AI and GDPR: How is AI being regulated?
Amid data privacy issues spawned by proliferating AI and generative AI applications, GDPR provisions need some updating to provide businesses with more specific AI guidelines.
GDPR provisions debuted six years ago to help standardize European privacy and data protection frameworks. Due to the massive interest in AI, the GDPR is seen as a first line of protection against the uncertainties of new techniques, business models and data processing pipelines.
Data privacy concerns have grown more complicated with the surge in generative AI applications. Companies like OpenAI have been reluctant to share information about how their training data was collected and how they address privacy concerns pertinent to the use of these AI models.
Regulators in Italy, for example, initially blocked the rollout of OpenAI's ChatGPT, citing data privacy concerns. Four weeks later, regulators allowed the chatbot interface to large language models (LLMs) to operate, only to report privacy violations in early 2024. Privacy issues aren't limited to large AI vendors. Enterprises are starting to mix and match newer LLMs with their internal processes and data.
Privacy experts are also concerned that the GDPR didn't plan for some of the potential issues arising from new AI models. "The fact that AI can be used to automate decision-making and profiling highlights the need for regulators to implement measures that ensure such activity is done fairly and ethically," said Martin Davies, audit alliance manager at compliance automation platform provider Drata. The GDPR, for example, contains provisions for algorithmic transparency in certain defined decision-making processes. But AI systems and models can become black boxes, making it challenging for regulators and enterprise leaders charged with protecting data to understand how personal information is used within them.
This article is part of
What is data protection and why is it important?
Resolving these issues is important not just for regulatory compliance, but also to build trust in AI applications, Davies reasoned. "By balancing its rapid technological advancement with a framework that protects fundamental rights," he explained, "an environment of trust and confidence in AI technologies will be created."
GDPR's AI limitations
The GDPR has been important in advancing privacy protections in Europe and inspiring regulators worldwide. But when it comes to AI, the regulation has many shortcomings.
Ironically, one of GDPR's biggest weaknesses is also a major strength -- the "right to be forgotten" framework emphasizing individual control over personal data, according to Davi Ottenheimer, vice president of trust and digital ethics at data infrastructure software provider Inrupt. "Imagine a robot that can only be powered off but not reprogrammed, and you see the problem with AI and GDPR," said Ottenheimer, who believes "the right to be understood" would better serve the framing of the GDPR. "This will force transparency engineering into AI systems such that individuals can comprehend and challenge decisions being made," he explained.
The GDPR applies to AI whenever personal data is processed during model training or deployment, said Sophie Stalla-Bourdillon, senior privacy counsel and legal engineer at data security platform provider Immuta. Yet the regulation doesn't always apply when trained on nonpersonal data, she said, adding that the GDPR also hasn't been the most effective mechanism for identifying red flags.
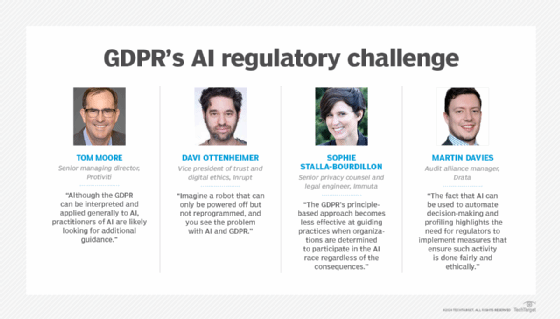
"The GDPR's principle-based approach becomes less effective at guiding practices when organizations are determined to participate in the AI race regardless of the consequences," Stalla-Bourdillon explained. Enterprises need clearer, earlier and more specific signals from the regulation to know when to hit the brakes. The EU Artificial Intelligence Act tries to fill this gap with a three-fold distinction among prohibited AI practices, high-risk AI systems and other AI systems as well as concepts like general-purpose AI systems and models.
Numerous AI risks in sectors like education, social benefits, judicial and law enforcement have often been ignored and should be considered in shaping new regulations, suggested Stalla-Bourdillon, who is also a visiting law professor at the University of Southampton, U.K. In the U.S., the COMPAS algorithm used for AI-powered sentencing in courts recommended longer sentences for black defendants compared to white defendants by unevenly predicting recidivism rates. The French equivalent of the U.S. Department of Education has been called out for an opaque college recommendation algorithm. The Dutch tax authority has been criticized for relying heavily on a flawed AI algorithm to pursue innocent people for tax fraud.
GDPR lacks specific AI guidance
The GDPR doesn't explicitly mention AI and the many new ways AI can be used to process personal information, which can create confusion among data management and technology teams. "Although the GDPR can be interpreted and applied generally to AI, practitioners of AI are likely looking for additional guidance," surmised Tom Moore, senior managing director at consultancy Protiviti. More specific guidance could further help enterprises take advantage of AI's data protection capabilities, achieve GDPR compliance and avoid the substantial penalties codified in the law. Moore said the GDPR faces several unique AI challenges, including the following:
- Transparency. GDPR's provisions for automated decision-making and profiling provide some rights to individuals, but they might not be sufficient to ensure transparency in all AI use cases.
- Bias and discrimination. The GDPR prohibits processing deemed as sensitive personal data, such as race, ethnicity, or religious beliefs, except under specific conditions but doesn't directly address the issue of algorithmic bias that could be present in the training data.
- Accountability and liability. The GDPR's provisions on data controller and processor responsibilities might not fully capture the complexity of AI supply chains, the potential for harm and who's responsible when harm occurs and multiple parties, such as developers, deployers and users, are involved.
