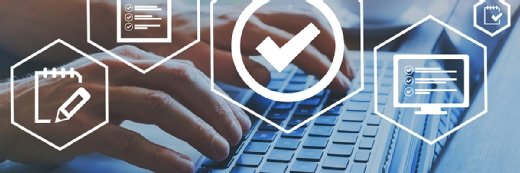
Getty Images/iStockphoto
Compare proprietary vs. open source for enterprise AI
Unsure whether to choose proprietary or open source AI for your enterprise deployment? Compare the pros and cons of both software models, including how each can benefit businesses.
When selecting enterprise AI software, one of the first decisions to make is whether to choose proprietary or open source.
In many respects, this choice involves the same considerations that typically shape decisions around open source vs. proprietary, also known as closed source, software. But for AI tools, there are special considerations to weigh, including the following:
- Intended use case.
- Customization needs.
- Infrastructure requirements.
- Cost models.
- Organization-specific characteristics.
Some organizations will find that either proprietary or open source AI best fits their specific requirements, while others might choose a mixture of both. By analyzing each software model's pros and cons, organizations can identify the best approach for their needs.
The differences between proprietary and open source AI
The term proprietary AI refers to AI platforms, tools or services whose source code is not publicly available, including popular chatbots from OpenAI and Anthropic. The source code of open source AI, in contrast, is freely available for anyone to download, inspect and -- in most cases -- reuse. Although open source AI isn't necessarily free of cost, most open source AI tools, such as Meta's Llama models and Mistral's Le Chat, can be freely used without paying licensing fees.
Much of the current conversation surrounding proprietary vs. open source AI focuses on generative AI, both due to broader hype around the technology and because several notable open source large language models have appeared over the past year or so. Many of these LLMs are positioned as alternatives to OpenAI's ChatGPT and other proprietary, vendor-developed LLMs. Consequently, they've gotten people talking about whether open source can become a real alternative to proprietary AI tools.
Importantly, even open source generative AI software often provides the public with access to models' source code, but not their underlying training data. Because no other type of software depends on training data in quite the same way generative AI does, this limits the flexibility of open source AI relative to other types of open source software. That said, this limitation might not matter for enterprises that intend to train open source AI models using their own data or use an open source model as is, regardless of how it was trained.
Enterprise AI also isn't limited to the generative AI realm. Proprietary and open source offerings exist across all major parts of the AI ecosystem:
- Descriptive analytics. Open source tools like Apache Spark are useful for analyzing large volumes of data for descriptive analytics purposes, as are proprietary options from vendors such as Splunk and SAS.
- Predictive analytics. Open source tools like H20 and Knime can help businesses implement predictive analytics. They exist alongside a large collection of proprietary predictive analytics offerings from vendors like IBM and Microsoft.
- Generative AI. Open source LLMs, which can be used to build generative AI software, are available from companies including Mistral, Meta and Google. A number of vendors also offer proprietary LLMs and generative AI services built on top of them.
No matter what your enterprise wants to do with AI, you can likely find both proprietary and open source software to help do it.
Comparing proprietary vs. open source AI
While proprietary and open source AI tools often offer the same core capabilities and features, they differ in other critical ways.
Customizability
Because anyone can view and modify the source code for open source AI software, these tools are easier to customize. Proprietary enterprise AI options typically support some customization options, but they don't provide the unlimited customizability that comes with having access to source code.
In addition, as noted above, many open source generative AI tools offer access to source code, but not training data. Without that data, AI models are more difficult to understand and customize. In this sense, open source AI tools could be less customizable than their open source label would otherwise imply.
Ease of use
In general, open source AI software requires more effort and expertise to set up and use compared with its proprietary counterparts. You'll need engineers who are adept at working with your AI use case -- whether descriptive analytics, predictive analytics or generative AI -- and they'll need to be ready to customize and troubleshoot the software.
