What is emergent medical data?
Emergent medical data (EMD) is health information gathered about an individual from seemingly unrelated online user behavior data. The term was coined in 2017 by Mason Marks, a professor at Florida State University College of Law.
Companies gather EMD from various online sources and then analyze that data using AI and machine learning (ML) tools. This is done with the purpose of creating detailed profiles of an individual's physical and mental health.
Collecting EMD produces several benefits, including the ability to track the spread of an infectious disease, identify individuals at risk of suicide or homicide and monitor drug abuse. However, the primary appeal behind emergent medical data is the opportunity for organizations to enhance behavioral targeting and optimize customer profiling and marketing.
Insurance companies can use EMD to determine an individual's accident risk and calculate insurance premiums. Advertisers can use personal medical data to deliver behavioral ads based on the individual's medical history.
This article is part of
AI in healthcare: A guide to improving patient care with AI
- Which also includes:
- Health IT (health information technology)
- precision medicine (PM)
- telehealth (telemedicine)
As a result, EMD has raised many concerns around personal and patient privacy. Patient data collected by healthcare providers is protected by the Health Insurance Portability and Accountability Act (HIPAA), but EMD receives little to no legal protection.
How emergent medical data works
Traditional forms of medical data include health information such as a patient's medical record or prescription records. This information is typically gathered in clinical settings and is protected by privacy laws like HIPAA.
Instead of being obtained directly from patient or hospital records, emergent medical data is obtained indirectly.
The process starts with a person’s interactions with the technology around them. The use of smartphones, desktop computers, wearable devices, and online platforms and services yield browsing and shopping behavior histories that represent disconnected digital traces. Individually, this data might not mean anything, but, when gathered together, it can start to reveal patterns that indicate a person's health status.
Companies can pull this seemingly unrelated data from a variety of sources, including Facebook posts, credit card purchases, the content of emails or a list of recently watched videos on YouTube. AI and ML models are then used to detect correlations and patterns between the user's data and known health conditions. For example, certain search terms might correlate with depression, or large language models could be used to rake through a user's social media to detect signs of anxiety. The result of analyzing all this data is EMD -- inferred health information that does not act as a medical diagnosis.
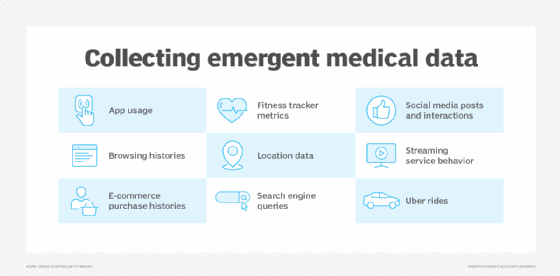
What kind of data does EMD include?
When people interact with technology, they leave a digital footprint of their actions and behaviors. AI, ML and big data algorithms collect and analyze this raw data to create EMD. As a result, almost any activity performed by an individual with technology will produce information that can be transformed into sensitive medical data.
Specific examples of information gathered in the emergent medical data mining process include the following:
- App usage.
- Browsing histories.
- E-commerce purchase histories.
- Fitness tracker metrics.
- Location data.
- Search engine queries.
- Social media posts and interactions, such as likes, shares and following lists.
- Streaming service behavior.
- Uber rides.
All this data can be analyzed to form a profile of the user's mental and physical health. As more online behavior is analyzed, more EMD is gathered, and the clearer the profile becomes.
Privacy concerns with emergent medical data
Although EMD can be a useful data source for a company, there are many public concerns around privacy and EMD. The potential risks of EMD are as follows:
- Companies can take advantage of loopholes in privacy laws and users' lack of knowledge regarding how EMD is collected to access personal medical records that are typically confidential.
- Online platforms can benefit by selling EMD to third parties that might use it as the basis for discrimination in a variety of decisions, including employment, insurance, lending and higher education.
- The more people learn about EMD, the more likely they are to start changing their online behaviors; this could significantly limit the free exchange of ideas over the internet.
- When a company puts users into groups based on medical conditions, they act as medical diagnosticians -- a role that should be reserved for trained and licensed professionals.
Emergent medical data has the potential to improve healthcare. For example, ads for evidence-based recovery centers could target users with substance abuse issues, and individuals with undiagnosed Alzheimer's disease could be referred to a physician for evaluation. However, collecting the necessary information to make this possible without explicit consent is unethical and violates individual privacy. Furthermore, the information gathered is not protected by HIPAA, and companies that gather this data are not subject to any related penalties.
