7 data modeling techniques and concepts for business
Three types of data models and various data modeling techniques are available to data management teams to help convert data into valuable business information.
A well-designed data model is the cornerstone of effective operational systems and BI and analytics applications that deliver business value by transforming enterprise data into a useful information asset.
Information is data in context, and a data model is the blueprint for that context. Data often has many contexts based on its use in different business processes and analytics applications. Therefore, an enterprise needs many data models, not just one that rules all uses. Various data modeling techniques are available to data management teams.
What are data models?
A data model is a specification of data structures and business rules. It creates a visual representation of data and illustrates how different data elements are related to each other. It also answers the who, what, where and why of the data elements. In a retail transaction, for example, the data model provides the details on who made the purchase, what was bought and when. The model might also contain additional data about the customer, product, store, salesperson, manufacturer, supply chain and much more.
It's best to build a data model in a top-down approach, from high-level business requirements to a detailed database or file structure. This approach uses the following three types of data models:
- Conceptual data model. A conceptual model identifies what data is needed in business processes or analytics and reporting applications, along with the associated business rules and concepts. It doesn't define the data processing flow or physical characteristics.
- Logical data model. This model identifies the data structures, such as tables and columns, and the relationships between structures, such as foreign keys. Specific entities and attributes are defined. A logical data model is independent of a specific database or file structure. It can be implemented in a variety of databases, including relational, columnar, multidimensional and NoSQL systems -- or even in an XML or JSON file.
- Physical data model. A physical model defines the specific database or file structures that will be used in a system. For a database, that includes items like tables, columns, data types, primary and foreign keys, constraints, indexes, triggers, tablespaces and partitions.
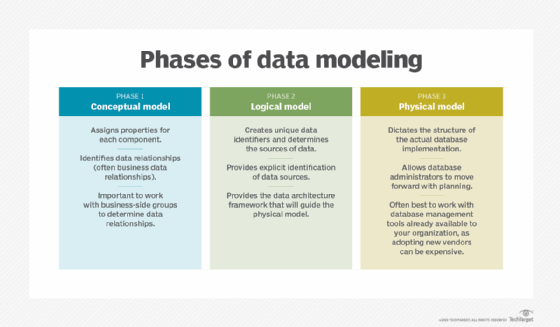
Common data modeling techniques and concepts
To better understand today's popular data modeling techniques, it's helpful to provide a quick history lesson on how modeling has evolved. Among the seven data models described here, the first four types were used in the early days of databases and are still options, followed by the three models most widely used now.
1. Hierarchical data model
Data is stored in a tree-like structure with parent and child records that comprise a collection of data fields. A parent can have one or more children, but a child record can have only one parent. The hierarchical model is also composed of links, which are the connections between records, and the types that specify the kind of data contained in the field. It originated in mainframe databases in the 1960s.
2. Network data model
This model extended the hierarchical model by allowing a child record to have one or more parents. A standard specification of the network model was adopted in 1969 by the Conference on Data Systems Languages, a now-defunct group better known as CODASYL. As a result, it's also referred to as the CODASYL model. The network technique is the precursor to a graph data structure, with a data object represented inside a node and the relationship between two nodes called an edge. Although popular on mainframes, it was largely replaced by relational databases after they emerged in the late 1970s.
3. Relational data model
In this model, data is stored in tables and columns and the relationships between the data elements in them are identified. It also incorporates database management features such as constraints and triggers. The relational approach became the dominant data modeling technique during the 1980s. The entity-relationship and dimensional data models, currently the most prevalent techniques, are variations of the relational model but can also be used with non-relational databases.
4. Object-oriented data model
This model combines aspects of object-oriented programming and the relational data model. An object represents data and its relationships in a single structure, along with attributes that specify the object's properties and methods that define its behavior. Objects may have multiple relationships between them. The object-oriented model is also composed of the following:
- Classes, which are collections of similar objects with shared attributes and behaviors.
- Inheritance, which enables a new class to inherit the attributes and behaviors of an existing object.
It was created for use with object databases, which emerged in the late 1980s and early 1990s as an alternative to relational software. But they didn't make a big dent in relational technology's dominance.
5. Entity-relationship data model
This model has been widely adopted for relational databases in enterprise applications, particularly for transaction processing. With minimal redundancy and well-defined relationships, it's very efficient for data capture and update processes. The model consists of the following:
- Entities that represent people, places, things, events or concepts on which data is processed and stored as tables.
- Attributes, which are distinct characteristics or properties of an entity that are maintained and stored as data in columns.
- Relationships, which define logical links between two entities that represent business rules or constraints.
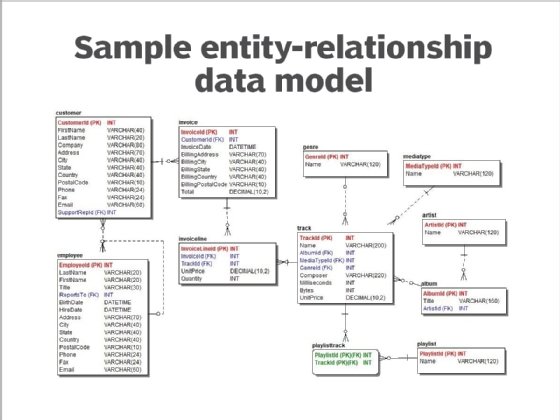
The model's design is characterized by the degree of normalization -- the level of redundancy implemented, as identified by Edgar F. Codd, who created the relational model. The most common forms are third normal form (3NF) and Boyce-Codd normal form, a slightly stronger version also known as 3.5NF.
6. Dimensional data model
Like the entity-relationship model, the dimensional model includes attributes and relationships. But it features two core components.
