid during the process.
What is a data cleanse?
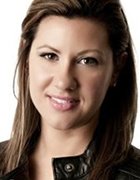
Kristin McMahon: Having rich, accurate and trusted data is at the epicenter of any successful business. All data goes through a lifecycle that starts from data collection and goes toward partitioning, cleansing, visualizing, analyzing, performing and sharing. Data cleansing in particular is a form of data management that involves reducing inaccurate, incomplete or irrelevant data. This ensures that all data is up to date, accurate and useful. Data cleansing primarily involves correcting and consolidating data, but it also includes monitoring, metadata management and information policy management. It ensures businesses go beyond just fixing data to solving the information challenge.
Why should organizations be deliberate in how they go about enterprise data cleansing?
McMahon: Digital business transformation is changing the conversation about data. It is a shift from 'data is not my problem' to 'data is everyone's opportunity.' Organizations are looking for ways to embrace the opportunity -- specifically by exploiting their information as a strategic enterprise asset. When organizations manage data quality proactively, they eliminate the risk to operations and analytics, but also create organization-wide information excellence and discipline. IT professionals can empower the business to understand, assess, analyze and improve the trustworthiness of data, and transform it into a strategic asset.
When data is presented at least partially cleansed, it is not only easier to understand, but it's much faster to analyze and bring about real insights for a business. When you take into account the different knowledge levels and various data backgrounds of whoever is working with the data, the time saved in this process could be substantial.
How do you do a data cleanse? What steps should IT pros follow?
McMahon: A good enterprise data cleansing strategy focuses on collaboration, transparency and business outcomes, and starts with creating a common understanding of data relative to your business.
Organizations should first start by conducting a data assessment -- similar to a doctor completing a health check or conducting tests before performing surgery -- to identify where the biggest needs are for data cleanup. Understanding how dirty data affects enterprise processes and performance can help you dramatically focus your data cleansing process to improve efficiency and profitability much more quickly. The key is to perform the data cleansing process before analyzing, moving or integrating data.
Next, it is all about transforming the data. Your data cleansing process should include workflows to:
- Parse, standardize and correct data.
- Validate data according to business rules and requirements.
- Enrich data with internal or external data sources to fill gaps within data you already have.
- Match and consolidate data by embedding data duplication checks directly into workflows or applications.
Once the initial data cleanse has kicked off, companies should continuously organize and keep their data clean and up to date. While it can seem tedious, cleaning data regularly will make it less overwhelming. More advanced artificial intelligence and machine learning tools are helping to make this process automated and more efficient.
What pitfalls should IT pros avoid when doing a data cleanse?
McMahon: Data cleanses should not be conducted in silos and without communication with key stakeholders. In this case, it's helpful to use collaboration tools to overcome the challenge of disparate data. Collaboration tools provide an opportunity for everyone to have visibility into data changes and alleviate the challenges that can come along with cleansing data.