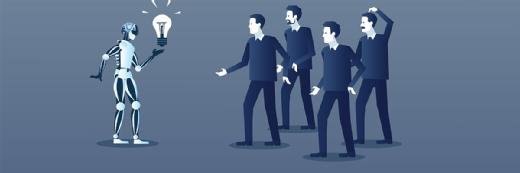
Lessons learned from 3 AI use cases
Sharing use cases is a form of storytelling, and that may be a key to proving just how valuable AI can be for companies, according to Gartner's Erick Brethenoux.
Use cases show rather than tell. For cutting-edge technology, such as machine learning and natural language processing, seeing AI technologies in action is a chance to see the value they can create.
Erick Brethenoux, research director at Gartner, described AI use cases as a form of storytelling that helps illustrate how the technology works, while demonstrating that, "despite the hype you hear about, AI is a real thing."
Brethenoux recently published a new research note on AI use cases, the last in a four-part series on AI. He shared several AI use cases to demonstrate key takeaways for companies just getting started. Here are three of them.
Inspiration from the outside
"One thing I often encourage my clients to look at is that you may want to go beyond your industry to find inspirational use cases," Brethenoux said during a webinar that accompanied his report.
A few years ago, Brethenoux was traveling across Europe with a friend who also happened to be chief engineer for a fighter jet engine manufacturer. His friend was giving a presentation on how AI has created a more intelligent cockpit with optimization technologies and Sensor fusion, software that combines data from multiple sensors. The technologies help pilots not only absorb information in real time, but react to that information appropriately -- something that could save pilot lives.
"So, he explained how the cockpit worked, how they used some of these techniques for optimizing input and output of data," Brethenoux said.
For a member of the audience from a large bank in Sweden, the presentation became an aha moment. To this bank employee, cockpits sounded a lot like trader workstations, which use similar technologies, like sensor fusion, and where information has to be absorbed and decisions made in rapid-fire fashion.
After a few months of discussion, the bank's trading desks were transformed to look and operate a little like the cockpits of fighter jets, showing how AI use cases can inspire new projects.
Know your business case
Brethenoux stressed that CIOs should first find a business problem before thinking about skills, data or technology. Starting with "a use case is not a good idea; it's a business case," he said during a phone interview. "So, start with a business case -- or two or three -- and scope them to the point where you can deliver things in weeks. That's where you start."
For a vodka producer Brethenoux consulted for, the business problem was maximizing sales during the Christmas season in the U.S. This was a couple of years ago, and Brethenoux said he started with machine learning to filter through data and find the best customer segments to go after -- those with the propensity to buy.
According to the data, the top customer segment turned out to be young males between the ages of 18 and 23. The problem was immediately evident: In the U.S., alcohol cannot be sold to anyone under the age of 21 -- in most places.
"And that's where this became complicated," Brethenoux said. "Every single county, every single city, every single state has its own rules and regulations on how to [market the product] and on what to authorize and not authorize." Brethenoux captured the regulations and implemented them in a rules-based system to comply with the laws enacted in every county, city and state.
Finally, Brethenoux and his team created a plan to distribute the product and used AI to optimize things like route and inventory management. "We needed to take into consideration many constraints and the predictive part of what we learned before to make sure we could have the inventory stacked in the right place at the right moment with the right flexibility," he said.
The value of external data
Brethenoux encouraged SMBs to count themselves in -- not out -- for AI use cases, even if they feel they don't have enough data to do so.
"The size of your enterprise, even the amount of data, is not really conducive to whether you should be using these techniques," Brethenoux said. In these cases, the data "has to be very high-quality data to be able to derive results," he said.
Or companies can offset internal data with third-party data. That's what a small chain of pastry shops in France did. The company had a hunch that weather patterns could impact what they were selling, dictating how they should display their goods. They proved out the hunch by taking historical sales data and combining it with localized third-party weather data they purchased to identify what items sold best during specific weather patterns and, consequently, what items should be placed prominently on top shelves and in storefronts.
It turned out that, when the weather's bad, glossier and heavier sugared pastries are in higher demand. "This project was a small project, but it was helpful for them," Brethenoux said.