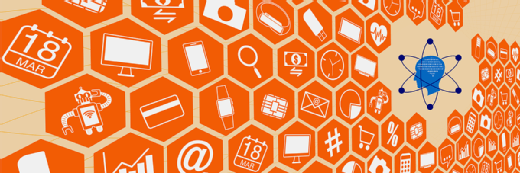
On-device AI features make connected tools smarter
As the number of connected devices grows, AI at the edge of networks is increasingly making connected devices smarter, enabling them to take advantage of emerging smart technologies.
According to research firm IHS Markit, there are more connected IoT devices than there are humans on the planet -- with nearly 27 billion such connected devices in 2017, and the likelihood of over 125 billion by 2030. These devices are doing everything from providing second-by-second performance data on aircraft engines to powering connected refrigerators.
IoT devices have powered many applications that continue to show the benefits in a wide range of industries. On-device AI is poised to deliver even more power and capabilities to these edge devices.
AI makes automation more intelligent
The use of machine learning, computer vision and natural language capabilities is enabling a greater number and variety of tasks that can be automated through increasingly smarter devices. Machine learning provides double opportunities for IoT devices: increased intelligence of the devices themselves with on-board AI capabilities and greater intelligence for the systems that monitor and manage distributed IoT devices.
With thousands, if not millions of devices in operation and constant communication, enterprises are being challenged to make sure these devices continue to work as intended and provide value as expected. AI-enabled monitoring devices can keep an eye on these systems to spot anomalies and patterns in data that suggest behavior outside of the acceptable norms.
These AI-enhanced IoT monitoring dashboards can also help spot trends that suggest potential problems for either the IoT device itself or the systems it is monitoring. In this way, companies can keep tabs on devices without having to be constantly looking at reports or waiting for failures.
Similarly, IoT tools can be powered with on-device AI to offer more intelligence and to operate autonomously. Machine learning models can be loaded on IoT devices and detect when trained activities happen, operating with little or no central control over specific activities. For example, sensors with anomaly detection systems can automatically shut down devices or systems when unusual conditions occur.
These devices can also make limited decisions when disconnected from the internet or in sometimes-connected situations, such as in remote oil fields, in the middle of the ocean or air, or on another planet, such as the new AI-enabled IoT enhancements NASA is adding to future rovers.
Taking things a step further, AI can also empower devices with vision and natural language processing power at the edge. Surveillance cameras are increasingly gaining deep learning-powered computer vision capabilities that enable them to not only detect motion, but to detect specific objects, identify people or animals, read license plates, and track unidentified or unrecognized objects across frames of video.
Similarly, devices from microwave ovens to automobiles are gaining the power to understand natural language and respond to voice commands without requiring any tactile input or feedback from users. Perhaps in the not-too-distant future, devices will be produced without any screens or buttons at all, counting on the fact that users will expect to talk to them.
Personalized device interaction
AI and machine learning are also empowering edge devices with more information than was previously available. Rather than simply configuring default or basic settings, many IoT devices are learning the behavior of users and adapting to their personalities and lifestyles. Turning on the light used to be as simple as flicking a switch, but now we have on-device AI that knows when you're coming home, what mood you're in, and even if you are alone or have guests.
This hyper-personalization enabled by machine learning is finding its way into a wide range of devices, from personal technology to storefront and retail uses that observe traffic and customer behavior and change their operation as a result. Fujitsu is piloting the use of AI-enabled wearable devices to determine if factory workers have potentially threatening heat stress over time.
AI systems are also applying learned behavior to help predict and prevent machine breakdowns, leaks, or other patterns that could lead to unexpected downtime or loss.
IoT devices used in especially mission-critical scenarios, such as in trains and mining operations, are keeping an eye on the staff operating those machines and acting preemptively when a disabled or distracted operator could potentially cause significant damage. The systems learn what normal operations look like and can then easily detect anomalous operations.
Caterpillar uses IoT and machine learning to uncover patterns in equipment and device data to make sure they continue to operate within design parameters. Rolls-Royce has AI-enabled IoT devices within their jet engines that use machine learning to help spot patterns and identify operational insights.
Even with more mundane use cases, AI is showing great value in IoT devices. Hershey has reported using AI to significantly reduce weight variability during production, and various shipping companies are using AI-enabled IoT devices to increase overall efficiency with things like ship cleaning and weight distribution.
AI improves security threat prevention
With all these connected devices in existence, many of which control or monitor critical infrastructure, it's no surprise that they are attractive targets for criminals, shadow government operators, and those out to cause trouble and mayhem. Indeed, to many in the cybersecurity field, IoT should be called the vulnerability of things instead.
In 2016, the well-publicized Mirai attack turned millions of IP cameras, home routers and other Linux-enabled IoT edge devices into remote-controlled bots that were used in the large-scale botnet attack. This opened many people's eyes to the fact that these devices are sitting unprotected on networks with little visibility into how much of a threat they can actually pose if compromised.
As such, security experts are attempting to leverage the power of AI to detect and disrupt attempts to compromise or otherwise interfere with the operation of IoT devices and the systems they control. Machine learning-enabled IoT devices are able to detect access and usage patterns that are outside the acceptable norms. They can then either flag the activity for further follow-up by human operators, proactively thwart the attack or even shut down as needed.
The correct behavior obviously depends on the use case, but it's clear that on-device AI can offer a more intelligent layer of protection that can automatically separate legitimate uses from those with malicious intent.
Making the edge more intelligent
The days of unintelligent IoT devices are numbered. There are simply too many valuable use cases for making these devices more aware of their environment, more responsive to continuously changing feedback, enhanced with vision and speech capabilities, and able to detect and prevent unauthorized usage. Indeed, organizations now deploying IoT devices on their networks or in their customers' homes or organizations should make sure they have machine learning-enhanced capabilities to deal with the reality of the AI-enabled future.