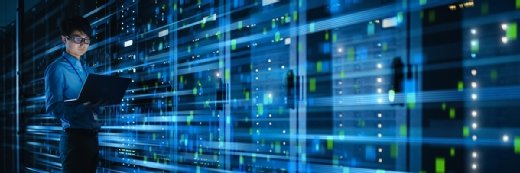
Gorodenkoff - stock.adobe.com
Nvidia partners, customers drive AI into data centers
Nvidia and its partners are providing the tools and infrastructure to build and deploy AI applications that companies say could transform their businesses.
SAN JOSE, Calif. -- Nvidia has won the business of the largest cloud providers with powerful GPUs to run their AI models and services. It's now heading downstream with a broad toolset and partner army focused on the enterprise data center.
This week, Nvidia GTC, the company's annual developer conference, attracted thousands of data scientists and electrical and computer engineers hoping to learn how to build, deploy and manage software unique to AI. Joining the technologists were customers and partners making deals and promoting an industry that analysts say will transform business.
Today's data centers of CPUs powering the servers that run business software will need to make room for infrastructure unique to generative AI (GenAI) models. Deploying and running the GenAI models will require new toolsets.
"General purpose computing has run out of steam," Nvidia CEO Jensen Huang said during his opening keynote here this week. "We need another way of doing computing."
Huang unveiled version 5 of the company's AI Enterprise platform with new technology that the executive described as a Nvidia inference microservice (NIM). Together, the combined software simplifies the process of creating and developing GenAI applications that leverage Nvidia's CUDA, or Compute Unified Device Architecture, parallel computing platform and programming model for the company's GPUs.
Analysts expect many enterprises to deploy small language models in-house so they can fine-tune them on corporate data without moving sensitive information to a public cloud. Running a model in the data center can sometimes be less expensive than the cloud.
Nvidia partners target enterprises
The NIM is helpful because it simplifies the process of regularly feeding real-world data to a trained model so it can make up-to-date responses -- a process called inference. Having tools that automate processes related to models means traditional software engineers can do the job instead of hard-to-find AI experts, said Robin Bordoli, chief marketing officer at Weights & Biases, an AI model-training platform maker.
Weights & Biases has integrated its software with Nvidia's inference engine so developers can do training and inferencing from a platform supporting 30 foundation models. Today, Weights & Biases has 1,000 customers, many of whom are government agencies and life sciences organizations, Bordoli said.
"We're helping the next set of customers, enterprises," he said. "They're never going to build a model from scratch, but they want to take an existing model and fine-tune it on their enterprise data."
Nvidia has built NIM to run as a container on Kubernetes, an open-source container orchestration platform familiar to enterprises, said Patrick McFadin, vice president of developer relations at DataStax, a provider of vector databases for AI applications.
"What I noticed right off the bat is it's deployed using Kubernetes," McFadin said. "People who run infrastructure at large enterprises are using Kubernetes, so they've plugged themselves into that really nicely."
Nvidia partner Dell Technologies offers a variety of PowerEdge servers with Nvidia's AI Enterprise software and GPUs, the H100 and the L40S.
"What we're seeing from the majority of enterprises is to take off-the-shelf models, whether it's large models or small models, and combine them with proprietary enterprise data," said Varun Chhabra, senior vice president of infrastructure and telecom marketing at Dell.
Dell believes retrieval-augmented generation (RAG) will be as important as inferencing within enterprises. RAG is an architecture that incorporates an information retrieval system to secure private data.
"RAG is a big area of focus for us," Chhabra said.
The most significant benefit of Nvidia NIMs is packaging many of the microservices needed for inferencing in a single container, Chhabra said. "It does that in a turnkey fashion."
AI software and the accelerated computing needed to run it is changing the data center, Chhabra said.
"It definitely feels like we're at an inflection point," he said. "That complete rebuild of the data center is coming."
Customers at Nvidia GTC
At the conference, Nvidia customers described how they work with GenAI, all of it at the early stages. The companies included LinkedIn; global advertising company WPP; cosmetics maker L'Oreál; and ControlExpert, GmbH, a German claims management software maker.
WPP partnered with Nvidia to develop a content engine for creating videos, 3D and 2D images of clients' products using Nvidia Omniverse Cloud, and GenAI. The system also uses photos from Getty Images and Adobe's content creation technology.
The quality of the advertising art depends on the data available to the AI models producing the works, said WPP CTO Stephan Pretorius.
"We find that in the cases where we work with clients that have a very, very crisp brand definition, a very accurate way of describing the brand's personality, tone, voice, et cetera, we get much better results than when that is diffuse," he said during a presentation.
Essentially, WPP uses AI to mimic the "human content creation process," Pretorius said. "But across the complexity and the scale and the amount of data that we have to work with, you can't execute things like this without AI."
Pretorius believes AI-powered voice communications with website visitors will eventually replace today's content-driven approach.
"We believe the future of content consumption will be largely conversational," he said.
L'Oreál is testing GenAI to produce images for storyboarding and Nvidia Omniverse for 3D renderings of the company's packaged products. It also uses several AI models.
The company feeds its models with thousands of brand images as well as background colors, different types of lighting and settings such as an elegant Parisian sunset. Ad creators can use natural language to have a model create images to stimulate ideas for marketing its 37 global brands.
The system can help in imagining scenarios such as the beauty salon of the future or ads using a space phenomenon such as the Red Nebula.
"There is a reinterpretation of data that happens," Asmita Dubey, chief digital and marketing officer at L'Oréal, said of GenAI in an interview. "And it's the speed [of creation]. It can do it faster.
Over the last six months, L'Oréal has worked with Nvidia Omniverse and WPP to create custom 3D models for its products so it can change backgrounds, color and shading without having to spend days in a studio with a photographer. L'Oréal needs only one studio session to capture all angles of the product packaging.
The company believes it can save time and money, but it is still in the early stages of using Omniverse.
In a panel discussion at the conference, Sabry Tozin, vice president of engineering at LinkedIn, said the company uses AI for language translation. This lets a customer service rep in Omaha, Nebraska, speak to customers in Spanish, French or German without knowing the languages.
"What this allows us to do is actually retain reps that are very good at understanding our products and giving in-depth answers to our customers," Tozin said.
ControlExpert's software makes it possible for insurance companies to have customers take pictures of car damage following an accident and send the images to the vendor through a mobile app.
AI models analyze the image, assess the damage and return estimated repair costs and a list of approved auto body shops. ConrolExpert customers include 90% of all insurance firms, according to the company.
The company trained its models on data collected over 20 years. It processes 20 million claims a year, according to Sebastian Schoenen, the company's director of innovation and technology.
Car model design changes occur regularly, and repair prices also fluctuate, so ControlExpert is constantly updating its models. Nevertheless, there is still a small fraction of cases that require human intervention.
"If we see that our models are not capable of doing things, we steer the claim to humans," Schoenen said.
Antone Gonsalves is an editor at large for TechTarget Editorial, reporting on industry trends critical to enterprise tech buyers. He has worked in tech journalism for 25 years and is based in San Francisco.