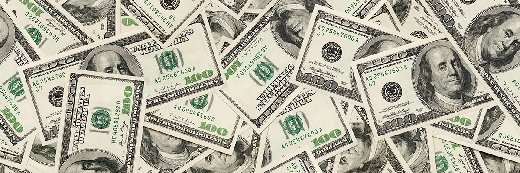
KazantsevAlexander - Fotolia
Fivetran raises $100M to advance enterprise data integration
The co-founder and CEO of Fivetran talks about what the vendor is doing to help organizations meet the challenges of enterprise data integration.
Among the most common approaches to data integration is extract, transform and load (ETL). However, the process can sometimes be hard to set up and configure.
That's the enterprise data management niche in which Fivetran specializes with its automated data integration platform. Fivetran, based in Oakland, Calif., was founded in 2012 and has grown steadily, with a data connector approach that aims to make it easier to bring different data sources together for analysis.
On Tuesday, Fivetran marked the next major phase of the its growth, disclosing that it had raised $100 million in a Series C round of funding led by Andreessen Horowitz and General Catalyst. The new financing will help the vendor to keep building out enterprise data integration technologies as well as expand its sales and marketing.
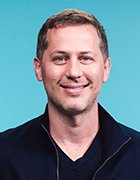
In this Q&A, George Fraser, co-founder and CEO of Fivetran, discusses the evolution of the enterprise data integration business and where the vendor is headed.
Why are you now raising $100 million in the middle of the global COVID-19 pandemic?
George Fraser: We're still plugging along doing well, despite the economic uncertainty. We haven't been unaffected by it, but we've been only a little affected by it.
Difficult times can also be an opportunity. It's an opportunity to hire a lot of great people who maybe aren't getting chased by as many companies as they usually are. So we received an opportunity to come up stronger on the other side of economic uncertainty and decided to go ahead and raise money now.
What have been the biggest changes in the enterprise data integration business landscape since you started Fivetran?
Fraser: One change is that businesses are using more and more tools. So the problem we solve of getting all your data together has just gotten harder and harder.
The other trend is the decreasing cost of compute and storage in the cloud, and the move of companies to the cloud, particularly in the analytics space, with the rise of Amazon Redshift, and then Snowflake and [Google] BigQuery.
Our approach, which is all about automation, is somewhat more compute- and storage-hungry than a more customized approach. So the resource tradeoff has become dramatically more favorable for us over time. Customers today prefer to choose more automation, even if it uses a little more storage and more compute. It doesn't matter because the cost of those is now low.
What do you see as the difference between Fivetran's ETL approach and data virtualization?
Fraser: So the difference in the technical implementation between an ETL approach and data virtualization is that with ETL, you move the data. With data virtualization, the data stays where it lives, and you just read it on demand. The problem with data virtualization is that it's often just too slow for many data sources.
The other dimension is the user experience. With a traditional ETL tool, the user experience is very complicated; you have to do lots of setup and maintenance and specify all of the details of how you move the data. Whereas in a data virtualization approach, the user experience is you just connect and presto, you can immediately start querying.
Fivetran is an interesting remix of the space. The technical implementation is like ETL. We move the data, but the user experience is like data virtualization, as it feels like we're not moving the data because you just turn it on and can query data quickly.
The way it works is there are connection wizards. So if I'm connecting to Salesforce, it's just a click-through to where you grant permission to access your Salesforce data. Then what happens is there is a little delay while we backfill all the historical data. It takes anywhere from a few minutes to a few days to backfill all the historical data. But then once we complete the backfill, all of our connectors are based on change capture, so they're just capturing what has changed.
What do you see as the big challenges of enterprise data integration in 2020?
Fraser: I think the challenge of just getting all your data in one place has gotten a lot easier. Data warehouses, like Snowflake, BigQuery and Redshift, are so fast and so cheap that you can just kind of centralize everything.
A lot of the challenges now are on the analyst side. The biggest problem that you still have to solve as a company is just making sense of all the data.
What's next for Fivetran?
Fraser: The primary thing we're doing is continuing this journey of providing automated connectivity to every single data source that businesses use. So we have about 150 connectors today. We probably need to have, in 10 years, like 10,000, so it is it is a giant mountain that we are partway up, and we're not stopping.
Then there's a lot of work around security and supporting all of the configurations that large enterprises use in the cloud. So we need to support all those variations. That's a big category of work for us over the next year as well.