What is data lifecycle?
A data lifecycle is the sequence of stages that a unit of data goes through from its initial generation or capture to its archiving or deletion at the end of its useful life. Data lifecycles entail any processes and tools organizations use for data creation, preparation, management, storage and security.
These processes also include understanding which data is confidential or private. Critical data is susceptible to data breaches and increasingly sophisticated cyberattacks. As a result, regulatory agencies as well as many companies have developed compliance frameworks to protect data.
Data lifecycle is an evolving concept. It was once simple to manage data lifecycle processes. However, now that organizations regularly generate terabytes of data and artificial intelligence (AI) is being incorporated into these platforms, these initiatives have become broader and more complex.
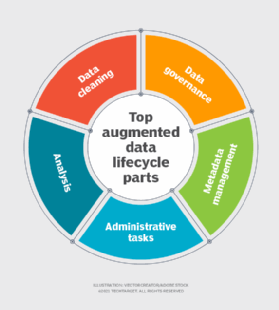
Data lifecycle stages
Although specifics vary, data management strategy experts identify six or more stages of the data lifecycle, as noted in the following example:
- Determine the need. Before generating new data or capturing existing data, organizations must ensure that the need for such data is established and confirmed. This step is important in minimizing data collection -- also called data minimization -- so that only necessary data is collected to avoid redundancy.
- Generation or capture. In this phase, data comes into an organization, usually through data entry, acquisition from an external source or signal reception, such as transmitted sensor data.
- Data preparation for use. In this phase, data is processed and prepared for use. The data processing can include scrubbing and integration, as well as extracting, loading and transforming. Also, data encryption prevents unauthorized access to sensitive information and personal data.
- Active use. In this phase, data is used to support the organization's objectives, operations and decision-making processes.
- Data management. During the management phase, data might be made public if appropriate, provided to stakeholders through data sharing or retained internally. Organizations can store data on a short-term storage platform for continued availability while security and privacy are constantly maintained.
- Long-term storage, retention or archiving. In this phase, data usage and data retention policies determine when data is moved from active production environments into an archive. At this point, it's no longer processed, used or published, instead, it's stored should it be needed in the future for legal or audit reviews or other purposes. The data might be stored internally on hard disk drives, solid-state drives or tape or with a cloud storage service.
- Purging and destruction. When the data becomes obsolete, every copy is deleted and destroyed as part of the removal process. The data destruction process might include the media on which the data resides.
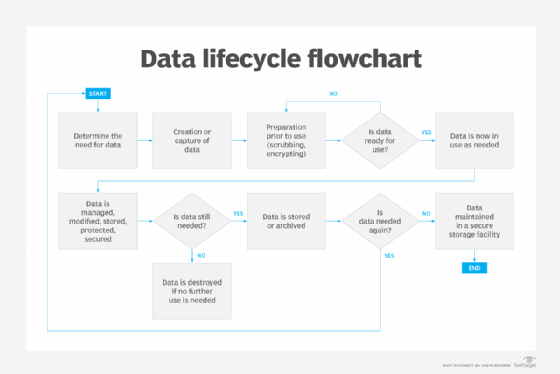
Why is data lifecycle management important?
Data lifecycle management (DLM) is getting more attention for a number of reasons:
- Large data volumes. An ever-increasing number of devices is generating enormous volumes of data. Proper oversight of all this data throughout its lifecycle is essential to streamline data handling processes, optimize its usefulness and minimize the potential for errors.
- Analytics. Big data analytics has become mainstream along with the internet of things, which generates the big data that needs to be analyzed. Today, data analysis is more easily done on large data volumes with the help of AI and machine learning tools.
- Organizational efficiency. Emerging automation and real-time functionality are making DLM processes more efficient. In addition, data archiving or deletion at the end of its useful life ensures that data stores don't consume more resources than necessary.
- Security and privacy. DLM ensures data security and privacy goals are met. These are collectively known as the CIA triad. One part of the triad is data confidentiality, which ensures sensitive data is stored in secure environments where unauthorized individuals can't access it. Data integrity is the second part of the triad, ensuring data can't be altered or corrupted. Data availability is the third part; it ensures that data access is available only to individuals or entities with the appropriate permissions.
- Compliance. DLM is essential for establishing and maintaining compliance with key data security and privacy legislation, such as the General Data Protection Regulation, Sarbanes-Oxley Act and Health Insurance Portability and Accountability Act.
- Data governance. DLM is a key element in the efficient functioning of data governance processes that oversee how organizations secure and use data.
Data lifecycle management vs. information lifecycle management
DLM and information lifecycle management (ILM) go hand in hand but have a key difference. Data lifecycle management is concerned with processes for managing data sets or files and knowing which ones to keep or discard. ILM is about examining files more closely to see if specific information is relevant and up to date. ILM is more granular than DLM.
This article is part of
What is data protection and why is it important?
DLM is concerned with factors, such as formats, sizes and types of data files; these aren't relevant to ILM. However, the two concepts aren't mutually exclusive. Both practices contribute to ensuring data quality, as DLM policies can be complemented with ILM efforts. These efforts might involve employees closely examining information and metadata in files.
Achieving compliance with data lifecycle management requirements
Data privacy and other standards regarding data security, such as ISO 27001, require businesses demonstrate compliance with data lifecycle controls. To show compliance, especially in preparation for or as part of an audit, organizations might have to do some of the following:
- Ensure data lifecycle management policies are up to date and implemented correctly.
- Examine data management procedures to ensure they're up to date and accurately reflect the state of data management in the organization.
- Generate copies of management reports that provide evidence of how an organization's data is managed, stored, archived and destroyed.
- Prepare evidence of how and where each piece of data is stored and archived, such as in cloud databases. Also, provide information on how data is secured and destroyed or secure data. This can be done with internal data management applications or third-party apps.
For an organization to conduct data lifecycle management properly, an effective data management team must be assembled that understands the data-driven world. Learn about the key roles needed for a data management team.