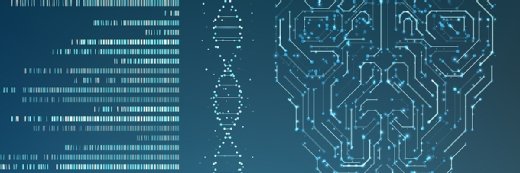
Nobi_Prizue/istock via Getty Ima
AI and the future of genomics
AI genomics' speed and accuracy in drug target discovery, disease modeling and detection, and gene therapy hold promise for delivering personalized, precise medicine to patients.
AI and genomics are undergoing rapid periods of innovation and change. Recent AI advancements in computing, management techniques, algorithms and multimodal large language models are enhancing genomic research.
Genomics is also getting a boost from better tools for sequencing DNA, connecting these depictions to insights into dark DNA, determining the impacts of genomic expression at different levels of abstraction, exploring the expression of genes across organs and tissues using spatial biology, and improving gene editing techniques that alter DNA sequences within a genome.
But the current state of genomics and AI is a mixed bag. Two decades after the first human genome was sequenced, DNA data has been reserved for understanding rare diseases, treating cancer and general research. While using DNA data for personalized medicine shows promise, it hasn't achieved mainstream adoption. And although gene editing appears to be an exciting application, the practice isn't widely used for treating common medical conditions. Still, industry observers see much potential for AI and genomics.
"AI is extensively used in genomics to expand its application potential and increase the speed and accuracy at which vast amounts of genomic data are analyzed," said Neeraja V, senior analyst at the consultancy Everest Group. AI has demonstrated the ability to analyze and identify genetic variations and detect patterns and protein structures, she added. AI also enables multiomic data analysis beyond genomics for integrated metabolomic, proteomic, transcriptomic and epigenomic analysis.
How AI and genomics are used today
Researchers and healthcare practitioners still struggle to understand how genomics data can translate into improving health outcomes. Yet there are tangible applications for AI in genomics, according to Neeraja V, including drug target discovery, disease modeling, disease detection, biomarker identification, host-pathogen interactions and protein structure prediction.
More specifically, Erik Pupo, director of the commercial health IT advisory at consulting firm Guidehouse, pointed to some of the more significant use cases of AI in genomics:
- Clinical genomics. AI-backed tools from health technology company Tempus interpret genetic data for rare diseases and cancer diagnostics, matching patients with the most effective treatments. Clinical genomics is the most common application of AI in genomic healthcare.
- Variant calling and interpretation. Researchers use Google DeepVariant to identify and interpret genetic variants with unprecedented accuracy. These tools have the capability to outperform traditional methods, significantly improving clinical diagnostics and personalized care outcomes.
- Protein structure prediction. Google's DeepMind AlphaFold can predict protein structures to facilitate faster drug discovery and greater insights into genetic diseases.
- Genome annotation. AI is used to identify functional genomic elements, such as regulatory regions and non-coding RNA molecules. Precision medicine software provider GenomOncology is just one company developing tools to streamline research and clinical genomics annotation. The pace of annotation is rapidly increasing due to AI tools.
Significant advancements in hardware, algorithms, generative AI (GenAI) techniques and genomic science underpin current progress and future opportunities. As a baseline, researchers have been rethinking the meaning of DNA sequences.
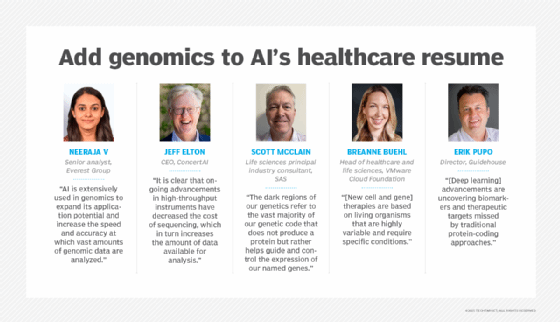
The international Human Genome Project, launched in 1990, aimed to break down the DNA molecule into millions of sequences to identify specific pieces associated with encoding proteins. But this focus was only a small percentage of all DNA, and the rest was called junk DNA. More recently, scientists discovered that these generic and repeating sequences play a significant role in controlling the expression of proteins, which has important implications for cancer research and disease progression.
