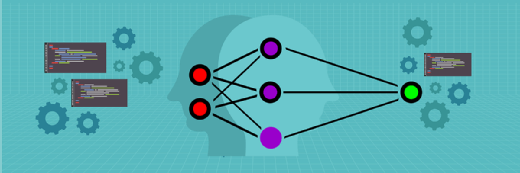
Evaluate open source vs. proprietary AIOps tools
Cut through the market hype surrounding AIOps to find the tool that suits your needs, comparing the pros and cons of open source vs. proprietary tools.
The debate surrounding open source vs. proprietary tools is nothing new. However, when it comes to AIOps tools there are some special considerations.
Not only is the AIOps tool market particularly complex, but the nature of AIOps tools -- which typically require access to sensitive data -- raises additional factors for buyers to weigh as they evaluate platforms.
Before we dig into the open source vs. proprietary debate, we must first define what makes a tool an AIOps tool. Then, to help buyers decide between open source or proprietary AIOps tools, we will compare the two and explain why an enterprise might prefer one type or another.
Defining AIOps
AIOps is a buzzword, and like most buzzwords, different people define it in different ways.
In this article, AIOps is defined as any type of IT tool or service that uses artificial intelligence (AI) or machine learning. This is a rather broad definition of AIOps; some vendors and analysts would contend that tools must use sophisticated AI and machine learning algorithms to qualify as AIOps.
However, given how rapid the AIOps market is growing, it can be helpful to think of AIOps in broad terms when evaluating the tools. Buyers might encounter some tools that perform AIOps functionality even if the tools aren't marketed explicitly as AIOps platforms. A narrow AIOps definition risks overlooking some of these options.
For a more comprehensive AIOps definition, watch this video.
Open source AIOps tools
To date, only a few open source projects label themselves as AIOps. Nonetheless, some open source platforms provide functionality that arguably qualifies as AIOps. For example, Kubernetes, which uses data analytics -- to a certain extent -- to automate workload orchestration, is partially an AIOps platform. Open source monitoring platforms, such as Nagios and Zabbix, also offer basic analytics functionality that you could consider to be AIOps, even if these tools don't define themselves as such. And a variety of open source programming language modules or frameworks, like PyTorch and TensorFlow, help implement AIOps functionality, even if they are not themselves complete AIOps platforms.
In many ways, the arguments in favor of open source AIOps tools are the same as those for any open source tool: Open source AIOps tools are generally less expensive and easier to modify or customize than proprietary alternatives. They also pose a lower risk of vendor and platform lock-in.
Beyond these factors, there are some special considerations to bear in mind when evaluating open source AIOps tools. One is that, so far, there are no end-to-end open source AIOps platforms. In other words, there is no single open source platform that offers all the AIOps functionality that a business would want to streamline its IT operations. Instead, there is a fragmented collection of different open source tools that each provide some AIOps functionality. To use these open source tools and take advantage of AIOps benefits, IT operations teams will need to integrate multiple tools. But that requires significant effort.
On the other hand, by their nature, AIOps tools require access to reams of data, some of which is potentially sensitive to a business, or which attackers could abuse to launch breaches. This means that, with a proprietary AIOps tool, buyers must trust the vendor to be a good steward of the data its tool ingests from their customer's systems and environments. There might also be compliance issues to consider, especially if the vendor's tool moves a user's data to the vendor's own infrastructure for processing or storage.
These concerns might affect open source AIOps tools, too, if the platforms require data processing on external infrastructure. But given that open source tools typically run within a user's data center -- or at least on public cloud infrastructure that the user controls -- there are likely to be fewer compliance or data privacy challenges. And the fact that anyone can inspect the source code of open source tools to determine exactly what they do with users' information adds further transparency and trust to data management processes.
Proprietary AIOps tools
In contrast to the open source space, the proprietary software market has produced a large and growing number of tools explicitly marketed as AIOps. For example, Broadcom Inc. heavily markets its observability software as AIOps. Splunk is also getting into AIOps, as well as smaller vendors like Instana and PagerDuty. At this point, more proprietary monitoring or incident response tools label themselves as AIOps -- at least partially -- than not.
Perhaps the most important reason to use a proprietary AIOps tool is that it's likely to be easier to implement than an open source alternative. Proprietary tools are generally more user-friendly and they tend to have broader AIOps functionality than open source options -- which, as noted above, offer only fragmented and partial AIOps features. Plus, many proprietary AIOps tools run as managed services, which eliminates the need for users to set up infrastructure to host them.
The data stewardship issues described above might be a challenge when working with some proprietary AIOps tools. However, if the AIOps vendor delivers the compliance guarantees and level of trust necessary to mitigate concerns about misuse of ingested data, these challenges are unlikely to be deal-breakers for the average enterprise. Most vendors in the AIOps space are well-established companies with a long history of experience managing customer data in a compliant and secure way.
The future of open source and proprietary AIOps
A final factor when evaluating AIOps tools is the rapid rate of the market evolution. While the open source ecosystem lags behind the proprietary software market in AIOps offerings as of early 2021, that might change as more open source developers and funders devote their resources to AIOps tooling.
It's also likely that proprietary vendors will continue to build out AIOps features. Today, most commercial AIOps tools fall into the category of monitoring and incident management solutions, but it's likely more proprietary AIOps tools will emerge in categories such as log management, infrastructure provisioning and other IT operations niches in the near future.
Evaluating AIOps tools requires patience and an attention to detail. Enterprises will also likely want to revisit their AIOps tool selections on a regular basis to determine whether better options have emerged.