- Share this item with your network:
- Download
Network Evolution
- FeatureNetwork analytics tools deepen with machine learning and AI
- FeatureNetworking elements of a digital transformation framework
- FeatureEnterprise network management in the wake of vendor consolidation
- OpinionNetwork data analytics must work alongside human ingenuity
- FeatureWireless networking technology helps craft IT modernization
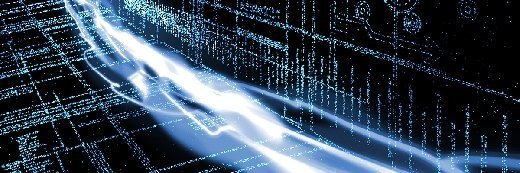
Kit Wai Chan - Fotolia
Network analytics tools deepen with machine learning and AI
Network analytics tools that utilize machine learning and AI can help IT pros with monitoring and performance evaluation, and provide business insight. But a holistic view of the network using analytics is still on the horizon.
David Morton, director of networks and telecommunications for the University of Washington, manages a wireless network for three college campuses, three hospital sites and 30 additional clinics; several research locations; and up to 85,000 users connecting up to 200,000 devices.
If anyone asked, he could pinpoint a single device and single user and know whether that person was standing inside a building or was walking outside on the way to class. What provides Morton such deep visibility are network analytics tools driven by machine learning and artificial intelligence principles.
Users of the university's network expect it to always work, no matter how much usage grows, Morton said. Applying new network analytics tools provides a much better look into network performance and helps the university keep up with demands.
Aruba's NetInsight highlights network performance issues
The level of granularity available from the network analytics tools it uses helps the IT team to monitor and provide insights to how users interact with the wireless network and how the team can make improvements to that experience, he noted.
The University of Washington created its wireless network using 12,000 access points and about 25 controllers from Aruba Networks. It also uses Aruba's NetInsight analytics software to collect and analyze data streaming from those access points and controllers. NetInsight applies algorithms built with a matrix of variables relevant to the devices and applications being analyzed and monitored, Morton said. The results are displayed in a dashboard that allows the IT team to drill down to individual users and network elements.
According to Morton, the analysis has helped IT identify problems that are too difficult to find on their own or take too long to find manually. For example, in one classroom of 450, students were asked to use clicking devices to enter responses in an interactive test. Of those students, 50 found that their clickers were not working. NetInsight automatically used an algorithm to find the problem and suggest how to fix it.
In a separate case, he added, the university discovered 361 access points were malfunctioning. Instead of IT staff having to dig through all the access points manually, the network analytics tool was able to provide a list of the malfunctioning sites and what was wrong with them.
Machine learning, AI and advanced network analytics
Eventually, the university hopes that NetInsight will be able to proactively solve issues that arise, Morton said, adding the environment for machine learning and AI is changing so rapidly that there's a learning curve to discover what analytics tools will be able to do someday.
IT managers can tap into more data generated by the number of devices proliferating across their enterprise networks. Data from those devices and other network sources can provide insight into how networks are performing. How that data is collected and analyzed to provide meaningful, actionable information is the key to making a difference in network operations. As machine learning and AI continue to mature, IT pros are turning to a new crop of network analytics tools to help them monitor and evaluate network performance and even provide insight to the business. Still, it will likely be several years before these tools are comprehensive enough to deliver a complete and holistic picture of the entire network -- from the branch office to the cloud.
The challenge of analyzing massive amounts of network data in real time is creating increased demand for advanced network analytics tools, particularly as more organizations use cloud services and place higher value on security needs in the wake of greater distributed denial-of-service attacks, according to Market Research Future in Boston. The technology research and consulting firm forecasts that global network analytics spending will increase from $2.54 billion in 2016 to $3.1 billion by 2022.
Perhaps no of networking better reflects the value of analytics than wireless technologies, where organizations have been able to improve their Wi-Fi and wireless LAN performance with the aid of network analytics tools that increasingly harness both machine learning and AI.
Vendors are trying to tap into this demand. In addition to Aruba's focus on analytics, Mist Systems has a wireless analytics service that blends AI and cloud-based machine learning to provide enterprises with information to monitor the performance of their wireless LANs. ExtraHop Networks offers Addy, its machine learning-as-a-service product, and recently cybersecurity analytics software to its IT performance monitoring platform. Nyansa's product, Voyance, is a campus and enterprise-grade network monitoring system that lets users diagnose performance issues across both wired and wireless networks.
Network analytics demand on the rise
The benefits of deeper levels of insight go beyond the network, however. Enterprise network managers have begun to see a corporate culture shift and the need to be more integrated with the business. Enhanced analytics helps provide this boost, according to Sanjit Ganguli, research vice president at Gartner.
For example, if a company has noticed a decline in sales for one of its online products, the network team can use analytics tools and possibly correlate the decline with a spike in latency at a particular time, he said. That gives the company meaningful information to act upon. "[With] every packet that goes over the network, there's a tremendous amount of application user information that can be garnered," Ganguli said. "Networks can be used even further to provide business insight that other teams might not have through any other mechanism."
