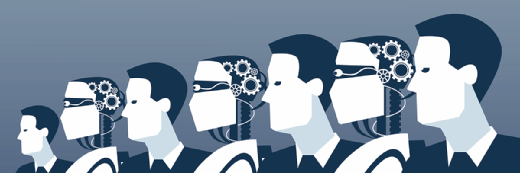
Collaborative robots' safety stalls enterprise implementation
Cobots are promising big gains, especially in enterprises utilizing manual labor. However, due to a number of safety concerns, human workers are still at risk.
Researchers and vendors are working on new AI technologies for robots that can not only work around people, but collaborate with them. However, as the technology advances, they're coming up against major challenges in the collaborative workforce.
One of the main benefits of collaborative robotics, or cobots, is humans, bots and the AI that runs on robots each have different strengths, which can complement each other. If grasping and manipulating items in a warehouse or store is difficult for robots, humans can step in to augment the process. At the same time, observing, counting, accurately measuring and comparing over time are tedious for people and straightforward for robots.
Experts see many growth opportunities for collaborative robotics. Early adoption in verticals like surgery, manufacturing, supply chain, warehousing, assisted living and hazardous materials cleanup have already proved successful. But, as implementation grows, safety precautions, rigorous training and enhanced communication will need to follow, particularly as robots grow in size, complexity and their ability to operate in the real world.
What can cobots do?
Collaborative robots have already seen major success in enterprise use settings ranging from mining to retail operations. Robotic AI ultimately helps inform and enhance human work, and there's a constant collaboration between what bots can do and what humans excel at.
"While there may have been some degree of human-robot separation in the past, we have now entered what we call the 'age of with,'" said Beena Ammanath, AI managing director at Deloitte Consulting LLP.
In robotic-assisted surgery, AI can make more precise cuts and movements, potentially leading to much less human error. But AI cannot make medical decisions. AI is also able to see things that humans can't, such as minute changes in skin color that suggest medical conditions.
Warehouses have been one of the earliest adopters of collaborative robotics, because many use cases are relatively simple, and safety issues are straightforward. The robots take on menial, repetitive tasks, so staff can be upskilled and undertake more rewarding, mindful work. Automated processes, in which a robot can pick items in supersized warehouses, will help control costs and enhance competitiveness for retailers.
Mario Harik, CIO at XPO Logistics, a transportation and logistics service in Greenwich, Conn., said their use of collaborative robots has significantly increased productivity with picking, packing and sorting tasks and reduced fulfillment time from multiple hours to 20 to 40 minutes. After redesigning the workflow in a way that made the best use of existing robotic technology, human productivity increased by four to five times and eliminated walking time by nearly 80%.
"In short, people alone can't do it. Technology alone can't do it. It takes humans and machines working together in a designed system to gain an advantage," Ammanath said.
Robotic safety processes
One thing both vendors and analysts agree on is collaborative robots' safety risk if not adequately trained. One of the biggest challenges with robots working around people is completely ensuring a robot will not harm a human worker, said Chris Harlow, director of product development at Boston-based Realtime Robotics, which develops processors for robotic motion planning.
Modern robots are only capable of doing a set of steps -- if a robot is told to pick up a package from one location and drop it in another location, it will do the action regardless of whether there is a person or object in the way. Ensuring worker safety sometimes requires limiting a robot's capabilities.
"Cobots tend to be weaker and slower moving than their industrial robot counterparts," Harlow said.
AI can help cobots learn as they go along to improve their function. AI and machine learning are helping robots better identify what objects they are picking up and the best way to pick those objects up, but safety is still a primary concern. Enterprises cannot afford to let robots learn by trial and error when human workers are alongside them, Harlow said. Simply put, any robot and human collision is unacceptable.
As of now, robotic movements must be completely deterministic so humans are sure of what the robot will be doing to assure worker safety. Improving algorithms through better semantic labels for characterizing the objects in the environment can boost worker safety without limiting function, Harlow said.
