AI and HIPAA compliance: How to navigate major risks
Balancing AI deployments with HIPAA compliance can be fraught with numerous risks. The first step is awareness, and the second step is to follow best practices to avoid pitfalls.
The use of artificial intelligence in healthcare among physicians has shown a significant increase over the past year. A 2025 survey from the American Medical Association found that 66% of physicians said they use AI in their practices compared to just 38% in 2023, and more than two-thirds of physicians surveyed indicated they see advantages to using AI tools.
While healthcare's expanding use of AI provides benefits, it also complicates the industry's ability to protect patient information and remain compliant with the data protections required by the Health Insurance Portability and Accountability Act (HIPAA). In fact, the risks from "AI-enabled health technologies" topped the list of the most significant technology hazards in the healthcare industry, according to the "Top 10 Health Technology Hazards for 2025" report by the nonprofit Emergency Care Research Institute, better known as ECRI.
Some observers believe AI vastly expands the work required to secure and protect patient data. "If we hadn't had a problem with data governance before, we have it now with AI," said Tony UcedaVelez, CEO and founder of consultancy VerSprite Security. "It's a new paradigm for [personally identifiable information] governance."
How AI is used in healthcare
The 2024 HIMSS Healthcare Cybersecurity Survey revealed that AI is used for such technical tasks as support and data analytics, clinical care applications like diagnostics, and administrative tasks such as virtual meeting transcription and content creation. AI is also used for healthcare operations, research, patient engagement and clinical training.
This article is part of
AI in healthcare: A guide to improving patient care with AI
- Which also includes:
- Health IT (health information technology)
- precision medicine (PM)
- telehealth (telemedicine)
AI "is being incorporated into almost all aspects of a healthcare lifecycle," acknowledged Ashley Casovan, managing director of the International Association of Privacy Professionals (IAPP) AI Governance Center. "AI is helping researchers to discover new treatments and clinical therapies more efficiently. Within day-to-day healthcare operations, AI systems are used in ambulatory care to assist with time-sensitive diagnosis and to improve patient and care-provider interaction. In the hospital, AI is used to assist healthcare providers with real-time information, use robotics to assist with delicate surgeries, and in hospital administration to improve patient experience. For ongoing care, virtual AI assistance is becoming more common."
The healthcare industry's use of AI is often aimed at improving operational efficiency as well as patient experiences and patient outcomes, said John B. Sapp Jr., vice president of information security and CISO at Texas Mutual Insurance Company. In such cases, the healthcare sector uses AI the same way as other sectors. AI systems such as chatbots and generative AI (GenAI) tools analyze customer and patient data to help doctors, nurses, administrators, customer service representatives and the patients themselves get the accurate, personalized information they need as quickly as possible.
AI works much the same way in clinical areas, Sapp added. AI models trained on vast amounts of historical data help clinicians render care to current patients. Here, AI tools handle a range of tasks, including interpreting the results of patient imaging exams, advising on diagnoses and recommending optimal treatment.
Additionally, AI is used in medical devices, surgical robotics and wearables, where the technology enables "real-time intraoperative decision support, precision automation and adaptive learning based on patient-specific data," explained Bhushan Jayeshkumar Patel, IEEE senior member and program manager at minimally invasive care and robotic-assisted surgery provider Intuitive. AI also aids in remote patient monitoring, Patel noted, "by leveraging real-time biometric data to predict and prevent health crises."
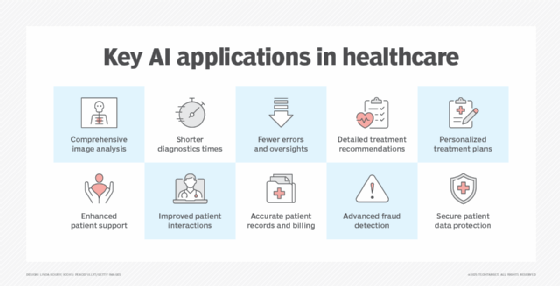
How AI jeopardizes HIPAA compliance
Despite its many benefits in healthcare, AI presents challenges to a healthcare organization's ability to comply with HIPAA standards for safeguarding protected health information (PHI). "There are concerns about where data lives, who has access to it and how that data is being used," said Pam Nigro, vice president of security and CISO at clinical data platform provider Medecision. "It's something that tech departments struggle with every day."