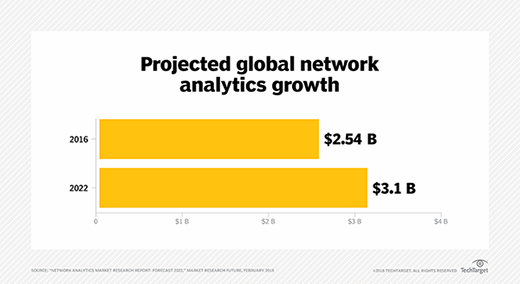
For Schlegel Villages, a company with 19 long-term care and retirement communities across Ont., Canada, being able to see deeply into network performance can be a matter of life or death, according to Chris Carde, director of IT. The healthcare company has rolled out an upgraded wireless network and is deploying new clinical applications that will allow its 4,800 employees to use mobile devices at the point of care to update medical records while interacting with residents.
Schlegel installed Aruba access points and controllers for the network and expects to complete its deployment of the PointClickCare electronic health record by mid-2018. By the time it's finished, all Schlegel healthcare teams will be able to use iPads and other Apple devices to access medical records at the point of care and update and share information in real time, Carde said.
At this point, Schlegel is conducting basic wireless management using Aruba products. Carde said he sees the company needing to move to analytics tools like NetInsight eventually. Having a fast, reliable wireless network is critical to providing high quality of care, and a deeper level of insight will help the IT staff know when and where network problems appear, he said.
Network transformation, machine learning and AI
The ability to have that deeper level of insight is part of the transformation both wired and wireless networks are going through, Ganguli said. In the past, organizations viewed their networks more like a utility. Now, increasingly the network "is a strategic part of overall business goals."
That's happening because business itself is undergoing a transformation, Ganguli added. "There is this massive shift toward digital business," with enterprises heavily relying on their applications to maintain and grow revenue, he said. Network managers have an opportunity to demonstrate to the business how important the network is by investing in analytics tools that offer enhanced performance and disaster recovery options. Machine learning and AI are steps in that direction, he said.
Machine learning is based on algorithms that allow computers to learn to perform functions like detecting anomalies on the network, correlating baseline performance and matching patterns of behavior. Vendors are investing on developing these features now, Ganguli said.
AI is more of an aspirational goal that takes machine learning to the next level by coupling a deeper understanding of network data with automated actions, he added.
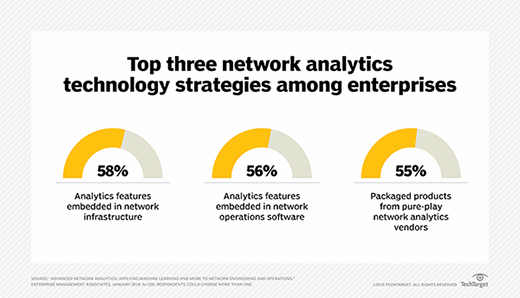
Even as analytics tools become more comprehensive, using them to create a cohesive, extensive picture of the entire network is still several years away, according to Andre Kindness, a principal analyst with Forrester Research.
Network monitoring vs. network analytics
Today, Kindness said network managers are primarily involved with monitoring network performance and activity. Where monitoring involves collecting enough data to look retroactively at the network, analytics takes that historical data and uses it to model the entire network. The trick is translating all of that information into a tool that can understand both what's occurred on the networks as well as proactively take steps to diagnose and alert IT managers to potential problems. That's much more complicated.
SaaS-based and cloud-based monitoring tools are now available that can help enterprises with the part, Kindness said. As software-defined networking and intent-based networking -- two areas in which analytics will be relied upon to provide that comprehensive view of the network -- are implemented, analytics tools will improve, he added.
Vendors and enterprises have to improve network analytics tools, Ganguli said. Most network managers already use tools for network infrastructure monitoring, trying to understand how routers and switches are behaving, Ganguli said.
Tapping into additional sources like packet data, and pulling out more comprehensive diagnostics data through the use of machine learning and AI will add a level of sophistication to analyzing networks that wasn't possible before. "Network performance monitoring tools can get into not only the health of the infrastructure," Ganguli said, "but who's consuming bandwidth, what type of applications, latencies on the network, end-user response time, breakdowns of delays and ultimately business intelligence. ... There's a much deeper view."
The collection and use of data to monitor and analyze network performance is increasing as networks grow and more devices are connected to them. But network teams are only at the forefront of creating meaningful information from that data through sophisticated network analytics tools like machine learning and AI. As those tools develop further, analytics will have a greater role to play in enhancing network performance.
Dig Deeper on Network management and monitoring
-
Should IT leaders plan for the quantum computing era?
-
How PAs, APPs Expand Patient Care Access, Patient Satisfaction
-
How Aberdeenshire Council is using Cherwell’s ITSM platform
-
Aruba Central touches more devices, gets better analytics