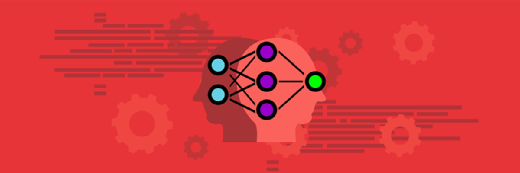
Combine ML with human intelligence for your security strategy
As hackers target the ever-increasing complexity of company networks, enterprises need to find a balance between machine learning and human intelligence when protecting systems and data.
Over the last decade or so, machine learning (ML) has helped to transform cybersecurity. With its ability to observe, contextualize and draw conclusions from data, ML can perform many critical security tasks and with greater speed and accuracy. This has tempted some to suggest their ML-powered solutions make human intelligence redundant. The truth is effective cybersecurity requires a balance of both human and machine.
Machine learning in cybersecurity
The commoditization of machine learning algorithms has been pivotal in curating the volume of alerts produced by organizations' security monitoring tools. As the volume of alerts these tools produce has grown, AI and machine learning have played an increasingly larger role in supporting analysts by sifting through security logs and automating other routine, time-consuming tasks. This has been fairly successful. Machine learning enables certain types of attacks to be identified and resolved more quickly, reducing the impact and cost on the organization. But rather than eliminating the need for human analysts, machine learning allows the security operations center to reclaim and reallocate human resources so they can focus more on threat analysis and responding to those destructive cyber threats.
Machine learning has also been critical for managing the explosion of new and more sophisticated malware. AV-TEST has identified more than 1 billion new malware samples in 2020 alone. Accurately identifying and classifying malicious files in this landscape isn't possible with the signature-based methods that were standard just five to 10 years ago. It has become essential to employ machine learning to process today's massive amount of data and spot deviations within it so analysts can respond efficiently and effectively.
Expanding applications through ML integrations
Machine learning is also taking on a greater role in identifying anomalous behavior. Hackers are taking advantage of increasingly complex company networks (e.g., cloud, containers, remote employees, mobile, IoT and other edge devices) to hide in plain sight. Without the manpower to ferret out unusual behavior among hundreds or thousands of user accounts, organizations are left vulnerable to manually executed attacks.
Machine learning is filling in these security gaps. By monitoring the behavior of every user within the organization, machine learning algorithms can learn what is normal, thereby allowing them to recognize what is abnormal. An employee logging into a server on a Saturday, when they've never previously accessed the network on a weekend, would be called out as suspect and generate an alert.
Human intelligence is still a critical part of the process, though. A human security professional is still needed to provide the right input model for the machine learning, to make sure that the model works on the right set of data, and to confirm it produces results that can be validated and make sense in a security context. This equilibrium is necessary to achieve accurate and effective security outcomes.
Perhaps more importantly, human analysis is necessary to verify each alert and determine the appropriate response. While machine learning can identify anomalies, it can't provide the context required to determine which are legitimate threats. The user in my earlier example may be working on an important project and just putting in extra hours to meet an impending deadline. Without a human analyst to recognize this, the user could be treated as an attacker and locked out of the account, causing both the user and the organization unnecessary headache.
The best of ML and human intelligence
Both machine learning and human intelligence play critical roles in an organization's desired security outcomes. The increase in security incidents coupled with the growing cybersecurity workforce gap makes machine learning essential for detection at scale. For detection to be trusted and effective, however, it needs to be validated by human expertise.
Few organizations have the security expertise and infrastructure to achieve these standards on their own. A proven managed detection and response provider will be able to augment an organization's security efforts with automated real-time incident detection and the human intermediation to verify complex security events prior to determining the most appropriate response. The addition of these capabilities is one of the best ways to harden your security posture and reduce the likelihood and impact of successful attacks.
About the author
Rohit Dhamankar is vice president of threat intelligence at Alert Logic. Dhamankar has over 15 years of security industry experience across product strategy, threat research, product management and development, technical sales and customer solutions. Prior to Alert Logic, Dhamankar served as vice president of product at Infocyte and founded consulting firm Durvaankur security consulting. He holds two Master of Science degrees, one in physics from the Indian Institute of Technology in Kanpur, India, and one in electrical and computer engineering from the University of Texas.