All iterations of AI run on data -- from the classic machine learning algorithms that have long been used in healthcare to more recent AI tools such as ChatGPT and open source large language models (LLMs). Much of the software used in healthcare applications now contain AI, and it might be unclear to healthcare organizations whether the software safeguards data in compliance with HIPAA standards, said Nigro, who's also a director at the professional IT governance association ISACA.
"AI-driven tools pose HIPAA compliance risks if PHI data is not securely managed at rest or in transit," said Sinchan Banerjee, IEEE senior member. "Many advanced AI tools are cloud-based, and making useful data available to those tools without compromising on data security is a complex challenge."
Healthcare industry experts specified eight ways AI can jeopardize HIPAA compliance.
1. Regulatory misalignment
"Traditional HIPAA frameworks were not designed for real-time AI decision-making," Patel said. "For instance, an AI-driven surgical guidance system adjusting intraoperative parameters dynamically must align with HIPAA while ensuring split-second clinical decisions are not hindered."
2. Cloud-based data transmissions
Many medical devices, such as surgical robots and wearables, transmit patient data to cloud-based platforms, increasing exposure to breaches, Patel said.
3. Data exchanges with outside parties
Because so many AI models are cloud-based or part of SaaS applications, healthcare organizations are moving patient data outside their own data protection measures and into those cloud-based applications, Sapp explained. Movement among clouds increases the risk of breaches and inadvertent exposure of the data. It also complicates the healthcare organization's ability to confirm that the data shared with cloud-based AI models is protected and secured to HIPAA standards.
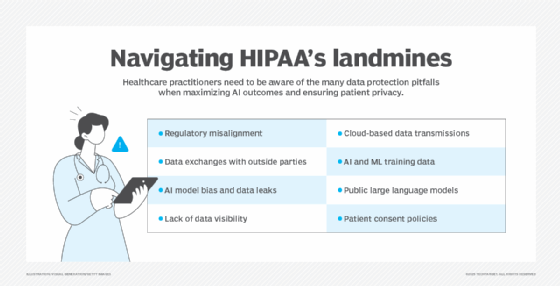
4. AI and ML training data
"Ensuring AI training data remains compliant is complex, as unencrypted, non-tokenized or non-de-identified data can lead to HIPAA violations," Banerjee warned.
5. AI model bias and data leaks
Patel cautioned that "some AI algorithms inadvertently retain PHI from training data, raising concerns about unintended data leaks." Federated learning techniques, he advised, can mitigate this risk by processing data locally on devices instead of centralized servers.
6. Public large language models
Employees could inadvertently expose protected data by using public LLMs. Sapp said an employee who uses a GenAI tool to craft a letter to a patient about treatment options might upload details that expose the patient's protected data. Clinicians, administrators or support staff, UcedaVelez added, might use a public LLM to help them transcribe notes, similarly exposing protected data.
7. Lack of data visibility
Healthcare organizations might lack visibility into whether any data they shared or stored with vendors is being analyzed by vendors for their own use, in ways that would violate HIPAA regulations, UcedaVelez said. "There are a number of large entities -- insurance, medical manufacturers -- who have large data sets that they're tapping into to optimize their products and services," he explained.
8. Patient consent policies
Healthcare organizations might find that the consent policies they share with patients don't adequately inform their patients about how patient data is used with AI tools, Sapp said.