Next-generation sequencing (NGS) platforms developed by DNA sequencing companies Illumina and Oxford Nanopore Technologies have transformed how data is generated, according to Pupo. The tools dramatically improve sequencing speed and cost efficiency and run directly off cloud platforms, such as AWS or Microsoft Azure, so the generated data can be quickly stored and retrieved. Specialized computing hardware, such as automated lab systems developed by Fluidigm, also improve sample throughput. In addition, platforms like Nvidia's Parabricks suite and Google's DeepVariant -- built on advanced deep learning architectures, such as recurrent neural networks and convolutional neural networks (CNNs) -- are improving the scale at which genomic data can be analyzed.
"It is clear that ongoing advancements in high-throughput instruments have decreased the cost of sequencing, which in turn increases the amount of data available for analysis," said Jeff Elton, CEO at SaaS and data platform provider ConcertAI. Using circulating tumor DNA analyses, researchers and doctors collect sequencing data from individual cancer cells derived from a patient's tumor and in blood samples. Parallelized workflows on GPUs and AI improve instrument throughput and accuracy while accelerating analysis. These tools and workflows include Nvidia's Clara for Genomics and Parabricks for accelerating the performance of traditional tools.
Mohan Uttarwar, CEO and co-founder of precision oncology diagnostics lab 1Cell.ai, points to new custom genomic chips that are less likely to miss mutations, thereby improving parameters such as sensitivity, specificity and detection limit -- moving from single omics to multiomics with multidimensional multimodal complexities. To process raw genomics data, Uttarwar said, significant progress has been made in hardware.
There have been considerable advancements in the algorithms needed to make sense of genomics data. The primary machine learning (ML) approach is CNNs, which can analyze genomic sequences for pattern recognition, Pupo explained. Standardized transformers, such as those used in deep natural language processing, can process the long-range data dependencies often found in genomics. Generative models, used by companies such as AI platform provider Atomwise, simulate molecular structures to predict gene or protein interactions, aiding in drug discovery.
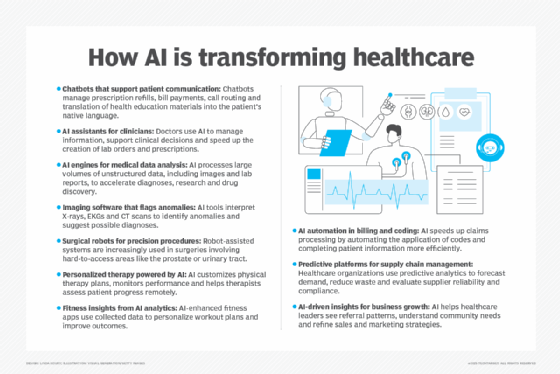
Large language models could potentially translate nucleic acid sequences to language, thereby unlocking new opportunities to analyze DNA, RNA and downstream amino acid sequences, said Aber Whitcomb, CEO of AI development and platform provider Salt AI. New models, such as Evo2 by Arc Institute, are demonstrating the power of AI in DNA analytics, while companies like Google X spinout Heritable Agriculture are showing AI's power in such applications as industrial biotech for agriculture. In clinical applications, oncology has been a trending therapeutic area for AI implementations, partly driven by the data explosion due to NGS.
To enhance genomic data aggregation for analysis, cloud providers are playing a key role, Pupo noted. Cloud-based genomic platforms, such as Illumina Connected Analytics and AWS HealthOmics, support seamless workflows that integrate NGS outputs directly into AI-powered analyses. These platforms also support the formation of new communities of healthcare researchers and institutions, leading to the creation of a network of more than 800 connected institutions, acknowledged Jurgi Camblong, CEO and co-founder of healthcare analytics software provider Sophia Genetics. These institutions upload nearly 30,000 genomic profiles monthly and more than 350,000 annually. The uploaded data trains algorithms to better harmonize, standardize and detect variants so researchers can share their knowledge with the community's membership.
How AI can ease genomics challenges
Sequencing the first human genome took 13 years and almost $3 billion. Today, we can sequence a human genome much faster and much cheaper. But sequencing the genome is only the first step. "Although tremendous advances have been made in accelerating the use of AI in genomics," Neeraja V said, "there are notable challenges with analyzing vast volumes of genomics data."
