The cost of AI in healthcare explained: Is it worth it?
AI projects incur obvious deployment costs as well as hidden costs such as change management. Tech adopters must consider the total cost of ownership when weighing AI use cases.
Business and technology leaders in healthcare have a long list of artificial intelligence use cases to evaluate for piloting and possible deployment.
Drug discovery for pharmaceutical companies, clinical documentation for physicians, revenue cycle management for hospital operations and digital assistants for insurance plan call centers are just a few areas where healthcare organizations can reap the benefits of AI. But each AI initiative comes with a price tag. The cost of implementing AI will vary according to a project's complexity, infrastructure requirements, technical integration needs and deployment approach.
AI adopters must also consider hidden expenses as well as obvious big-ticket items. Training and change management costs might be overlooked, for example, amid widely acknowledged expenses such as training a large language model (LLM). A deployment's ongoing operation and maintenance costs are also considerations.
Cost considerations for AI implementation and maintenance
Getting a handle on the cost of AI in healthcare helps organizations evaluate use cases and select those with the highest ROI potential. Eight cost factors should be considered when deploying a new AI project.
This article is part of
AI in healthcare: A guide to improving patient care with AI
- Which also includes:
- Health IT (health information technology)
- precision medicine (PM)
- telehealth (telemedicine)
1. Building an AI team
Businesses adopting AI will generally need a team to set a strategy, identify use cases, establish success metrics and manage projects. That group might be called an AI center of excellence, a governance organization or an AI accelerator.
Whatever the name, the organization charged with guiding AI projects will require specialized staffing, and their pay will likely be the biggest cost contributor. The average total compensation in the U.S. for an AI director, who might lead the AI group, is about $200,000, according to open positions listed on the Glassdoor jobs website. Dice, an IT careers website, estimated a project manager's average salary at $121,110 and a data scientist's pay at $106,130. An AI ethicist's salary could run in the $80,000 to $120,000 range, according to Edmates, a career guide platform.
So, even a small AI center of excellence or accelerator could easily cost $500,000 in salaries alone.
An AI team should provide "innovation muscle" that guides projects, rather than an onerous process that impedes them, advised Dr. Bill Fera, a principal at Deloitte Consulting. "It's building trust that you can move quickly to identify the right use cases and deploy them, measure them and, if they are working, scale them quickly -- and, if they are not, move on to the next one," he explained.
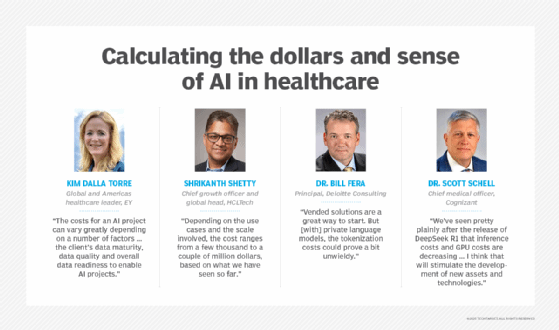
2. Getting a grip on data collection and preparation
Wrangling data for use in AI models and data analytics is one of the top challenges to adopting the technology. It's also a notable cost contributor.
Organizations must harmonize data in disparate formats and focus on tasks such as cleansing, deduplicating and collating. Industry executives recommended healthcare organizations set up data management and governance programs to coordinate such activities, address regulatory compliance and ensure proper use of collected data.
Those structures are sometimes absent or not fully mature in healthcare organizations. But a lackluster approach to data won't suffice when healthcare organizations plan to pursue AI at scale, said Shrikanth Shetty, chief growth officer and global head for life sciences and healthcare industries at IT consultancy HCLTech. "The first thing they have to do," he advised, "is create a strong data governance focus."
Developing such a focus requires investment in personnel such as chief data officers, data stewards and data architects. A healthcare organization might also need to upgrade to a modern, cloud-based data infrastructure. Costs are highly variable because cloud data warehouses such as Amazon Redshift, Google BigQuery, Microsoft Azure Synapse Analytics and Snowflake charge customers based on the amount of storage they consume.
