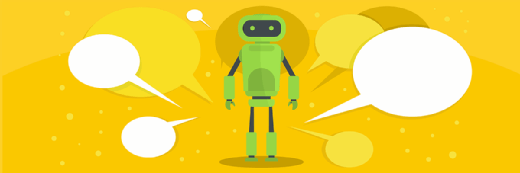
Can the future of voice assistants include enterprise use?
Voice assistants haven't had the success that companies originally expected. AI and machine learning could go a long way to boost this technology.
With Apple's introduction of Siri in 2011, the virtual voice assistant was launched. A voice assistant uses voice recognition, natural language processing and speech synthesis to answer questions and assist users -- typically through phones and voice recognition applications.
Despite major companies creating their own versions – Google's Home and Microsoft's Cortana -- the overzealous expectations for voice assistants have yet to be met. Voice assistants are used sparingly in enterprise, retail and professional settings.
Despite the slow burn, experts are now looking to advanced AI and machine learning to ensure the future of voice assistants.
Origins of voice assistants
Voice assistant technology, ultimately made up of conversational interfaces powered by NLP, derives from consumers' desire for 24/7 service. Devices can provide answers, directions and content across time zones and service hours. Thus, the technology took off in personal and home use in this niche performance.
Consumers expect to have the ability to ask a question, and get answers -- not just links, Will Hayes, CEO of Lucidworks, California-based enterprise search technology company, said.
"It's much more efficient to talk to a real person and get an answer rather than combing through a long list of FAQs," Hayes said.
This desire for a back-and-forth opened the door for the development of voice assistants. But when a voice assistant struggles to accomplish the task of simulating conversation or when it fails to answer a question, the illusion is broken.
Explaining the struggle
With the widespread adoption of voice technology for home use, alternate use is surprisingly sparse, despite a need for better search in the enterprise. Specific answers are often lost in the reports, papers and documents on websites, and being able to communicate directly what you are searching for is where voice assistants could excel.
To this point, however, relying on a voice assistant that can only handle simple and structured questions has not been a viable answer to more complex issues. Part of what has held these voice assistants back is what had previously led to their rise.
"There's a lot of low-hanging fruit being handled by simple implementation of rules, or 'if, then' statements," Hayes said. "But building too many rules over the years built up reactions that are manual and heavy."
Creating a voice assistant and assigning it answers to specific questions doesn't allow for dynamic adaptation capabilities and is very simple AI technology. This process can also become too time-consuming because it requires continuous curation of rules and constant review.
Hayes says while it may help with 20% of frequently asked questions, it doesn't provide adaptation for the remaining 80% of queries. These then lead to phone calls and human interaction, which slows the process even more.
This all boils down to the data and the approach companies take when it comes to voice assistants.
"Voice assistants' failure in the enterprise so far is not a problem, but a symptom of the larger problem: Enterprises are not efficient at managing data, information and insights holistically," said Radu Miclaus, director of product, AI and cloud of Lucidworks.
The future of voice assistants
Voice assistants require more complexity in order for them to become a fixture in the enterprise. Using AI, machine learning and NLP, voice assistants can handle a greater diversity of questions. These assistants must reach a level of semantic understanding in order to be utilized more effectively.
"Filtering data through natural language processing (NLP) capabilities [can] help assistants map users' intentions to information," Hayes said. "A question with 'where' may be looking for a response about a location. A 'how' question is searching for a procedure. And a 'when' question is likely time-related, or a date."
It begins with preparing data in a way that allows it to be adaptable to machine learning. Working from the ground floor of voice assistants (the data they utilize) would require great investment of time and resources but would increase adaptability.
"Successful implementations of voice assistants in the consumer space is a result of most of these applications being deployed against publicly available data hosted on websites or apps," Miclaus said. "There is a lot of data and information standardization done on websites and apps for the sole purpose of data find-ability, making this info ripe for the picking by voice assistant layers using NLP and NLU."
The goal of the voice assistant has not just been to answer questions from customers and users but to act as a conversational interface and make it appear as though the user is speaking with someone or something that can understand their issue and provide a result. AI, machine learning and NLP are the options to move voice assistants closer to this goal.