Descriptive labels make it easier to train algorithms to understand the mechanics of items, including differences that come with moving a pallet of ball bearings versus feather pillows. Motion planning algorithms could also benefit by moving to dedicated hardware, which can improve the response time to changes in the environment.
Another big challenge lies in solving for multiple edge cases that allow robots to safely navigate in complex and dynamic environments, said Phil Duffy, vice president of innovation at Brain Corp., a robotic OS provider based in San Diego. Each new environment introduces a multiplicity of edge cases that must be recognized and solved in order to ensure safe operation.
One strategy being pursued is to allow the robots to each gather data about newly discovered edge cases, which can be used to train other robots in the fleet. The larger the fleet, the larger the set of training data that's shared across the network. This is facilitating the evolution from isolated industrial robots to autonomous mobile robots that work collaboratively alongside people, Duffy said.
They are also experimenting with a learning-by-demonstration paradigm, which enables the end user to easily teach the robot before having it perform in autonomous mode. An operator has the choice to train the machine to perform some behaviors autonomously and perform other tasks manually based on the perceived risks.
Facilitating communication
One of the big challenges for AI researchers is finding ways for humans and robots to create a framework for communicating. Researchers are starting to explore different techniques for bringing communication to robots that could allow for free-flowing interaction.
"Communication between robots and humans will be crucial for many tasks," said Chad Edwards, Ph.D., professor of communication and co-director of the Communication and Social Robotics Labs at Western Michigan University.
An early example was the way Baxter, a social manufacturing robot, used eye movements to indicate which direction it was going to move on an assembly or packing line. Edwards said he believes more work needs to be done on understanding how humans use natural language that takes advantage of a shared context to facilitate collaboration. His research has found that people expect similar communication styles and patterns when communicating with robots as they do with humans.
"We don't speak in simple commands, but rather starts and stops, change directional flow and vocal fillers," Edwards said.
Heightening communication can also allow the robots and humans to have distinct goals that are separated by well-defined boundaries, according to Richard Schwartz, founder and CEO of drone developer Pensa Systems, based in Austin, Texas.
Schwartz said he expects to see the development of algorithms that allow humans and robots to negotiate specific tasks and actions when interacting in the same place at the same time. This will require teaching the robot to anticipate unexpected or even illogical actions and while protecting human workers and regaining control over completing a portion of the overall task.
Opening the black box
Robotic developers will also need to invest more time in understanding and communicating the ethical dilemmas raised by different kinds of collaboration, argued Alexander Wong, chief scientist and co-founder of AI platform DarwinAI, based in Waterloo, Ont. There are a lot of ways collaborative robots' safety can be compromised when manipulating physical objects in noisy, real-world environments.
"AI systems have advanced tremendously in the past decade, particularly in the area of deep learning. But the navigation of ethical decisions is still in the early-to-adolescent stage of development," Wong said.
Heuristic-driven AI systems typically navigate ethical decisions with simple, static rules that are hardcoded by the creators. As a result, the morals and values of a system are often a reflection of their creators, which can be faulted by natural human unconscious bias. As AI has evolved from heuristics-driven systems to machine-learning-driven systems, where the AI learns the rules directly from data itself, the system's morals and values are a reflection of the data it is trained on and the labelers who annotate it.
The systems are often termed black boxes, because it is not clear how they use the underlying data to reach particular conclusions. These ambiguities make it difficult to determine how or why a system is acting the way it is. For robots to begin making ethical decisions, developers need to open the black box for more communication between labelers and machine learning bots. Opening the black box of AI to explain how a system came to a conclusion will be critical for implementation and collaboration with humans in the future, especially in heavily regulated industries, or where human lives are at risk.
"An important first step toward safe and ethical AI-driven robots is the ability to understand and explain the factors behind AI decision-making so they can be configured to adhere to specific moral codes," Wong said.