PyTorch
What is PyTorch?
PyTorch is an open source machine learning (ML) framework based on the Python programming language and the Torch library. Torch is an open source ML library used for creating deep neural networks and is written in the Lua scripting language. It's one of the preferred platforms for deep learning research. The framework is built to speed up the process between research prototyping and deployment.
The PyTorch framework supports over 200 different mathematical operations. PyTorch's popularity continues to rise, as it simplifies the creation of artificial neural network models. PyTorch is mainly used by data scientists for research and artificial intelligence (AI) applications. PyTorch is released under a modified BSD license.
PyTorch was initially an internship project for Adam Paszke, who at the time was a student of Soumith Chintala, one of the developers of Torch. Paszke and several others worked with developers from different universities and companies to test PyTorch. Chintala currently works as a researcher at Meta -- formerly Facebook -- which uses PyTorch as its underlying platform for driving all AI workloads.
How does PyTorch work?
PyTorch is pythonic in nature, which means it follows the coding style that uses Python's unique features to write readable code. Python is also popular for its use of dynamic computation graphs. It enables developers, scientists and neural network debuggers to run and test a portion of code in real time instead of waiting for the entire program to be written.
PyTorch provides the following key features:
- Tensor computation. Similar to NumPy array -- an open source library of Python that adds support for large, multidimensional arrays -- tensors are generic n-dimensional arrays used for arbitrary numeric computation and are accelerated by graphics processing units. These multidimensional structures can be operated on and manipulated with application program interfaces (APIs).
- TorchScript. This is the production environment of PyTorch that enables users to seamlessly transition between modes. TorchScript optimizes functionality, speed, ease of use and flexibility.
- Dynamic graph computation. This feature lets users change network behavior on the fly, rather than waiting for all the code to be executed.
- Automatic differentiation. This technique is used for creating and training neural networks. It numerically computes the derivative of a function by making backward passes in neural networks.
- Python support. Because PyTorch is based on Python, it can be used with popular libraries and packages such as NumPy, SciPy, Numba and Cynthon.
- Variable. The variable is enclosed outside the tensor to hold the gradient. It represents a node in a computational graph.
- Parameter. Parameters are wrapped around a variable. They're used when a parameter needs to be used as a tensor, which isn't possible when using a variable.
- Module. Modules represent neural networks and are the building blocks of stateful computation. A module can contain other modules and parameters.
- Functions. These are the relationships between two variables. Functions don't have memory to store any state or buffer and have no memory of their own.
PyTorch benefits
Using PyTorch can provide the following benefits:
- Offers developers an easy-to-learn, simple-to-code structure that's based on Python.
- Enables easy debugging with popular Python tools.
- Offers scalability and is well-supported on major cloud platforms.
- Provides a small community focused on open source.
- Exports learning models to the Open Neural Network Exchange (ONNX) standard format.
- Offers a user-friendly interface.
- Provides a C++ front-end interface option.
- Includes a rich set of powerful APIs that extend the PyTorch library.
PyTorch vs. TensorFlow
PyTorch is often compared to TensorFlow, a deep machine learning framework developed by Google. Because TensorFlow has been around longer, it has a larger community of developers and more documentation.
However, PyTorch does have advantages over TensorFlow. PyTorch dynamically defines computational graphs, unlike the static approach of TensorFlow. Dynamic graphs can be manipulated in real time. Additionally, TensorFlow has a steeper learning curve, as PyTorch is based on intuitive Python.
TensorFlow may be better suited for projects that require production models and scalability, as it was created with the intention of being production ready. However, PyTorch is easier and lighter to work with, making it a good option for creating prototypes quickly and conducting research.
Top PyTorch use cases
PyTorch is one of the most popular deep learning frameworks due to its flexibility and computation power. It's easy to learn and is used in many applications, including natural language processing (NLP) and image classification.
The following are a few common use cases of PyTorch:
NLP. NLP is a behavioral technology that enables a computer to understand human language as it's spoken or written. Main elements of NLP include machine translation, information retrieval, sentiment analysis, information extraction and question answering.
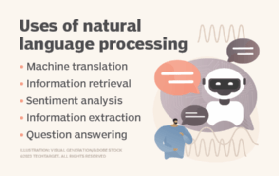
Deep neural networks are behind several breakthroughs in machine understanding of natural languages such as Siri and Google Translate. But most of these models employ a recurrent neural network method to treat language as a flat sequence of words, whereas many linguists support the recursive neural network model, as they believe that language is best understood when presented in a hierarchical tree of phrases. PyTorch makes these complicated language models easier to understand. For example, in 2018, Salesforce developed a multi-task NLP learning model that performs 10 tasks at once.
Reinforcement learning. The Python library known as Pyqlearning is used for executing reinforcement learning (RL), which is a subset of ML. In RL, a machine is made to learn from experience so that it can take proper decisions to get the best reward possible. RL is mainly used for developing robotics for automation, robot motion control or business strategy planning, and employs the Python Deep Q learning architecture for building a model.
Image classification. This process classifies an image based on its visual content by using an image classification algorithm. For example, the algorithm can tell a computer vision application whether a certain image contains a cat or a dog. While object detection is effortless for the human eye, it can be challenging for computer vision applications. By using PyTorch, a developer can process images and videos to create an accurate computer vision model.
The PyTorch framework plays an important role in the world of NLP. Here are five reasons why chatbots need NLP and how sophisticated NLP, including intent and sentiment analysis, can improve their performance.