Deriving helpful information from the genome to provide targeted and personalized medical treatment will require advanced analytical capabilities powered by AI. Camblong elaborated on the many challenges to practicing precision medicine and where AI can be effective:
- Data volumes are massive. A typical human genome requires 3 GB of data storage space. Sequenced genomic data is outputted into a Variant Call Format (VCF) file, which includes information on the 3 billion base pairs of human DNA expressed in the form of ACGT. This simple text data alone can be up to 3 GB in size. AI can be used to scan the human genome and extract only pertinent pieces of information by using pattern recognition and algorithms to understand when to dig deep and when to stay at a higher level. It also can be used to optimize data storage sizes.
- Data is messy. No two human genomes are alike, and even an individual's genomic data will vary according to the technology used to generate it. Other factors might affect the data output as well, such as the test tube used to collect the sample or the chemistry used to extract the DNA. Genomes are also in a constant state of biological flux. It is estimated that about 1 billion chemical reactions occur in a single human cell every minute, creating a difficult playing field for analysis. AI plays a critical role in harmonizing and standardizing genomic data. It can clean up missing data using ML techniques that recognize patterns in similar information and sort human genomic data so that one genome can be compared to another, which is critical for the data analysis step in the process.
- Genome variants are hard to detect. AI uses complex techniques to identify alterations or deletions. Algorithms read and compare genomics to reference data and spot critical differences. Thousands of research papers have been written on the various methods used.
- Lives could depend on prompt and accurate results. AI technology can expedite genomic processing and analysis, especially when a patient's life hangs in the balance. It also can reduce false positives and negatives in patient outcomes.
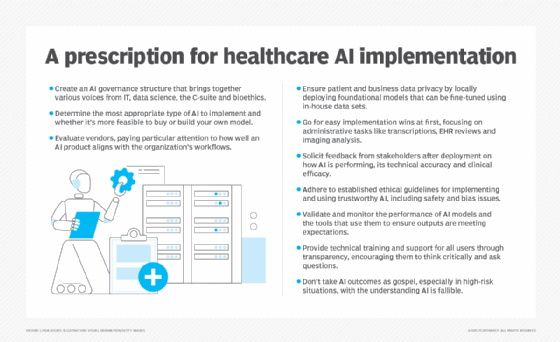
AI genomics in health research
There's speculation that AI genomics tools could be combined to make genomics data more accessible, enhance various aspects of health research and open new opportunities for discovery in many key health sciences-related areas.
Spatial biology
Gene expression in the human body is not uniform, and it's further complicated by diseases like cancer. Spatial biology seeks to combine specialized microscopic analysis and genetic sequencing to understand how gene expression occurs in individual cells and organelles, which are subcellular structures that perform specific jobs in the cell.
Dark DNA
Raw DNA comprises a long series of repeating bits punctuated by a few distinct bits to make proteins. Protein bits have been relatively easy to sequence, while the rest is much harder. Until recently, these lesser-repeating bits were mostly characterized as dark or junk DNA. Researchers now believe these bits are responsible for deciding which protein sequences to express.
"The dark regions of our genetics refer to the vast majority of our genetic code that does not produce a protein but rather helps guide and control the expression of our named genes," explained Scott McClain, life sciences principal industry consultant at analytics and AI software provider SAS. Researchers believe that precise control of the continuous on/off nature of gene expression isn't distributed equally throughout the human body or even within one organ.
Spatial biology and the associated physical chemistry and algorithmic knowledge graphing of the data an individual receives down to the single-cell spatial level are helping to decode the interconnected nature of genetic operations. New medical breakthroughs will come from data at this subcellular spatial level, which will, in turn, provide new insights into biological processes.
Deep learning models by startups such as biotech company Deep Genomics identify regulatory patterns in dark regions associated with disease development. "These advancements are uncovering biomarkers and therapeutic targets missed by traditional protein-coding approaches," Pupo noted. But results depend on the availability and quality of spatial data.
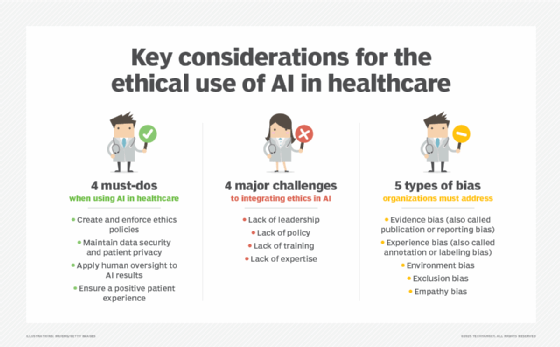
Omics
Genomics plays an essential role in broader research into the wider field of omics, which examines the interplay of genes and proteins across different levels of abstraction, including RNA transcription (transcriptomics), proteins (proteomics) and metabolism (metabolomics). "Technological advances have enabled delving into specific areas beyond genomics like epitranscriptomics, epiproteomics, DNA-RNA interactomics, RNA-RNA interactomics and DNA-protein interactomics," Neeraja V reported. "Projects like the Human Cell Atlas, a global consortium aiming to map 37 trillion cells in the human body and understand human biology and disease using advanced omics platforms and AI, are expanding the potential of omics and spatial biology across various biological applications."