"Across payers, providers and life sciences, the move toward cloudification of data has long been underway," acknowledged Dr. Scott Schell, chief medical officer at IT consulting and outsourcing services company Cognizant. Though historically pricey, he added, cloud data costs are moderating. "I think that all the hyperscalers are now, in many ways, in a level playing field," he surmised. "Commoditization has driven down the cost."
3. Style of deployment
With an AI strategy and clean data, an organization can move on to the adoption phase. The style of deployment will determine the cost.
At the high end, the cost of building a fully customized LLM starts at around $2 million and goes up from there. This approach requires a business to acquire the necessary infrastructure, such as GPU clusters. "Very few [healthcare organizations] are doing this on their own," Shetty noted.
Healthcare entities, however, can also opt to fine-tune a third-party LLM with its own domain-specific data. This approach provides a level of customization at a lower cost than a built-from-scratch model. "Depending on the use cases and the scale involved," Shetty said, "the cost ranges from a few thousand to a couple of million dollars, based on what we have seen so far."
But perhaps the least expensive option is tapping into the array of generative AI (GenAI) tools available on an off-the-shelf basis. Options include Google's Gemini and the healthcare-specific MedLM, OpenAI's ChatGPT and Microsoft's various Copilot products. Microsoft Dragon Copilot, a clinical documentation tool unveiled in March, will be available in May in the U.S. and Canada.
"AI is going to become very rapidly infused in all types and all areas of just about every application that we see," Schell said. "There's going to be a large block of capabilities that can be deployed generically." These capabilities offer limited customization, he added, but compensate for that with quick deployment, expanded use-case support and an affordable starting point.
Healthcare organizations employing widely available AI tools will accrue user-based charges. Prices vary by vendor, but a $25 to $40 per user, per month fee is common for organizations deploying such offerings as a GenAI strategy.
4. Type and complexity of the AI model
The bigger the model, the greater the expense has been a general rule of thumb in AI. Healthcare organizations, however, could catch a cost break based on recent developments in GenAI. The arrival of the DeepSeek R1 LLM in February suggested that capable AI models could be built with a much smaller investment than previously believed.
"We've seen pretty plainly after the release of DeepSeek R1 that inference costs and GPU costs are decreasing," Schell said. "That is a huge positive. I think that will stimulate the development of new assets and technologies."
DeepSeek, based in Hangzhou, China, provides its R1 models under an open source license. DeepSeek can be used as a GenAI tool along the lines of ChatGPT, but organizations can also develop AI applications on it.
This platform approach -- a characteristic of DeepSeek R1 and similar offerings -- changes the economics of language models, according to Fera. "It speaks to a necessary pivot from vended solutions to platform-based solutions where people can use open source models to decrease costs," he explained. "Vended solutions are a great way to start. But [with] private language models, the tokenization costs could prove a bit unwieldy."
Tokenization is the system GenAI vendors use to price access to their LLMs. The number of tokens an enterprise consumes depends on frequency of use and the length of a GenAI tool's responses. This variable cost ranks among the key expenses businesses need to consider when calculating ROI.
The lower cost of open source models could attract more healthcare providers, which Fera said have tended to work with vendors. "DeepSeek proved that they are entirely adequate to do most jobs," he said. "If you could train those models to be fit for purpose, I think providers will start to realize how accessible that is."
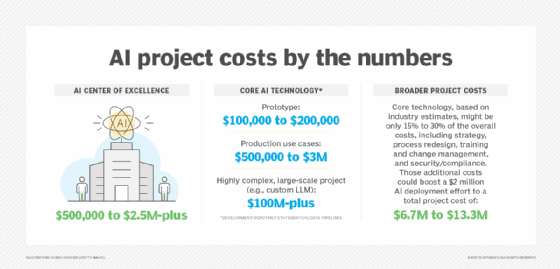
5. Integration with other systems
The complexity of legacy systems integration lurks within the healthcare industry's broader healthcare-AI integration challenge. That task adds another cost to an enterprise AI project.
