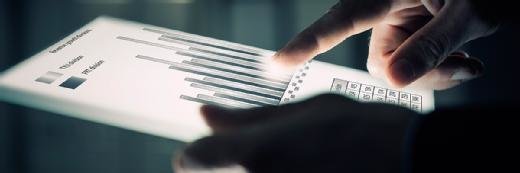
Sergey Nivens - Fotolia
11 features to look for in data quality management tools
As the need for quality data has increased, so have the capabilities of data quality tools. Learn how collaboration, data lineage and other features enable data quality.
In the early days of data warehousing, acute data quality issues drove the need to standardize and cleanse inconsistent, inaccurate or missing data values. At the time, the state-of-the-art data quality management tools mostly performed address standardization and correction coupled with data deduplication.
Conventional wisdom has since transitioned from trying to correct data to proactively preventing errors from being introduced. Instead of focusing on data cleansing, today's tools support practices for data governance and stewardship while operationalizing data quality assessment.
Capabilities to look for in data quality management tools include:
- Collaboration features: Team members may need to share data element definitions and data domain specifications, as well as agree on semantic meanings.
- Data lineage management: Data quality management tools can enable the organization to map the phases of the information flow and document the transformation applied to data instances along the flow.
- Metadata management: The tool should provide a repository to document both structural and business metadata, including data element names, types, data domains, shared reference data and contextual data definitions that scope the semantics of data values and data element concepts. This metadata environment should allow multiple data professionals to submit their input about these definitions to facilitate harmonious utilization across the enterprise.
Data quality management tools can help an organization cleanse, track, manage and govern data. - Data profiling for assessment: Data profiling capabilities in data quality management tools can perform statistical analysis of data values to evaluate the frequency, distribution and completeness of data, as well as the data's conformance to defined data quality rules. Data profiling tools can also be used to assess data quality.
- Data profiling for monitoring: Data profiling tools enable users to define the validity rules against which data sets can be tested. They also ensure that the data profiling facility is flexible enough to integrate proactive data quality validation to monitor compliance with defined expectations.
- Implementation of data controls: Data controls are operational objects that can be integrated directly into the information production flow to monitor and report on data compliance or the violation of defined rules. Many tools can automate the generation of data quality controls for application integration.
- Data quality dashboarding: Data stewards are tasked with monitoring data quality, taking action to determine the root cause of flagged issues and recommending remediation actions. A data quality dashboard can aggregate the status of continuously monitored data quality rules, as well as generate alerts to notify data stewards when they need to address an issue.
- Connectivity to many data sources: As the breadth, variety and volume of data sources expand, the need to assess and validate data sets originating from both within and without the organization has become acute. Look for data quality management tools that can connect to a wide selection of data source types, both from a system -- e.g., RDBMS vs. NoSQL database -- and a platform -- e.g., on premises vs. cloud -- basis.
- Data lineage mapping: Data lineage mapping scans the application environment to derive a mapping of the data production flow and to document the transformation.
- Identity resolution: Identity resolution is the process of linking various records and is the main engine for record deduplication, which can enable some aspects of data cleansing.
- Parsing, standardization and cleansing: Last, but not least, there is always going to be a need for the traditional aspects of data quality, such as address standardization and the correction of known invalid values.
Don't limit the evaluation to only these features, though. As data quality management becomes more collaborative, it will be important to document processes and allow for a collaborative review to ensure the auditability of the data quality procedures.
Reasonable data quality management tools do not only support the operational aspects of data stewardship, but they can also increase awareness of the value of high-quality information and motivate good data quality practices across the organization.