- Ethical considerations. The GDPR doesn't directly address broader societal concerns and ethical questions beyond data protection, like AI's impact on jobs, the potential for manipulation and the need for human oversight.
- Sector-specific requirements. The GDPR provides a general framework for data protection that's not necessarily sufficient to cover industry-specific risks and challenges.
The EU AI Act takes a risk-based approach to fill these gaps by imposing requirements based on associated risk levels with specific AI applications. It also includes provisions on transparency, human oversight and accountability.
Governments want their economies to realize the benefits of AI, but society is just learning about the risks associated with it. Crafting better regulations that balance rewards and risks can require input from several sources. "The European Data Protection Board [EDPB], European Data Protection Supervisor [EDPS], national data protection authorities, academics, civil society organizations as well as commercial enterprises and many others all want to have their voices heard during any legislative process," Moore said.
Establishing regulations that are adaptable and future-proof to keep pace with technological progress, Moore noted, can be difficult and time-consuming. Previous examples of European technology protection legislation, including the GDPR, Digital Markets Act and Digital Services Act, took the EU many years and, in some cases, decades to develop and enact. Stalla-Bourdillon said a lack of consensus among lawmakers and intense lobbying by AI vendors can also slow the regulatory process. "Every piece of legislation is a political compromise," she said, "and politics takes time."
The AI Act moved much more quickly, but Moore believes a faster pace could sacrifice sufficient deployment details and specificity. "Until and even after the authorities provide implementation details," he conjectured, "industry practitioners will want to work with their advisors to help assess the law's implications."
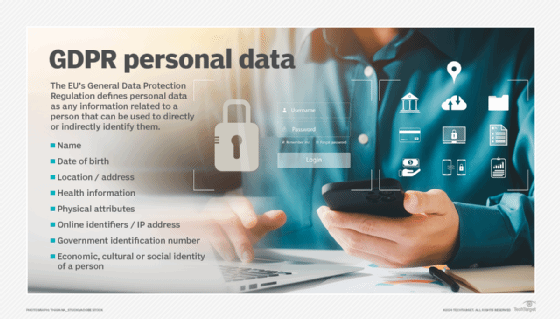
How will GDPR be affected by AI?
The GDPR established national data protection authorities and European-wide bodies, such as the EDPS and EDPB. These bodies are likely to issue guidance to help citizens and enterprises understand AI and the various laws governing it. They might also enforce AI practitioners' behavior, either alone or with other regulatory bodies, and use AI to manage enterprise data protection activities, respond to citizen inquiries and conduct investigations.
In addition, Moore said GDPR provisions can influence the development and deployment of AI in several ways, including the following:
- Increased scrutiny of data protection procedures. AI systems consume and analyze an incredible amount of data to train and operate effectively. As a result, organizations developing or deploying AI must pay even greater attention to data protection principles and practices outlined in the GDPR, such as data minimization, purpose limitation and storage limitation.
- Stronger transparency requirements. The GDPR requires organizations to provide individuals with clear and concise information about how their personal data is processed. For AI systems, that could include explaining the logic behind automated decision-making and providing meaningful information about the consequences of such processing. Given the complexity of AI systems, meeting these transparency requirements can be challenging.
- Focus on data quality and accuracy. AI systems are only as good as the data they're trained on. The GDPR's data accuracy and quality principles become even more critical in the context of AI, because biased or inaccurate data can lead to discriminatory or unfair outcomes. Enterprises must ensure the data used to train AI models is accurate, relevant and representative.
- Greater need for human oversight. The GDPR protects individuals from being solely subjected to automated decisions and profiling, implying the need for human oversight and intervention in certain AI use cases and forcing organizations to rethink their AI governance structures.
- More complex data protection impact assessments. The GDPR requires organizations to conduct DPIAs when processing is likely to result in a high risk to an individual's rights and freedoms. Given the potential risks associated with AI, such as privacy intrusion, bias and discrimination, DPIAs could become more common and more complex for AI projects.
- Emphasis on privacy by design and default. The GDPR's principles of privacy by design and default require businesses to integrate data protection safeguards into their systems and processes from the outset. For AI systems, techniques that enable data analysis and model training while preserving individual privacy might be affected, such as federated learning, differential privacy or secure multiparty computation.
- Liability and accountability challenges. AI systems can involve complex supply chains and multiple parties, making it difficult to determine responsibility and liability when things go wrong. The GDPR's provisions on data controller and processor responsibilities might need to be adapted to better capture AI development and deployment efforts.
Regulating AI in the future
"The future of AI and regulations in Europe," Moore conjectured, "is likely to have a significant impact on the industry globally as the GDPR did upon its introduction." The AI Act, for example, could become a global benchmark for AI governance, influencing regulations in other jurisdictions and shaping industry practices worldwide.
However, the AI Act includes numerous exceptions, which risk undermining its whole purpose, Stalla-Bourdillon argued. It delegates standards-setting for new metrics to various bodies, which will depend on their work and the oversight performed by auditors. Standards and auditing, for example, typically focus only on processes rather than substance to protect privacy.
The rapid adoption of AI will require building trust rather than just faster models, Inrupt's Ottenheimer cautioned. "AI development accelerates when it's made measurably safer, like how a fast car needs quality brakes and suspension," he explained. "It fosters public trust and enhances competitiveness." With the emphasis on safe AI in the AI Act, he added, "Europe now serves as a global model for ethical practices, shaping the future of the industry in tangible societal benefits and establishing important benchmarks for individual freedom and progress."
George Lawton is a journalist based in London. Over the last 30 years, he has written more than 3,000 stories about computers, communications, knowledge management, business, health and other areas that interest him.