"A typical healthcare organization will be composed of many different systems that aren't connected and often require bespoke integrations," said Darren Challender, client engagement partner at Hitachi Digital Services.
Kim Dalla Torre, global and Americas health leader at consultancy EY, also pointed to the issue of outdated systems among payers and providers. "We are dealing with a lot of legacy systems that are operating in silos, and a lot of our clients are at a point where they have decided they've got to modernize," she said. "From the infrastructure perspective, it's a couple of factors. We have old electronic health record systems that need to be modernized and brought to the cloud. We have a lot of point solutions that don't talk to each other and prevent interoperability from happening."
Modernization costs add to the price tag of AI integration. The legacy systems disconnect also results in differing data formats and triggers quality issues. Those problems, Challender noted, only grow when considering the data that wearables and IoT devices generate. This mix of data requires lots of preprocessing -- cleansing and organizing -- before it can be brought together, he added.
The data preparation stage tends to be expensive, especially for organizations that must preprocess very large and complex data sets. But the expense doesn't end there. Organizations must invest in security as they harmonize, cleanse and organize data. "All of this has to be done with a key metronome focusing on safeguarding patient information and complying with HIPAA-related standards," Schell said.
6. Compliance and risk management costs
HIPAA, the U.S. Food and Drug Administration's 21 Code of Federal Regulations Part 11 and the EU's GDPR represent a broad compliance mandate for healthcare organizations.
"Compliance, security and risk management practices are some of the important areas to focus on," said Mahmood Majeed, managing principal at management consultancy ZS. "To address these, we see that clients are developing structures, governance platforms and dedicated ethics committees to oversee AI." He also emphasized the need to integrate security into AI development from the outset as a security and risk management practice.
7. Change management and training costs
Preparing employees for new ways of working is sometimes overlooked or underestimated as an important component in the adoption of AI. But change management, a systematic approach to process and technology change, is critical for transformational projects such as AI adoption. It's also potentially a big piece of an AI project budget. In the case of GenAI costs, McKinsey & Co. reported that organizations should plan to spend as much as three times more on change management than they do on development.
Within change management, training will also contribute to the overall cost of a project. "Integrating AI has a huge technical debt from the perspective of training the users," Schell said. Training must include physicians and nurses on the front line as well as people working in back-office functions, he added.
8. Ongoing operations and maintenance
The end of an AI project doesn't mean the end of investment. Healthcare organizations that use off-the-shelf GenAI tools will have pay-as-you-go tokenization fees. Healthcare entities that build or fine-tune models must keep them up to date.
AI models and algorithms will need to be monitored, retrained, tuned and governed to ensure their accuracy and applicability, Challender said. Maintenance costs could also increase as AI systems scale, he noted, but the possibility of higher efficiency in the near future could bring those expenses down. Challender cited DeepSeek's reduced need for computer power as a development that could lower maintenance costs.
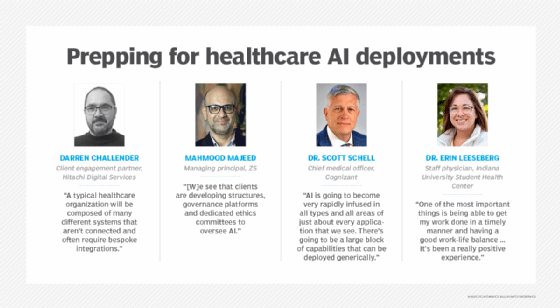
Estimating how much AI healthcare projects cost
Calculating an AI project's cost is a complicated chore, given the multitude of cost considerations.
"The costs for an AI project can vary greatly depending on a number of factors," Dalla Torre said. "First, there is the obvious complexity of the AI capability being developed, but perhaps even more important is the client's data maturity, data quality and overall data readiness to enable AI projects."
Many healthcare organizations, she reported, need help getting their data in place to support AI development -- an undertaking that includes infrastructure, platforms and governance.
