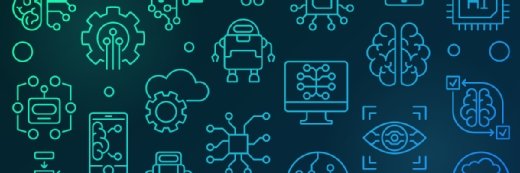
AI-focused storage choices, features and considerations
The list of GenAI-focused storage options grows as Pure, Dell, HPE and other major vendors innovate to win over IT infrastructure buyers.
The rise of generative AI workloads has placed significant pressure on data center storage infrastructure, with AI workloads requiring substantial compute power, data throughput and storage capacity to train models and perform inference tasks.
With that, most major storage vendors have redesigned their systems to support massive AI workloads, many through partnerships with Nvidia. Some have also baked generative AI (GenAI) right into their infrastructure to automate IT admin tasks, such as storage management.
The major cloud storage platforms, including AWS S3, Google Cloud Storage and Microsoft Azure, offer a range of storage services optimized for AI, including object storage, block storage and file storage.
In addition, specialized storage vendors have launched storage systems tailored for AI workloads. These vendors offer features such as high-performance all-flash arrays, object storage and cloud-integrated infrastructure to address the unique requirements of GenAI applications.
Storage products for AI
At Pure Accelerate 2024 this month, Pure Storage launched an AI copilot to help IT admins manage fleets of storage using natural language. The copilot uses data insights from Pure customers to help investigate issues and proactively protect data, according to the company. Similarly, Dell delivered a generative AI assistant for Apex in May that provides a natural language interface to address infrastructure questions.
Pure, which was early in delivering AI-focused infrastructure with the AIRI system back in 2018, also launched a new storage-as-a-service system for AI this month. Evergreen One for AI provides "guaranteed storage performance for GPUs to support training, inference, and HPC workloads," according to the company.
In addition to Pure, some examples of other storage systems that support GenAI include the following:
- Dell AI Factory, a portfolio of hardware, software and services to support AI, includes a new PowerScale scale-out file system used for unstructured data and training.
- Hitachi Vantara's Hitachi iQ offers industry-specific AI systems that use Nvidia DGX and HGX GPUs alongside the company's storage systems.
- HPE upgraded its Alletra MP storage arrays to support increased server connectivity and capacity, while integrating Nvidia's NIM microservices into its GenAI supercomputing and enterprise systems.
- IBM Spectrum Storage for AI, integrated with Nvidia DGX, provides a converged, scalable system that includes compute, storage and networking tailored for AI workloads.
- NetApp now offers product integrations with Nvidia's BasePod and SuperPod, as well as integration of Nvidia's NeMo Retriever microservices into its OnTap hybrid cloud storage.
- Vast Data launched its Vast Data Platform in 2023, which marries its QLC flash-and-fast-cache storage subsystems with database-like capabilities at the native storage IO level and DGX certification.
- Weka, a hybrid cloud NAS provider, delivered a hardware appliance certified to work with Nvidia's DGX SuperPod AI infrastructure.
- Western Digital recently launched new high-performance SSDs and high-capacity HDDs for AI workloads.
Feature considerations
When evaluating infrastructure vendors to support AI initiatives, look for the following features.
Scalable and flexible compute power. A storage system for AI workloads should provide scalable and flexible compute resources, including the use of GPUs and tensor processing units, to support the training and execution of complex AI models.
The infrastructure should also be able to dynamically scale up or down based on the workload demands, ensuring efficient resource utilization.
High-bandwidth networking. AI-powered storage should provide low-latency and high-bandwidth networking to support transfer of large data sets and connectivity of compute resources. The networking infrastructure should also be optimized to mitigate potential bottlenecks and ensure low inference times for AI-driven applications.
Intelligent data management. The generative AI assistant within storage products should be capable of automatically managing and configuring the storage infrastructure, including optimizing workload placement, predicting and preventing system failures, and proactively planning for resource and capacity needs.
AI-powered data management capabilities should also include intelligent data classification, policy-driven data protection tasks and enhanced security measures to safeguard the organization's data.
Generative AI integration. The system should seamlessly integrate GenAI and large language models to improve data operations across the entire data pipeline.
The AI capabilities should enable automated data observability, proactive issue identification and resolution, and the generation of reports and visualizations to enhance data team productivity.
Preparing for AI storage
Adopting storage for GenAI workloads presents several key challenges and risks that IT pros must prepare for to ensure a successful implementation.
Data volume. Generative AI models consume and generate vast amounts of data, often in real time. The storage infrastructure must be capable of handling the high volume and velocity of data. Inadequate storage capacity and performance could lead to bottlenecks, impacting model training and inference times.
Capacity planning. Evaluate types of storage that offer scalable, high-performance capabilities, such as all-flash arrays, object storage and distributed file systems. Conduct thorough capacity planning and stress testing to ensure the storage can accommodate current and future data growth.
Data security and governance. Sensitive data used for training GenAI models must be secured and governed. Improper data handling could lead to data breaches, compliance violations and reputational damage. It's important to implement robust data security measures, including encryption, access controls and data lineage tracking. Also, ensure the storage environment aligns with your organization's data governance policies and regulatory requirements.
Infrastructure complexity. Integrating the storage infrastructure with the broader AI ecosystem, including compute, networking and software components, can be complicated. A modular, open architecture approach enables seamless integration with various AI frameworks and tools.
Vendor lock-in. Selecting a storage system that is tightly coupled with a specific AI platform or cloud provider can limit flexibility and increase the risk of vendor lock-in. Storage providers that offer vendor-agnostic compatibility, enabling the user to mix and match components from different providers, are an option to prevent this issue.
Bridget Botelho, editorial director, news, compiled this report.