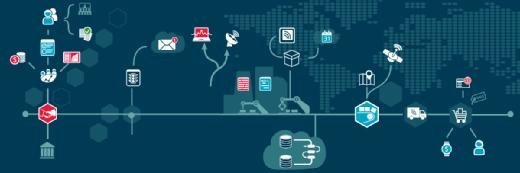
Fotolia
AI at the edge spurs decentralization, IoT interconnectivity
As AI spreads into most enterprises, it's imperative that devices or programs can make immediate smart decisions. Localized AI at the edge is aiming to tackle the lag.
As the internet of things has rapidly proliferated, edge computing has been introduced to accommodate and infuse localized data centers with processing power. As the combination of IoT and edge computing has extended the utility and potential of internet business operations across the board, artificial intelligence is looking to similarly expand.
The potent enterprise blend of IoT, edge computing and AI yields something new on the digital horizon -- distributed intelligence. The fresh and intriguing realm of possibilities for machine learning in internet-based apps and services is supported through decentralized processing and subsumption approach to information.
Moving to the edge
distributed computing intelligence is enterprise-level problem-solving power broken down into localized chunks, each designed to focus on a particular need. Rather than a centralized big data or machine learning infrastructure, the enterprise deploys LAN AI services in various physical sectors of the business: warehouses, factory floors, traveling fleets and employees in the field.
AI is being ushered into these localized, often peripheral regions by a number of factors. First, there is an increased need for real-time decision support and spontaneous adaptations in a specific process. In human-machine hybrid decision systems -- such as customer support -- immediate sentiment analysis is the ideal solution. There is no time for round trips to the cloud with copious local data that needs to be parsed immediately.
Secondly, model creation for AI training specific to IoT data is best accomplished in the location where the modeling is happening, rather than in a remote simulation -- thus, recurrent networks are ideal for working with time-series data.
Many experts believe AI at the edge will overtake and eventually dwarf current, cloud-based machine learning systems. In a blog titled, "The Edge Will Eat the Cloud," Gartner analyst Thomas Bittman noted that as engineers, workers and consumers become heavily concerned with latency issues and full automation, the cloud will give way to centralized, local AI at the edge.
Places far from data centers operate very simply and sparsely. AI at the edge wants those environments to change with the flow of data creating real-time responses to their occupants.
What does AI at the edge mean for software? For one thing, digital twins -- models of objects and environments to which comparisons of actual objects and environments can be made -- become far more impactful on performance metrics and predictive analytics if the model is right there in the environment, rather than back at headquarters.
Evolving AI
AI at the edge isn't just AI in a new place; it's a new kind of AI: a real-time, localized intelligence that can adapt in the moment or support spontaneous decisions. Streamed data from IoT can -- while on the edge -- trigger a process change on the spot immediately, then pass the metadata from the response back to the home cloud after the fact. AI's decisions in the field that suffer from uncertainty or latency can be analytically bolstered when intuition and existing data isn't enough.
Moreover, today's analytics in the field tend to be straightforward use cases, single-point services that have grown up organically. But that simplicity can't hold out much longer. Local solutions to issues in stores, factories and on the road must increasingly take one another into account, as each AI-inspired evolution affects other facets of an enterprise. This calls for a smarter, more sophisticated edge where everything of concern to the enterprise is within AI's purview.
Two of these environments -- the factory or warehouse and traveling vehicles -- are rapidly becoming spinoff AI edge industries in themselves: the industrial internet of things and the automated driving system. Local, real-time AI in these areas is quickly turning into an enterprise competitive advantage, with its own standards and practices. These industry uses of distributed intelligence are growing so rapidly that they can currently be autonomous and self-justifying, and not dependent on enterprise cloud systems.
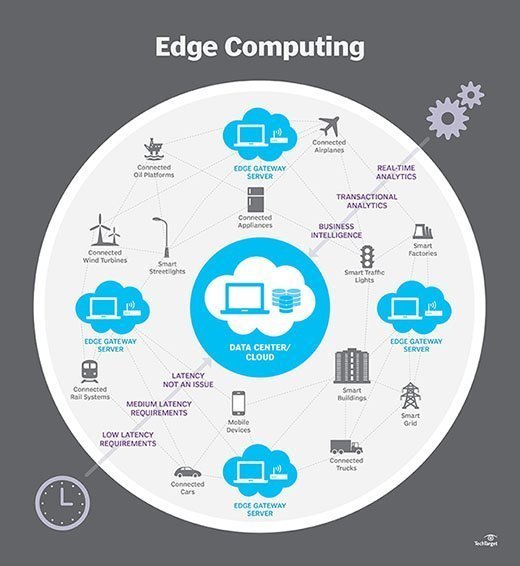
New challenges
Despite the positive inertia and obvious ROI, distributed intelligence, IoT and the edge are still a Wild West frontier, with too many options and not enough best practices. There are many unsolved problems -- such as no clear way to distribute processing between servers, gateways and IoT devices efficiently.
Security becomes another problem. With so many devices connecting to enterprise systems, AI at the edge creates a far greater risk of potential attack than most companies normally face. Diligence with gateway access is a point of concern, and the devices themselves require firmware updates, creating a new layer of maintenance burden.
Tackling these problems will mean that security will have to gradually shift privacy control upfront -- images, voices, video and other personal data that is being gathered must be scrubbed into compliance before it gets sent to headquarters. The operational reliability of IoT-based systems -- hampered today by the awkward sprawl of device types -- will eventually improve, as performance is increasingly mitigated by local intelligence.
These are -- relatively speaking -- good problems to have. The IT and security industry and its AI creations are beginning to make each other better, and improving the AI in the world will become easier as the world around us gets smarter.