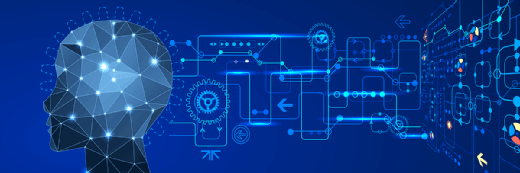
Managing unstructured data is crucial to enterprises' AI goals
Unstructured data makes up a huge portion of most businesses' data volume. But, with data-hungry AI systems coming online, making sense of these stores has never been more important.
For CenturyLink, customer surveys play an important role in understanding the customer experience. But, in the past, without the ability to analyze the unstructured data in these surveys, customer service teams couldn't drill down to identify the nature and characteristics of issues.
"Using unstructured data is really important for us because that's where the real detail -- the meat -- comes in," said Beth Ard, vice president of customer experience at the Louisiana-based network services provider. "When you do scoring, for example, you don't have enough actionable insight to make the changes that you need without it."
Most business analytics processes require clean and well-structured data, but enterprises are increasingly managing unstructured data formats -- emails, chat transcripts, audio and video, and social media posts.
By most estimates, unstructured data accounts for 80% to 90% of all data collected by enterprises.
But only 18% of companies are currently taking advantage of unstructured data, such as product images, customer audio files or comments from social media, according to a Deloitte survey released in July.
So, the race is on to extract intelligence from unstructured data, and many companies are turning to cloud platforms to make this happen.
According to another recent Deloitte survey, 39% of companies prefer to get technology like advanced analytics as a service, compared to 15% choosing an on-premises software.
In fact, the AI-as-a-service market is growing by 48.2% a year, according to Deloitte.
"Cloud-based software has the scalability, flexibility and agility to manage all the data and analysis we need to gather insights and act quickly," Ard said.
To make sense of all the customer feedback CenturyLink receives from customers, Ard's team turned to text analytics. By analyzing customers' messages and assessing them in natural language, the team could understand at a deeper level problems faced by customers.
But natural language text comes into the company through many other channels, Ard said. Customers often start with an email question, which can lead to an online chat with a live agent, and may eventually resort to a telephone conversation.
As a result, it was important for CenturyLink to get as much capability as possible from a single platform.
Ard said that the company decided to go with Qualtrics, a Utah-based experience management company that SAP recently acquired for $8 billion. The platform enables the company to analyze customer feedback and take actions to address problems more quickly, Ard said.
Traditionally, one way to manage unstructured data from customer service interactions is to have actual human beings read the emails, listen to the audio recordings and review the chat messages.
But, if a product manager is reading comments that a hundred customers left on a review website six months ago, she's not going to get a complete perspective of customer sentiment, said Sahil Sethi, head of product marketing at Qualtrics.
The data is going to be too old to be meaningful, and there will be too little of it for a representative sample.
But looking at larger data sets creates its own problems.
"Most organizations lack the resources or tools to parse the unstructured data," he said. "These are massive data volumes we are talking about."
Qualtrics offers a natural language processing engine to read the text of customer feedback and extract meaning from it automatically. "It helps parse the comments into the topics people are talking about or the sentiment that people have," Sethi said.
To train AI systems like Qualtrics', vendors typically start with a set of training data -- a set of customer emails, say, sorted by human beings as being "nasty" or "nice," or other descriptors. The system can then learn to differentiate new customer correspondences based on features it learned to identify in the human-sorted example sets.
As companies use AI more broadly and start to build their own AI models, the need to build some kind of structure into unstructured data will become even more important, according to Praful Krishna, CEO of Coseer, a San Francisco-based cognitive computing consultancy.
"To train, deep learning needs all the data annotated and tagged into a structure," he said. And it's not a process that can be done once and gotten out of the way, he added.
"It's very tempting to take the pain early on and impose a structure on fluid data," he said. "However, any structure that can possibly work must be slave to the problem at hand -- each question needs a different structure to find the answer."
Fortunately, AI also offers a possible solution to the problem of managing unstructured data, he said.
"Recent advances in artificial intelligence have enabled a highly granular search over any kind of unstructured information," he said. "It is possible to answer very specific questions and, using this capability, auto populate structured templates or tables."
This kind of approach to unstructured data could make a company future-proof, he said, and make technology more responsive to human needs.
"Our computer systems are as rigid as something can be -- literally thinking in absolutes of zeros and ones," he said. "But thought is anything but structured. It's our nature to work in a fluid, associative way, often without a clear path from A to B."