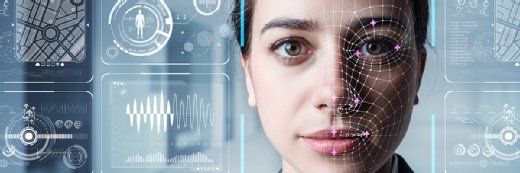
metamorworks - stock.adobe.com
AI bias, for good or ill
Bias in AI algorithms can produce harmful results, but it can also help train models. This third part of a three-part series on AI ethics explains the pros and cons of AI bias.
In 2017, Amazon quietly shelved an AI-powered job recruiting platform it had been developing for the past three years. The software, it was later revealed, contained bias against female job applicants -- a problem Amazon noticed two years earlier but had been unable to fix.
The problem was in the decade's worth of job application data Amazon had used to train its AI algorithms. The data reflected a disproportionate number of male versus female hires among tech companies, and the algorithms simply took in the data and produced algorithms that tried to produce that same pattern.
A similar controversy involving potentially harmful bias in AI algorithms erupted earlier this year when activist shareholders and then a group of AI scientists objected to Amazon selling its Rekognition object and facial recognition software to U.S. government agencies.
For its part, Amazon, facing a major backlash for possible bias in the Rekognition platform, responded with blog posts on its AWS site.
Matt Wood, general manager of deep learning and AI at AWS, commented in one post on what he characterized as "several misconceptions and inaccuracies" in the research papers and articles that helped fuel criticism. He said results might have been skewed due to using the tools improperly and with confidence levels that are different from the recommended ones.
A pervasive problem
Harmful bias is a common problem for AI and tech businesses, even if they don't always notice or deal with it.
It's a part of today's "fix it later" culture, said Joseph Hellerstein, chief strategy officer and co-founder at Trifacta, a data wrangling and data prep software vendor.
Organizations will "undoubtedly see a lot of (bias)," he said.
It can be easy to miss data and AI bias "if you don't have eyeballs on data and constant checks going on," Hellerstein, who is also a professor of computer science at the University of California, Berkeley, said.
Machine learning and AI algorithms often exist in a black box, meaning they are difficult to dig into and see where something might have gone wrong. AI users, and sometimes AI researchers, may be unable to quickly determine why an AI system acted in a particular way.
Often, too, Hellerstein continued, it can be difficult to track the chain of data custody -- where data came from and how it was obtained, and later modified -- making it hard for businesses to determine just how biased the data they are using for AI algorithms is.
Even if an organization does detect data or AI bias in its work, the fast pace at which many modern companies operate might push that work out with the promise or intention of a later fix.
"Getting that culture to be more cautious about bias is tricky," even if it's necessary, Hellerstein said.
Harmful bias
Besides Amazon, other high-profile tech vendors have come under criticism over the last few years for using biased data and creating biased AI algorithms. Earlier this year, the U.S. Department of Housing and Urban Development sued Facebook after the agency found Facebook allowed advertisers on its platform to target audiences based on characteristics such as religion, gender and race.
The suit came only a week after Facebook, facing numerous complaints, agreed to overhaul its advertising system.
Around the same time, researchers claimed in a scientific paper published by Cornell University that Facebook's internal algorithms for advertising are themselves biased and skew the tech giant's ad delivery.
"We're surprised by HUD's decision, as we've been working with them to address their concerns and have taken significant steps to prevent ads discrimination," Facebook said in March 28 statements emailed to media outlets.
Facebook and Amazon did not respond to SearchEnterpriseAI's requests for comment for this story.
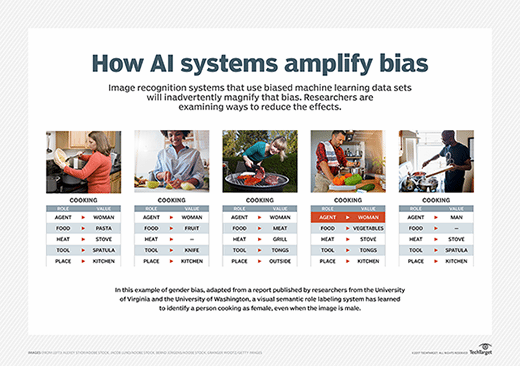
What to do
For companies that have bias in their AI system, whether it's from biased data or biased algorithmic programming, using that system is the "equivalent of shooting yourself in the foot with a machine gun," said Stan Christiaens, chief technology officer and co-founder of Collibra, a vendor of data governance software.
A biased algorithm produces large amounts of errors and biased data quickly, he explained, and since most algorithms are in black boxes, companies might not even notice how severe the damage is until it's too late.
Christiaens urged AI tech companies to use caution, and suggested several methods of limiting bias, such as consulting with ethics committees, hiring data science teams made up of people with varied personal and work-related backgrounds, or working with a third-party data governance vendor.
However, like Hellerstein, he said constant vigilance and moving to adjust a potential problem quickly could significantly help businesses prevent or eliminate bias.
But even while AI bias can skew data and results unfavorably, sometimes introducing bias into an AI algorithm can help train it.
Not always bad
Indeed, for some training applications, introducing bias can help produce better results, said Chris Hazard, chief technology officer at Diveplane, a start-up focused on creating understandable and explainable AI systems.
Take self-driving cars, for example. If a car trains on common driving data, it might be able to drive in normal weather conditions skillfully. However, Hazard noted, the training data probably wouldn't contain many instances of abnormal weather conditions, such as driving in high winds or extremely heavy rain or snow, and the car would be unable to operate optimally in those conditions.
So, "it's important to introduce bias in the model," Hazard said, including extra sets of driving data from snow and abnormal weather.
Just as bias can hurt a model and its produced results, it can help it. Either way, transparency is critical. The European Commission released a set of guidelines highlighting the importance of transparency, accountability, explainability and fairness for AI systems. The commission also accentuated the need to remove bias in data before using it to train algorithms.
"In order to achieve trustworthy AI, we must enable inclusion and diversity throughout the entire AI system's life cycle," the AI guidelines state.
Participating organizations will follow the guidelines during the summer of 2019 in a test phase. The data that is collected will be reviewed in 2020 as the European Commission decides next steps.