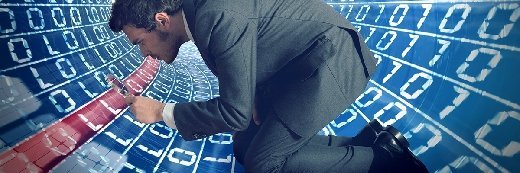
alphaspirit - Fotolia
GDPR mandates push data quality improvement into IT spotlight
Data quality issues cost companies significant amounts of money in lost revenue and added expenses, and their impact will only get bigger when the EU starts enforcing its GDPR law.
Data quality has always been the ugly duckling of the data management world. IT professionals are keen to get involved in projects involving big data, machine learning and other trendy technologies, but few queue up to volunteer for a data quality audit.
That isn't particularly surprising: Checking large data sets for errors and other issues, and then fixing the problems, can be tedious work. Yet the consequences of not paying attention to data quality are serious -- and they're likely to become even more serious once the European Union's wide-ranging General Data Protection Regulation (GDPR) takes effect.
There's ample evidence of the need for stronger data quality improvement efforts in organizations. For example, a Harvard Business Review article published in September 2017 detailed an executive education exercise in Ireland that involved 75 business managers from different industries assessing 100 recent data records from their departments for errors. One-quarter of the participants found inaccuracies in 70 or more of the records they checked; just two managed a score of at least 97 error-free records, the level deemed acceptable by the article's authors. Bear in mind that pretty much all enterprise data quality initiatives have targets of 95% accuracy or higher.
Perhaps the most famous data quality blunder to date involved the Mars Climate Orbiter, which was launched by NASA in 1998 at a total cost of $327 million. The spacecraft was supposed to collect data about the atmosphere of Mars. Alas, due to a ground-based software component using the wrong unit of measurement for pressure -- imperial vs. metric units -- it entered the Martian atmosphere at the wrong trajectory in September 1999 and was incinerated.
Poor data quality takes a financial toll
Businesses may not experience a flameout of that magnitude, but IT departments waste considerable staff time trying to find and correct problems with data accuracy, timeliness, completeness and consistency. Business operations also spend time unproductively resolving returned deliveries and fixing incorrect payments that result from data quality errors. Systemic problems can affect the reputation of businesses and their brands -- and hit them where it counts, in the corporate wallet.
A 2016 study of 272 U.K. companies by postal company Royal Mail's data services unit found that 70% of the participants had incorrect or out-of-date customer data, with estimated costs caused by data quality problems equaling an incredible 6% of their corporate revenue on average. The 2017 version of the survey showed similar results. Meanwhile, 29% of its 281 respondents pointed to GDPR compliance as their biggest customer data management challenge, topping the list of such challenges.
To be sure, some estimates of the cost of low-quality data need to be taken with a pinch of salt. A much-quoted IBM report from 2016 estimated that the U.S. economy alone loses $3.1 trillion to data quality issues annually, which seems implausibly high given that the combined profits of all U.S. corporations that year were only $2.1 trillion. Nonetheless, there's little doubt that businesses are being affected in a big way, and the situation appears to be barely improving, if at all.
Enterprises have long struggled with data quality improvement in systems built on mainstream relational databases, and the challenges are further complicated now by the proliferation of corporate data in Hadoop clusters and other big data systems, as well as spreadsheets and Word documents.
Also, as more and more data comes from outside the corporate firewall, there's the issue of reconciling internal and external data records. For example, you want to be sure that a certain Dun & Bradstreet company number with a particular credit rating refers to the same company as the one in your accounts payable system, especially if you're about to make a loan or extend credit to that company.
GDPR lays down the law on data
If there's good news, it's that regulations can stir management into action. GDPR comes into force in the EU on May 25, 2018, with the threat of large fines for organizations that fail to keep proper track of the personal data they hold on customers and whether people have consented to the use of their data. Businesses must also be able to process requests from customers to review or delete their data.
Customer data is typically scattered among numerous applications. Even within a single ERP system, there may be many separate instances of fields, like date of birth. Under GDPR, organizations should be able to state with confidence where all such personal data resides and show that it's properly governed. How confident is your company about being able to do so?
To that end, a positive side effect of the GDPR mandates may be stimulating interest in data quality improvement processes and the often neglected assortment of software tools that can profile data and help find duplicate entries and anomalies in data sets.
Data quality software has been around for decades, much of it aimed at tackling the humble problem of fixing incorrect names and addresses of customers and suppliers. Built-in algorithms can detect likely data entry errors, such as missing postal codes that need to be filled in. They'll also recognize that separate entries for Andy Hayler and Andrew Hayler with the same address are probably duplicates.
Software deployed in response to a regulatory issue like GDPR can also be used to improve data quality in many other ways. It's a pity that governments need to nudge corporations into investing in data quality improvement initiatives that are in their own best interest anyway. However, such nudging may turn out to be one of GDPR's best qualities.