In contrast, most proprietary AI tools require limited expertise for enterprise use. In fact, vendors like Microsoft and Google are increasingly integrating AI features into software that enterprises already use, meaning enterprises can use proprietary AI without much effort at all. When custom application development is required -- for instance, when building applications that connect to OpenAI's APIs -- the complexity of using proprietary AI services is typically much less than deploying and integrating open source AI from the ground up.
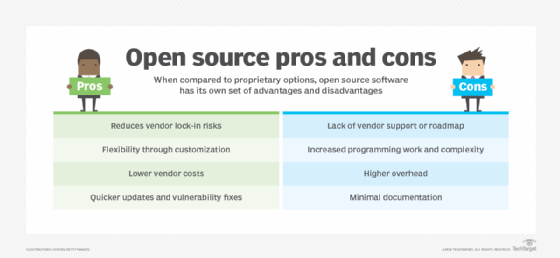
Infrastructure requirements
Many proprietary AI platforms are available as fully managed offerings -- meaning enterprises don't need to set them up on their own infrastructure -- and are powered by pretrained AI models. Consequently, they don't require the acquisition of new hardware.
This is often not the case when working with open source AI software. Such software might require enterprises to purchase massive storage resources to house the data that their software analyzes or trains on. They might also need access to specialized servers, such as ones equipped with GPUs, to perform model training if they want to train or customize models.
Cost models
It's impossible to categorically say whether proprietary AI costs more than open source AI or vice versa; the costs of each can vary depending on factors like an enterprise's applications and frequency of use. However, the underlying cost models for each approach are different.
Open source AI software tends to have low upfront costs because there are usually no licensing fees to pay. However, implementation and ongoing support costs could be higher due to the staffing and expertise necessary to deploy and administer open source AI.
Proprietary AI can also demand a fair amount of employees' time to set up and maintain, especially if the AI software requires bespoke configurations or integrations. However, the ongoing administration costs of proprietary AI are usually lower. The main cost is the upfront fees that companies pay to access proprietary AI tools or services.
Proprietary and open source AI: Better together
There's no reason to go all in on proprietary AI or all in on open source AI. Organizations can -- and, in many cases, should -- use both at the same time.
For instance, a business could use proprietary AI services built in to software that its employees already use to enhance employee productivity without having to implement complex open source AI software. At the same time, its developers could use an open source LLM to build a custom application that generates content unique to the business.
In short, the future of AI at most enterprises is likely to include both proprietary and open source software. What will vary from one company to the next is the ratio of proprietary vs. open source AI software.
Factors to consider when choosing between open source vs. proprietary AI
There are no simple rules for determining whether proprietary or open source AI is a better fit for your organization's needs. Most organizations will want to weigh several factors:
- In-house AI expertise. The more AI experience your engineers have, the better equipped you'll be to implement open source AI.
- AI use cases. For simple AI use cases, such as increasing employee productivity by using generative AI to draft contracts or documentation, proprietary AI often suffices. If you have complex or unique use cases, however, you might want to build your own tool using open source AI as a foundation.
- Access to training data. As noted above, you might need to train or customize models when using open source generative AI, which requires training data. If you don't have that data, or if you lack the infrastructure necessary to train models, you might be better served by proprietary software.
- Governance and compliance needs. If you are required to implement certain security, privacy or controls for AI technology due to governance or compliance mandates, you'll want to make sure that any proprietary AI you choose can support the configuration you need. Open source options are more flexible in this regard because you can customize them.
- Long-term goals. If you plan to use an AI tool for a long time -- say, at least a decade -- it arguably makes more sense to choose open source AI, since open source projects are less likely to close or be disrupted through mergers or acquisitions. Proprietary AI tools are more likely to change; for example, it seems unlikely that OpenAI, a fast-changing startup, will continue to offer the same services 10 years from now that it does today, if the company still exists at all.
Chris Tozzi is a freelance writer, research adviser, and professor of IT and society who has previously worked as a journalist and Linux systems administrator.