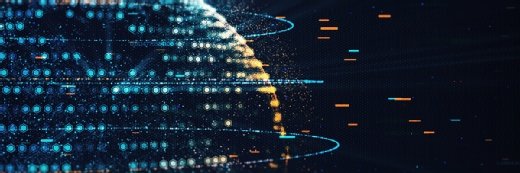
Getty Images/iStockphoto
The pros and cons of big data outsourcing
More companies are seeking outside help to capitalize on data's value. Examine the benefits and drawbacks that come with outsourcing big data processing projects.
There are several reasons enterprises outsource some, or even all, elements of their big data projects. Although many executives get excited about the AI capabilities of outsourcing big data efforts, it is important to recognize much data management grunt work is involved in making these projects successful.
By some estimates, enterprises spend five times as much time on data engineering -- preparation, cleaning, collection and transformation -- as they do on data science. Big data outsourcing can help kickstart these initiatives because teams have more resources to tap into to conduct data science. Outsourcing can also lay the foundation for more sustainable and repeatable data science results.
"If data is the new oil, the refinery is the large data technology system and processes," said Amaresh Tripathy, global business leader of analytics at Genpact, a digital transformation professional services firm.
Why outsource big data projects
As the data value chain matures, companies are focusing more on insight development while increasingly relying on partners to drive the refinement of data.
There are economies of scale and expertise that partners can bring, along with mature development processes and a global scale that help manage cost. For example, a large cloud data migration at a regional bank helps manage the long-term cost of data infrastructure ownership. According to Tripathy, partnering with service providers also adds speed to the data value chain as enterprises become more data hungry. Companies can focus on providing added value to their data scientist teams while taking advantage of provider services that help manage other data projects.
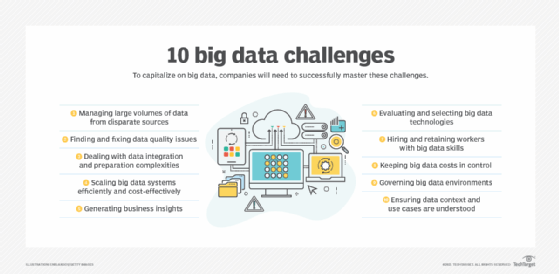
Peter Mottram, managing director and global enterprise data and analytics leader at Protiviti, said the top reasons enterprises outsource big data projects can include the following:
- not enough subject-matter experts on legacy systems at the company that know the data;
- new technologies and skills making resources hard to find or manage;
- cost savings from offshore or nearshore outsourcing;
- adopting new delivery and support models to reduce costs; and
- consulting firms providing variable resource pools for projects so the enterprise doesn't need to hire new staff.
Big data outsourcing pros
Scaling for complex data processes
Many aspects of larger big data projects require considerably more manual work to address lineage, metadata and quality.
"A benefit to using a third party is being able to ramp up resources for the push in the project and then ramp down after the data issues are addressed," Mottram said. "It's also important to make sure to automate and put controls on the processes along the way."
Keeping pace with disruption
Many enterprises are quickly moving data infrastructure to the cloud in response to COVID-19 and new work-from-home requirements. In addition, there are often big jumps in automation usage in the wake of economic recessions, according to Mike O'Malley, senior vice president at the IT outsourcing firm SenecaGlobal.
While businesses want to invest in automation, there is currently a global shortage of experts in big data engineering and cloud-native technologies to support these initiatives.
"More organizations are turning to outsourcers that can offer the exact data science/big data expertise with cloud-native development experience that is required," O'Malley said.
Identify bottlenecks
Big data projects require significant investments and change management. Some of these investments are complex decisions, given the number of tools available in the market and the rapid pace of innovation. Change management involves strategic, technological and operational changes that need to be carefully considered. The knowledge of these outsourcing partners regarding where a big data migration or greenfield program might run into potential bottlenecks can help mitigate risks early.
"Strategic outsourcing partners in this space have, over many big data implementations across their clientele, accumulated necessary war scars to help organizations navigate these investment decisions as well as carefully consider all change management efforts required," said Sandhya Balakrishnan, region head of data analytics and engineering at Brillio.
Keeping the swamps at bay
Many organizations have also burned their fingers by embarking on big data programs that failed to ensure users have high-quality data and can easily discover data. Such data swamps can be avoided by thoughtful, architectural decisions upfront in the program. By providing industry best practices and ways to combine optimized technologies, big data outsourcing providers help clientele ensure their investments demonstrate high ROI, Balakrishnan said.
Automate data hygiene best practices
Business decisions made on data-driven insights are only as accurate as the underlying data. Big data outsourcing providers often have expertise in applying industry-standard methodologies to clean the data within the context of industry and domain applications. They also have more familiarity with the appropriate technology stack to further automate the intense manual data cleaning and standardization processes.
Consequentially, the development of a clean data foundation layer becomes less person-oriented and more process-oriented, said Tripathy. For example, Genpact is improving the cash flow forecasting of a large beauty company that relied on data from a dozen separate ERP systems.
Big data outsourcing cons
Loss of knowledge
Enterprises need to consider how to retain the talent and knowledge of their systems over time. They need to deploy new ways of working and leverage partners to help deploy this organizational change.
"If they only rely on outsourcing, they could become hostages to the outsourcing firm going forward," Mottram said.
Ensuring appropriate domain expertise
There is a wide range of big data outsourcing firms. It is important to find one with the appropriate expertise in a specific vertical and data processing tool sets. Just because a firm has mastered data science, it does not guarantee they know how to clean your data efficiently. Data reflects business processes, but companies often outsource it to pure-play technology companies that may not have the proper context of the business, said Tripathy. This often leads to issues with data quality.
Lack of proper context
Another drawback is the lack of a talent pipeline. Many data scientists cut their teeth in data management, which helps them understand the nuances of a business. If the company exclusively relies on partners for data projects, it could create a talent gap for more sophisticated business analysis over time, Tripathy said. There are ways to mitigate for this risk, but companies must plan for them proactively.
New security and privacy issues
Another critical concern is around data security and privacy with external vendor partners having potentially sensitive access, said Balakrishnan. It's important to work with the data privacy officer to ensure that data sharing does not violate any of the newer privacy regulations, which may be less understood by the vendor.