- Facts, which are measurements of an activity, such as a business transaction or an event involving a person or devices. Facts are generally numeric, and fact tables are normalized and contain little redundancy.
- Dimensions, which are tables that contain the business context of the facts to define their who, what, where and why attributes. Dimensions are typically descriptive instead of numeric.
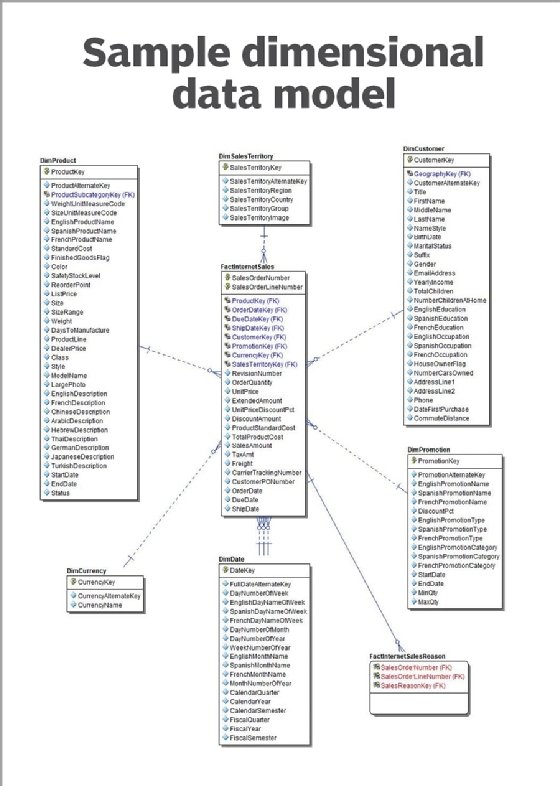
The dimensional model has been widely adopted for BI and analytics applications. It's often referred to as a star schema -- a fact surrounded by and connected to multiple other facts, though that oversimplifies the model structure. Most dimensional models have many fact tables linked to many dimensions that are referred to as conformed when shared by more than one fact table.
7. Graph data model
Graph data modeling has its roots in the network modeling technique. It's primarily used to model complex relationships in graph databases, but it can also be used for other NoSQL databases such as key-value and document types.
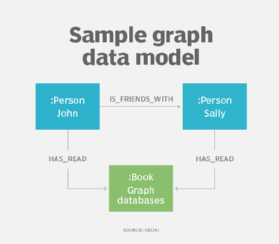
There are two fundamental elements in a graph data model.
- Nodes, which represent entities with a unique identity. Each instance of an entity is a different node that's akin to a row in a table in the relational model.
- Edges, also known as links or relationships. They connect nodes and define how the nodes are related. All nodes must have at least one edge connected to them. Edges can be undirected, with a bidirectional relationship between nodes, or directed, in which the relationship goes in a specified direction.
One of the more popular graph formats is the property graph model. In this model, the properties of nodes or edges are represented by name-value pairs. Labels can also be used to group nodes together for easier querying. Each node with the same label becomes a member of the set, and nodes can be assigned as many labels as they fit.
Data modeling best practices
Best practices for data modeling include eight steps that can help an enterprise derive the desired business value from its data.
Treat the data model as a blueprint and specification. Data models should be a useful guide for the people who design the database schema and those who create, update, manage, govern and analyze the data. Follow the progression from conceptual to logical to physical models if a new data model is being created in a greenfield environment with no existing models or physical schemas.
Gather both business and data requirements upfront. Get input from business stakeholders to design conceptual and logical data models based on business needs. Also, collect data requirements from business analysts and other subject matter experts to help derive more detailed logical and physical models from the business requirements and higher-level models. Data models need to evolve with the business and technology.
Develop models iteratively and incrementally. A data model may include hundreds or thousands of entities and relationships. That would be extremely difficult to design all at once. Don't try to boil the ocean. The best approach is to segment the model into the subject areas identified in a conceptual data model and design those subject areas one by one. After doing that, tackle the interconnections between them.
Use a data modeling tool to design and maintain the data models. Data modeling tools provide visual models, data structure documentation, a data dictionary and the data definition language code needed to create physical data models. They also can often interchange metadata with database, data integration, BI, data catalog and data governance tools. And if there are no data models on existing databases, leverage a tool's reverse-engineering functions to jump-start the process.
Determine the level of granularity that's needed in data models. In general, maintain the lowest level of data granularity -- in other words, the most detailed data that's captured. Only aggregate data when necessary and only as a derivative data model, while still retaining the lowest-grain data in the primary model.
Avoid extensive denormalization of databases. Denormalizing a database adds redundant data to optimize query performance. But it assumes a specific relationship between entities that may limit its usefulness for different analytics applications. As with aggregation, when denormalization is used, it's best applied in a derivative data model -- for example, in a schema for a BI application rather than in a data warehouse.
Use data models as a communication tool with business users. A 10,000-table entity-relationship model can make anyone's head spin. But a data model, or a portion of one, focused on a specific business process or data analysis offers the perfect opportunity to discuss and verify the schema with business users. The assumption that business users can't grasp a data model is a fatal mistake in modeling efforts.
Manage data models just like any other application code. Enterprise applications, data integration processes and analytics applications all use data structures, whether they're designed and documented or not. Rather than allow an unplanned "accidental architecture" to develop and crush any chance of obtaining a solid ROI from their data, organizations need to get serious about data modeling.
Editor's note: This article, originally published in 2021, was updated by TechTarget editors in July 2024 to improve the reader experience.
Rick Sherman, who died in January 2023, was the founder and managing partner of Athena Solutions, a BI, data warehousing and data management consulting firm. He had more than 40 years of professional experience in those fields.