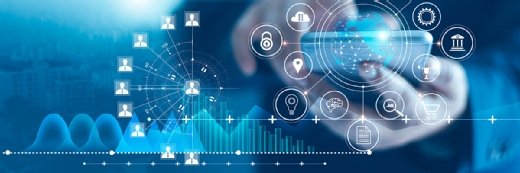
Getty Images/iStockphoto
Databricks steps up in competitive machine learning market
At its Data + AI Summit, Databricks introduced AutoML, glass box tools and Delta Lake-based Feature Store capabilities for its AI platform.
Independent AI vendor Databricks Inc. today unveiled two new capabilities for its AI platform -- AutoML and Feature Store -- to enable enterprise customers to better automate, discover and govern AI models.
The features will be immediately available on the Databricks cloud, executives of the San Francisco-based vendor said at the virtual Data + AI Summit, being held May 24-28.
The AutoML engine enables developers to speedily produce trained models from a user interface or API, and also makes the resulting code visible so customers can control, customize and enhance models, the vendor said.
The Feature Store
In addition to the "glass box" AutoML capability, the new Feature Store enables data scientists and business analysts to discover and reuse features with assured consistency, scattered across the enterprise, both with offline and real-time data, and within MLOps systems.
For instance, customers can reuse rather than reinvent features for models and be assured that the offline applications, such as training materials and real-time online engineered features, are maintained consistently, according to Databricks. The Feature Store is aimed at managing code changes that are often a source of friction among different teams in an organization.
Features are stored in the Delta Lakes open format storage layer and accessed through the native API of Delta Lake, Databricks' data lake system.
One analyst said the machine learning (ML) capabilities make Databricks' AI platform more competitive with AWS, Google Cloud, IBM and Azure, which currently provide mature AutoML capabilities, as well as with MLOps vendors such as DataRobot and H2O.ai.
"Databricks' AutoML capabilities will enable them to compete across a larger addressable market and bring differentiated value propositions for citizen data scientists, complementing their traditional mindshare dominance among enterprise architects and AI engineers," said Chirag Dekate, an analyst at Gartner. "AutoML capabilities are crucial in enterprise production journeys, especially for rapid prototyping, validation and deployment."
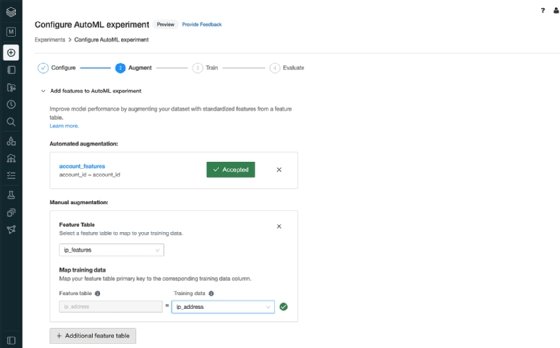
The Feature Store will also enable Databricks to more aggressively compete with specialized vendors such as Tecton, Feast, Iguazio, Logical Clocks, Splice Machine and Scribble Data, Dekate added.
Databricks and AWS
Databricks also partners with the major cloud vendors. At Data + AI on May 26, Matt Garman, senior vice president, worldwide sales and marketing at AWS, a Databricks partner, said John Deere and Comcast are among the pair's joint customers that have migrated from Hadoop clusters to Databricks on AWS.
While not commenting directly on Databricks' AutoML and Feature Store, Garman said customer demand is strong for unlimited storage and ML capabilities. "They want to let teams run ML and analytics and the right security and management," Garman said.
AutoML has become a must-have for all serious AI platform players, analysts said. Databricks' glass-box version of AutoML enables enterprise users to generate and customize ML models with full visibility into the development code.
"Most products give you a UI and drag-and-drop, but often have limitations. If you run out of power, it's [a limitation] and doesn't work for all real-life uses," said Clemens Mewald, director of product management, machine learning and data science at Databricks. "In addition to UI workflow, we auto-generate native code. You see code and [can] apply expertise and customize it."
Positive reactions
A data scientist familiar with Databricks said the enhancements will be useful.
"Both products will help to gain agility in order to develop data science models and govern the features that are used to generate them," said Matías Stanislavsky, data manager at Telecom Argentina, who worked with Databricks at his previous job. "I think it is the right step in the Databricks' platform."
And a data scientist and research analyst at TDWI agreed that Databricks is meeting customer demand for AutoML technology.
"As organizations look to move to unify their data and analytics in a platform, tools like AutoML should be part of the platform," said Fern Halper, vice president and senior director at TDWI Research for advanced analytics.
"Many organizations are trying to upskill their business analysts to perform data science, and tools like AutoML that help with feature engineering, model building and hyper-parameter tuning are increasing in importance," she added. "AutoML can also save the data scientists time."