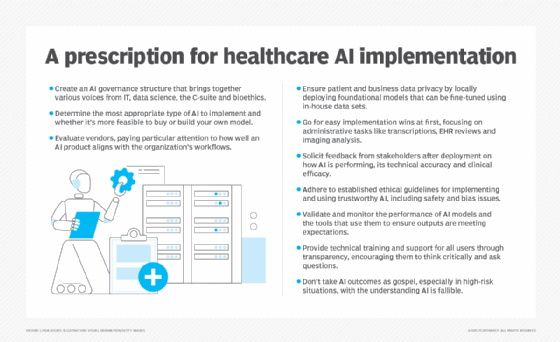
Best practices for using AI in compliance with HIPAA
Despite the complexity of the application, healthcare organizations can't let AI use cases compromise their ability to comply with HIPAA.
"AI systems are not a special scenario falling outside of these existing robust compliance obligations," said Cobun Zweifel-Keegan, IAPP managing director, Washington, D.C. "AI is just like any other technology -- the rules for notice, consent and responsible uses of data still apply. HIPAA-covered entities should be laser-focused on applying robust governance controls, whether data will be used to train AI models, ingested into existing AI systems or used in the delivery of healthcare services."
Healthcare industry experts offered 12 best practices to navigate the major risks and ensure AI is applied in healthcare to derive the maximum benefit while complying with HIPAA regulations.
1. Develop clear policies, procedures and codes of conduct
Create specific rules on the application of AI and how, when and where the technology can be used in compliance with HIPAA. "We want to encourage the adoption of AI," Sapp said, "but it must be secure and responsible."
2. Ensure third-party contracts address AI risks
Organizations should ensure their partners, vendors and suppliers meet the security and data protection standards required to mitigate AI risks, Nigro advised. Healthcare entities, she noted, should review existing contracts to ensure they address those needs and, if not, establish new agreements.
3. Establish a strong governance program
Governance should ensure employees, partners and vendors are educated and trained to follow and comply with the healthcare organization's policies, procedures and code of conduct, UcedaVelez said.
4. Institute a comprehensive risk management program
A well-crafted AI strategy and strong AI governance program do not eliminate risks without a strong risk management strategy, Sapp said.
5. Implement security measures
Healthcare entities should identify and establish the security measures necessary to mitigate risks as outlined in a risk management strategy. UcedaVelez recommended encryption, networking traffic monitoring and access control tools as well as a strong access management program.
6. Select appropriate types of AI tools and software
AI tools have varying levels of security and protection built in, with public LLMs and GenAI tools having the greatest likelihood of exposing data, Nigro said. Therefore, healthcare organizations must provide clear guidelines for selecting and implementing software and AI tools to ensure they align with organizational security and data privacy requirements.
7. Incorporate secure-by-design principles
Another way to help protect sensitive data is to build security and privacy into the AI models and tools. "AI has to have privacy by design as a core tenant," Sapp said.
8. Install a zero-trust security architecture
"Implementing multifactor authentication, granular access controls and encryption ensure that AI-powered devices and robotic systems remain compliant with HIPAA while protecting PHI," Patel said.
9. Use edge AI and on-device processing
Instead of relying solely on centralized cloud processing, Patel suggested running AI models and algorithms directly on such devices as surgical robots or wearable devices at the edge of a network to minimize data exposure risks.
10. Consider federated learning for AI training
"Instead of centralizing PHI for AI training," Patel explained, "federated learning enables AI models to be trained across multiple local devices without sharing raw patient data. This approach is gaining traction in digital health."
11. Perform regulatory sandboxing
"AI systems used in robotic-assisted surgery and digital health wearables should undergo continuous auditing, bias detection and explainability testing to ensure compliance without compromising AI performance," Patel advised.
12. Seek legal support
The legal and compliance teams should work in tandem with technology and business teams, Sapp recommended, so they understand the risks posed by AI tools as well as how to mitigate those risks and ensure compliance with HIPAA, other regulations, industry standards and enterprise standards.
Mary K. Pratt is an award-winning freelance journalist with a focus on covering enterprise IT and cybersecurity management.