One final concern around EMD is its potential to promote algorithmic discrimination, which occurs when a computer system systematically and repeatedly produces errors that create unfair outcomes -- such as favoring one group of users over another, more vulnerable group.
The machine learning algorithms used to find EMD sort users into health-related categories that are assigned positive and negative weights -- or importance. Discrimination occurs when an algorithm designed to find new job candidates assigns negative weight to groups of individuals with disabilities, thus preventing them from accessing job postings and applications for which they could have been qualified.
Algorithmic discrimination can also cause companies to wrongfully deny people access to important resources, such as housing and insurance, without realizing it.
Health and privacy laws and regulations
The various privacy concerns around EMD and personal data collection have raised the discussion around public health ethics and the boundaries for the responsible use of emergent medical data. Several governments have responded with new laws to protect personal data further, however.
In April 2016, the European Union approved the General Data Protection Regulation (GDPR), which increased consumers' rights to control their data and how it is used. Businesses that fail to comply are penalized with large fines. The GDPR went into effect in May 2018.
California passed the California Consumer Privacy Act (CCPA) in June 2018, which supports California residents' rights to control their personally identifiable information (PII). Under the CCPA, citizens have the right to know what personal data is being collected and who is collecting it, as well as the right to access their PII and refuse its sale to third parties.
In addition, U.S. Sens. Amy Klobuchar (D-Minn.) and Lisa Murkowski (R-Ark.) proposed the Protecting Personal Health Data Act in 2021, which aimed to protect health data collected by wellness apps, fitness trackers, social media platforms and direct-to-consumer DNA testing companies. The bill, as it was written, could have also posed the risk of creating an exception for EMD, however.
While the Protecting Personal Health Data Act was introduced, it never advanced out of the committee and was not voted on by the Senate or House. It was not formally rejected, however, and could be reintroduced in some form in the future.
Washington state also introduced the My Health My Data Act, which passed in 2023. The bill is meant to protect personal health data that falls outside of HIPAA from being collected and shared without consumer consent. It also grants consumers the right to access and delete their health information.
Examples of emergent medical data
American retail corporation Target hired statisticians to find patterns in their customers' purchasing behavior. Data mining revealed that pregnant female shoppers were most likely to purchase unscented body lotion at the start of their second trimester. Before analyzing this data, unscented body lotion had no recognized connection to a health condition. However, using this information, Target was able to reach out to consumers identified as expectant mothers with coupons and advertisements before other companies even learned the women were pregnant.
Studies involving Facebook also reveal how nonmedical online behavior can be used to find users with substance abuse issues and other disease risks. For example, one study showed that the use of swear words, sexual words and words related to biological processes on Facebook was indicative of alcohol, drug and tobacco use. Furthermore, the study determined that words relating to physical space -- such as up and down -- were more strongly linked to alcohol abuse, while angry words -- such as kill and hate -- were more strongly linked to drug abuse.
Facebook also discovered that the use of religious language -- especially words related to prayer, God and family -- could predict which users had or might develop diabetes. Other areas of focus include analyzing ordinary Facebook posts to determine when specific users feel suicidal.
Project Nightingale was a controversial partnership between Google and Ascension -- one of the top ten largest health systems in the U.S. The project was kept hidden until November 2019, when it was revealed that Google had gained access to over 50 million Ascension patient medical records through the partnership.
The partnership gave Google unprecedented power to determine correlations between user behavior and personal medical conditions. Patents filed in 2018 reveal that Google aimed to use Project Nightingale to increase its emergent medical data mining capabilities and eventually identify or predict health conditions in patients who have not yet seen a doctor.
While Project Nightingale could provide numerous medical benefits and improve the healthcare system, it also raised major concerns regarding personal privacy. It is likely that Google will conceal its discoveries as trade secrets and only share them with its parent company, Alphabet, and its subdivisions -- including Nest, Project Wing, Sidewalk Labs and Fitbit.
Each of these subdivisions provides a service with a data mining operation that collects and analyzes user information. Therefore, a major concern around Project Nightingale is its potential to use its collection of personal medical data to create an unprecedented consumer health surveillance system that spans multiple industries and technologies.
In November 2019, a federal inquiry was opened to investigate Project Nightingale and Google's attempt to collect millions of Americans' protected health information.
Project Nightengale was never officially canceled, but following public backlash, there haven't been any updates that indicate whether the project is still ongoing, significantly altered or shelved altogether.
Healthcare data collected by traditional means are generated, collected and processed in different ways from EMD. Learn more about the healthcare data cycle.