The development and implementation costs of custom AI capabilities and tools cover a wide range of price points. A low-complexity prototype can be built for $150,000 to $200,000, Dalla Torre surmised. But the cost to integrate such a prototype and ensure production readiness for all components and connections, she added, could cost two to three times that amount or more. For a more complex AI project, development and integration might start in the $1 million-plus range, Dalla Torre said.
The actual price tag depends on factors such as how many heterogeneous data sources need to be brought together and the complexity of the AI implemented. And the cost of training an LLM -- among the most expensive AI projects -- can run into hundreds of millions of dollars at the high end.
While AI development costs are an obvious source of expense, AI adopters should also be wary of latent costs. The primary costs healthcare clients overlook are associated with training as well as operations and maintenance, Dalla Torre noted. Cloud consumption, she said, is another potentially hidden cost that organizations must consider. Fera also cited consumption/compute cost as a factor clients must consider when calculating total cost of ownership.
The good news is cost tends to go down as organizations take on more AI projects. After the initial use case, the cost of the next use cases using the same data sets drops by 30% to 40%, Fera said. Subsequent use cases, he conjectured, could see even steeper cost decreases, depending on a use case's complexity.
How can AI reduce costs in healthcare?
While an AI deployment carries significant overhead, the technology also offers potential cost savings.
Industry executives see revenue cycle management as one area where AI can trim costs. Fera cited the example of AI-generated appeal letters responding to insurance companies' prior authorization denials as one of the use cases in the revenue cycle. "Those can be huge financial wins for provider organizations," he said.
On the payer side, claims processing and waste, fraud and abuse detection are among the leading AI use cases for cost reduction, Dalla Torre said. Such applications, she added, help cover the cost of AI and pave the way for the next wave of healthcare use cases. "We are now moving toward patient- or member-experience improvement and using AI in a way that helps them navigate the healthcare system better," she said.
She also pointed to a movement toward using AI in medical imaging and integrating AI with wearables for chronic disease management. Those applications, she explained, result in reduced hospital stays, lower costs and better patient outcomes.
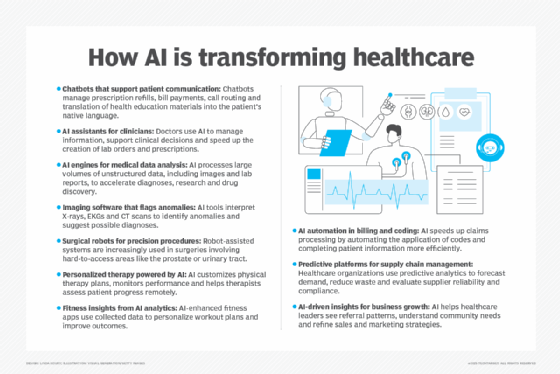
Weighing the value of AI projects
Organizations can assess an AI project's value in various ways. Majeed said he considers value capture and value creation. Value capture focuses on a healthcare organization's existing business and looks for results such as cost reduction, improved decision-making or better patient engagement. Value creation seeks new sources of revenue, such as launching a data business.
Meanwhile, healthcare organizations should weigh ROI across financial, experience and satisfaction dimensions, Fera advised. Ideally, a healthcare provider, payer or life sciences company would generate returns across all those areas.
A single project might span more than one ROI target: A 2024 Deloitte study identified R&D as the No. 1 value opportunity in the life sciences industry and reported that accelerating drug development "could provide both cost savings and revenue uplift."
Other healthcare AI use cases, such as clinical documentation, can deliver results across experience and satisfaction measures. Dr. Erin Leeseberg, a staff physician at Indiana University's Student Health Center, has been using Sunoh.ai's note-taking product since 2024. She said Sunoh reduces her note-taking chores by 5 to 15 minutes per patient. Automation of a previously manual task has cut back on the time she spends working on clinical notes during lunch or after work.
"One of the most important things is being able to get my work done in a timely manner and having a good work-life balance," Leeseberg said, adding that she can also work more efficiently on behalf of her patients: "It's been a really positive experience."
John Moore is a writer for Informa TechTarget covering the CIO role, economic trends and the IT services industry.