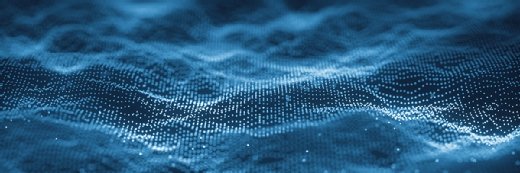
Nabugu - stock.adobe.com
Data mesh aids democratization with decentralization
Real-time analytics enables faster decision-making and insights. As data democratization rises in importance, data mesh helps decentralize that data for all users.
Decision-makers try to capture, filter and sort business data rapidly, without compromising the quality of the data to get useable results. To put data into the hands of those who need it most, organizations decentralize approaches and democratize data.
How data gets sorted and arranged depends on the architectural design. Simply put, these architectural designs are centralized or decentralized. Commonly, data architectures are a centralized function. A decentralized architecture assigns critical data decision-making to the domain teams and users, empowering employees to ask and work with data-related questions.
Among data architectural designs and tools, data mesh is an attractive decentralized option to enable employees to work with accessible and secure data, and facilitate the democratization of data.
This article explores how data mesh aids the democratization of data and the value it brings to an organization. Learn from examples of how companies apply data mesh today.
Data mesh and democratization
Data mesh operates on the twin principles of domain-driven design and product thinking. By transferring responsibility from a central data team to the domain team, data mesh gives the latter greater control and autonomy. This enables faster decision-making, easier data discovery and accessibility, greater interoperability, scalability, security, compliance and even better data quality.
Data democratization is an ongoing process wherein everyone in an organization -- regardless of their technical know-how -- can work with data comfortably and feel confident about it. This leads to data-informed decisions and customer experiences built by data.
As both the creators and consumers of their data, domain teams capture, filter and sort relevant data, breaking down data silos. This sorting and filtering of relevant data helps prioritize valuable information and promote collaboration within and across domains. Data becomes more than a slice of information; it is relevant within and across multiple domains. The utility and usefulness of the data is driven by the domain owner with the end user in mind. Data is much more than an asset; it is treated as a product, with federated governance, speed and agility.
Value from democratization
The value of data mesh and the democratization of data rests on key tenets: cultivating trust, usability and speed to accessibility, interoperability, scalability and insight. Critical elements of data mesh involve domain ownership, data as a product, self-service infrastructure and federated data governance.
Domain ownership. Domain owners are accountable for their data. They manage, store, sort, classify and share their data. Data sharing is based on relevancy and usefulness to the prospective user. The value of domain ownership is accessibility, breaking down barriers between domains without distancing data from the domain owner.
When a domain owner uses a piece of data to gain insights, such as a data table, report, dashboard, etc., it becomes a data asset. Any of these types of data assets could also be a standalone product, providing value to a client or decision-maker by answering a specific question.
Data as a product. The difference between a data asset and data as a product is product thinking: identifying what makes something useful to a client or decision-maker based on their needs. To classify what is useful involves certain types of data characteristics. To capture user needs, apply product thinking to data and include questions that describe the characteristics of data: Is data accessible, trustworthy, secure, interoperable and comprehensible?
These characteristics apply to data code, data policies, metadata and so on. While data as an asset primarily speaks to how to gain insights, data as a product goes beyond insights to embed these characteristics in multiple data components with an understanding of how, what and where the client could potentially use it. The key value of data as a product is prioritizing valuable information so that users can get the right data faster.
Self-service data platforms. As owners of their data, domain teams typically maintain full autonomy and manage their data products. This requires a self-service infrastructure that removes all complexities of data product lifecycle management. The value of self-service data platforms is collaboration, while prioritizing critical information. During collaboration with cross-functional teams, domain owners share relevant data.
Federated data governance. A key pitfall of distributed or decentralized domain data ownership is duplication of effort. Without centralized control, individuals can create data silos and interoperability issues across domains. A federated data governance model with a common data language -- definitions, terms, standards, policies and metadata -- is critical to data mesh. It enables the democratization of data in the data mesh. Domain teams follow a set of rules and retain their autonomy.
Real-life applications
Several industries use data mesh to democratize data and empower employees. These examples highlight data mesh in different industries.
Banking modernization. A global banking company was in the process of modernizing its platform, going from on-premises to the cloud.
The goals were as follows:
- Have an enterprise-wide well-defined cloud and data strategy.
- Reduce costs.
- Reuse the data while unlocking new data opportunities.
Using data mesh architecture, each domain owner created data products that were interconnected across several business domains. Employees could provide accurate data and update data within each domain thanks to the standardized data definitions, terms and policies, and metadata management to track data lineage and provenance. In gaining the trust of internal employees, and eventually external clients, the bank increased its data use, leading to a data literacy program.
IT data overhaul. A global IT company sought to empower its employees to create and own high-quality data-driven systems. The goal was smarter product experiences.
The data division within the company experienced issues around data discovery, trust and access and use of relevant data. Data division employees, in collaboration with other workers, developed a set of internal processes, data products and consumable data across several domains with data mesh architecture. This resolved several issues related to roles, authorship, documentation, duplication, quality, governance and management of data. As employees across the board gained trust in accessing accurate and useful data, they became data enthusiasts -- even some data champions.
Data mesh is a paradigm shift of how to view, manage and experience data. It enables the democratization of data without disrupting the workforce or daily operation. When thinking of data mesh as an architectural design or framework, an organization must define its data needs, use, maturity and culture. Setting up a decentralized ecosystem demands resources, time and effort with myriad domain teams. It demands continuous quality improvement, with attention to how, what and where data resides. It demands oversight of standardized data governance.