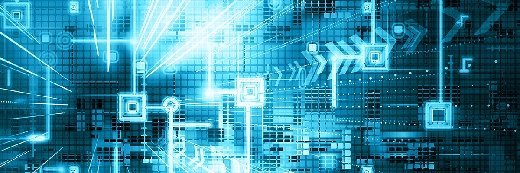
Petya Petrova - Fotolia
IT pros look to VMware's GPU acceleration projects to kick-start AI
The virtualization giant's recent acquisition of Bitfusion and its close partnership with Nvidia bring GPU virtualization to vSphere.
SAN FRANCISCO -- IT pros who need to support emerging AI and machine learning workloads see promise in a pair of developments VMware previewed this week to bolster support for GPU-accelerated computing in vSphere.
GPUs are uniquely suited to handle the massive processing demands of AI and machine learning workloads, and chipmakers like Nvidia Corp. are now developing and promoting GPUs specifically designed for this purpose.
A previous partnership with Nvidia introduced capabilities that allowed VMware customers to assign GPUs to VMs, but not more than one GPU per VM. The latest development, which Nvidia calls its Virtual Compute Server, allows customers to assign multiple virtual GPUs to a VM.
Nvidia's Virtual Compute Server also works with VMware's vMotion capability, allowing IT pros to live migrate a GPU-accelerated VM to another physical host. The companies have also extended this partnership to VMware Cloud on AWS, allowing customers to access Amazon Elastic Compute Cloud bare-metal instances with Nvidia T4 GPUs.
VMware gave the Nvidia partnership prime time this week at VMworld 2019, playing a prerecorded video of Nvidia CEO Jensen Huang talking up the companies' combined efforts during Monday's general session. However, another GPU acceleration project also caught the eye of some IT pros who came to learn more about VMware's recent acquisition of Bitfusion.io Inc.
VMware acquired Bitfusion earlier this year and announced its intent to integrate the startup's GPU virtualization capabilities into vSphere. Bitfusion's FlexDirect connects GPU-accelerated servers over the network and provides the ability to assign GPUs to workloads in real time. The company compares its GPU vitalization approach to network-attached storage because it disaggregates GPU resources and makes them accessible to any server on the network as a pool of resources.
The software's unique approach also allows customers to assign just portions of a GPU to different workloads. For example, an IT pro might assign 50% of a GPU's capacity to one VM and 50% to another VM. This approach can allow companies to more efficiently use its investments in expensive GPU hardware, company executives said. FlexDirect also offers extensions to support field-programmable gate arrays and application-specific integrated circuits.
"I was really happy to see they're doing this at the network level," said Kevin Wilcox, principal virtualization architect at Fiserv, a financial services company. "We've struggled with figuring out how to handle the power and cooling requirements for GPUs. This looks like it'll allow us to place our GPUs in a segmented section of our data center that can handle those power and cooling needs."
AI demand surging
Many companies are only beginning to research and invest in AI capabilities, but interest is growing rapidly, said Gartner analyst Chirag Dekate.
"By end of this year, we anticipate that one in two organizations will have some sort of AI initiative, either in the [proof-of-concept] stage or the deployed stage," Dekate said.
In many cases, IT operations professionals are being asked to move quickly on a variety of AI-focused projects, a trend echoed by multiple VMworld attendees this week.
"We're just starting with AI, and looking at GPUs as an accelerator," said Martin Lafontaine, a systems architect at Netgovern, a software company that helps customers comply with data locality compliance laws.
"When they get a subpoena and have to prove where [their data is located], our solution uses machine learning to find that data. We're starting to look at what we can do with GPUs," Lafontaine said.
Is GPU virtualization the answer?
Recent efforts to virtualize GPU resources could open the door to broader use of GPUs for AI workloads, but potential customers should pay close attention to benchmark testing, compared to bare-metal deployments, in the coming years, Gartner's Dekate said.
So far, he has not encountered a customer using these GPU virtualization tactics for deep learning workloads at scale. Today, most organizations still run these deep learning workloads on bare-metal hardware.
"The future of this technology that Bitfusion is bringing will be decided by the kind of overheads imposed on the workloads," Dekate said, referring to the additional compute cycles often required to implement a virtualization layer. "The deep learning workloads we have run into are extremely compute-bound and memory-intensive, and in our prior experience, what we've seen is that any kind of virtualization tends to impose overheads. ... If the overheads are within acceptable parameters, then this technology could very well be applied to AI."