Gene editing
Novel gene editing approaches, in theory, could program the behavior of certain genes. Genes responsible for cancer production, for example, could be programmed to be less prevalent, and the genes integral in regrowing cartilage that's lost to osteoarthritis could be programmed to be more prevalent. But the science behind this approach is considered haphazard. Researchers are exploring how AI could improve gene editing techniques and reduce the side effects.
CRISPR (clustered regularly interspaced short palindromic repeats) gene editing capabilities using AI might become the analytics tool of choice in identifying, targeting and programming genes, McClain said. AI is also more capable of thoroughly integrating the data across all the physiological processes involved in a particular gene to ensure the safety and efficacy of a new drug or therapy. More precise genome modifications at scale might be aided by techniques such as Perturb-seq, prime editing and Shuffle-seq, added Ron Mazumder, partner at Illumina Ventures.
Gene therapies
Advances in AI and genomics could become essential in manufacturing new cell and gene therapies, said BreAnne Buehl, head of healthcare and life sciences at Broadcom's VMware Cloud Foundation. Novel AI and genomic approaches could ensure consistent and repeatable processes. "After all, these therapies are based on living organisms that are highly variable and require specific conditions," she explained.
Many organizations, Buehl noted, are doubling down on personalized medicine and bringing the manufacturing process closer to the patient -- sometimes, directly into the hospital. Because of the highly sensitive nature of genomic data and the IP surrounding any manufacturing process, organizations are bringing these workloads on-premises or in a private cloud for utmost security, privacy, cost control and scalability. Children's Hospital Los Angeles, City of Hope and Cedars-Sinai are actively using gene therapy techniques.
What's ahead for AI genomics
The combination of AI and genomics promises even greater advancements in healthcare. Advances in GenAI, Neeraja V surmised, will help build predictive models that can identify genetic variations, their impact on populations and their links with specific diseases to guide personalized medicine. GenAI will also play a significant role in synthetic genomics, creating new genomes and improving gene editing capabilities.
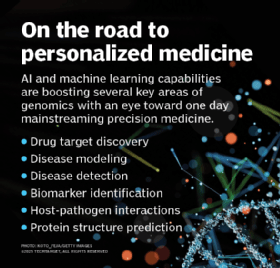
Pupo outlined the developments necessary to ensure scalability, adaptability and real-world success for AI in genomics, including the following:
- Multimodal data integration. AI must integrate data sets spanning genomics, proteomics and imaging to deliver holistic biological insights. Genomics company Helix is among the leaders in these integration efforts.
- Real-time analysis. With on-device processing platforms emerging, such as Google's AI Edge, genomic analysis at the point of care is closer to reality. Guidehouse has been working with Google to increase AI capabilities in IoT devices.
- Standardized biobanks. Training data consumption in genomics is still a challenge due to the large data sets and the need to access large amounts of data at once when training AI models. Biobanks, such as those managed by UK Biobank, are critical for ensuring diverse data is represented for inclusive AI training.
- Regulatory frameworks. Genomics AI frameworks are either limited in scope or nonexistent. Governments and agencies, including the FDA, are setting more clearly defined guidelines to validate the clinical usability of AI tools.
Meanwhile, more targeted treatments, including therapies designed for specific biomarkers, will continue to evolve, Camblong said. Uttarwar predicted that new multimodal GenAI techniques will improve the ability to integrate multiomics data by combining genomics, transcriptomics, proteomics and epigenomics to examine disease mechanisms. AI will help with risk prediction, assessing genetic variants in disease, designing more efficient and precise therapies and drug targets, and patient-specific tailored therapies, specifically AI-driven matching of patients to specific therapies.
George Lawton is a journalist based in London. Over the last 30 years, he has written more than 3,000 stories about computers, communications, knowledge management, business, health and other areas that interest him.