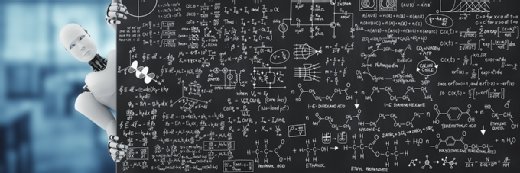
Blue Planet Studio - stock.adobe
How generative AI can make data visualizations accessible
Data teams can use generative AI to make data visualization creation approachable for business users of all technical skill levels -- if organizations can manage the challenges.
Innovations in generative AI offer capabilities that simplify data visualization workflows. Data teams that solve GenAI challenges can make data visualization experiences more accessible for all users.
GenAI improves visualizations by automatically identifying data needed for a specific audience and generating the text or images for the user. It can also analyze the visualizations to identify trends or relationships and accelerate time to insights.
However, GenAI is a work in progress. The industry is grappling with issues including hallucinations, bias and trust in results. As advancements in GenAI address these concerns, it has the potential to integrate into common data visualization uses.
GenAI uses in data visualizations
Data visualizations can integrate GenAI capabilities in several ways to improve performance.
Generate user-friendly charts
GenAI can help create the charts, graphs and graphics that analysts use to illustrate a data set. GenAI tools can recommend the best visualization for the data. Automated recommendations significantly lower the skill level for users trying to determine whether a bar graph, line chart or a Sankey diagram is the best tool for the job.
Provide insights from visualizations.
GenAI can automatically analyze large data sets to identify patterns and trends. The process can be faster than a human analyst, and it can identify patterns they might not detect. GenAI can also update and change the visualization in real time, but setting up dynamic updates requires more work from the data team.
Data exploration
Data exploration tells the story behind data with easy-to-read, visually informative and engaging charts. It also reveals potential problems and opportunities. Although the process improved with modern BI tools, it can still be overwhelming, especially for IT pros who aren't data scientists. Less data-literate users might struggle to start in a vast sea of data, and they might not know how to interpret what the data points really mean for their business.
GenAI, combined with data enrichment, can accelerate data exploration for tasks, such as analyzing unstructured data, geocoding, translation or combining data with external data sources. Finishing the tasks quicker leads to faster insights.
Data insights and actions
The goal of analytics is to reduce the time spent converting data to insights and actions. The process is an ongoing cycle as the actions can create new sets of data. GenAI can accelerate the process of transforming insights into actions, said Mike Lempner, vice president of engineering and technology at Richmond, VA-based credit card servicer, Mission Lane.
The process creates a feedback loop that provides a way to tell a story with the data. Visualizations can present the data in a more understandable way instead of relying on tabular or grid data. Visualizations make it easier for the data user to digest insights by highlighting similarities, anomalies and differences.
Streamlining analytics processes
GenAI can automate manual tasks, such as data segmentation and categorization, to streamline analytics processes, said Anthony Loss, director of solution strategy at San Francisco-based AWS consulting company, ClearScale.
For example, data democratization calls for the classification of teams with responsibilities related to their business outcomes. GenAI can quickly categorize large amounts of data input so data teams can focus on their individual tasks and responsibilities, which can eventually turn into a visualization.
Data visualization and GenAI challenges
Data analysts must manage the challenges of GenAI and visualizations to use both effectively.
Choosing a visualization
The first challenge an analyst encounters is determining which visualization to use, Lempner said. After choosing a visualization type, they must format and prepare the data for that specific visualization. GenAI enhances the productivity and efficiency of data teams by automating some of the tasks. It determines which type of visualization to use based on the characteristics of the data and available metrics.
Data prep and formatting
Another challenge is the preparation and formatting of data to answer various questions or perform different types of analysis. GenAI automation can reduce the time the process takes.
"What previously may have required eight steps to format data for GenAI a year ago may be down to a few simple steps as GenAI expands to handle more formats of input data and gains new capabilities each month," Lempner said.
Limited context windows
The context window is one of GenAI's metrics. Early models could only hold a few hundred words -- known as tokens -- in their working memory at a time. Context windows are improving, but some of the most popular models struggle with contextualizing data in the middle of a large request. The problems grow when teams try to process larger data sets, which might involve breaking data into consumable chunks using new tools such as LangChain or vector databases.
GenAI implementation increases in complexity with each additional data set factored into the analysis and visualization, Lempner said. With each new data set, it becomes more important to understand the relationship, quality and constraints of that data set and how it interacts with the other data sets inputted into the GenAI model.
Context
Understanding the context of the data is an ongoing challenge and which requires data users to have some subject matter expertise. For example, GenAI can generate visualizations from customer survey data. Users need to understand how data capture works to understand the quality of the data and insights, and identify any potential gaps.
George Lawton is a journalist based in London. Over the last 30 years, he has written more than 3,000 stories about computers, communications, knowledge management, business, health and other areas that interest him.