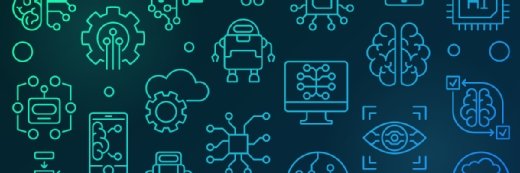
Intersection of generative AI, cybersecurity and digital trust
The popularity of generative AI has skyrocketed in recent months. Its benefits, however, are being met with cybersecurity, digital trust and legal challenges.
The tagline of the comedy show Whose Line is it Anyway? is: "It's the show where everything's made up and the points don't matter."
In the context of generative AI, all that is made up from it could matter and could have potentially serious implications -- to the extent that top AI executives have likened the risk of extinction from AI to be on par with risks posed by pandemics and nuclear war.
Generative AI uses machine learning techniques, such as deep neural networks, to enable users to quickly generate new content based on inputs that include images, text and sound. The output from generative AI models is highly realistic, ranging from images and videos to text and audio. The output is so realistic that an attacker used AI-generated voice files to successfully impersonate a CEO's voice to illegally access bank account information.
Generative AI content has gained immense popularity in recent years, and its use is proliferating. Concerns about its legal implications and associated cybersecurity risks are also emerging. Progress has been made, but there's a long way to go in addressing even the typical generative AI risks, such as hallucination and biases. After all, the lifeblood of generative AI systems is data, and parts of the data sets fed to train models can inadvertently determine some output, which may include perpetuation of stereotypes or reinforcement of discriminative views.
Let's look at the intersection of generative AI content, cybersecurity and digital trust, and explore the legal challenges and risks involved, as well as some key takeaways for consideration.
Legal implications of generative AI content
One of the primary legal concerns of content created from generative AI is related to intellectual property rights. As seen from the complexities faced in the data privacy space -- and because laws and interpretations could vary across regions and jurisdictions -- organizations must carefully consider the intellectual property rights tied to AI-generated content. Margaret Esquenet, partner with Finnegan, Henderson, Farabow, Garrett & Dunner LLP, told Forbes that, for a work to have copyright protection under current U.S. law, it "must be the result of original and creative authorship by a human author. Absent human creative input, a work is not entitled to copyright protection. As a result, the U.S. Copyright Office will not register a work that was created by an autonomous artificial intelligence tool." Note, however, that such law may not be in effect in other jurisdictions.
Another factor that could influence the ownership and liability implications of AI-generated output is the input. Generative Ais, such as large language models, work best given a context or prompt. The more quality input and context given, the better the output generated is. For some systems -- namely those from a service provider -- organizations need to be cautious about sharing any input that might be proprietary information. On the flip side, from a legal perspective, organizations that build these systems currently are not required to declare the data used for their model training. Some controls need to be in place to protect intellectual property. Determining the legality of AI-generated content becomes complex, particularly in cases involving fair use and transformative works.
Another determining factor on the ownership and liability implications would be on the output generated. Generative AIs became popular with the success of ChatGPT's adoption beginning in late 2022. Before then, technical knowledge required to build such systems meant only large companies could develop and run generative AI models. Now, with more APIs available, it is easy to get on board -- all it takes is to connect to a pre-trained generative AI model via an API and users can develop a new model over the course of several weekends.
As major cloud providers, such as Google and Microsoft, roll out AI- and machine learning-specific services, new models can be trained quickly for little to no cost. The open source nature of these services enables people without programming knowledge to download a desktop client, such as Stable Diffusion, and start creating images without the controls or safety features of an OpenAI product.
So, who should be the rightful owner and be responsible for the output content created through generative AI algorithms? These systems, while highly efficient in producing output content, could infringe existing copyrights, raising questions about ownership and attribution. For example, if a developer uses an AI-powered coding assistant, such as GitHub Copilot, to generate an application's source code, would the developer and the organization become the owner of that application and its data set? A lawsuit brought against GitHub and Microsoft in November 2022 put the spotlight on the legality of generative AI systems, including how they are trained and how they reproduce copyrighted material.
The relation between copyright legislation and AI is still not being fully considered. This could also be the reason several prominent AI researchers signed a call to pause giant AI experiments.
To address legal concerns, regulation and legislation enforcement need to come into play. The European Commission's AI Act, which goes into full effect in the next year or two, requires generative AI systems to provide more transparency about the content they create. The aim is to prevent any illegal content from being generated and to disclose any copyrighted data used.
The 10-member Association of Southeast Asian Nations agreed to develop an ASEAN Guide on AI Governance and Ethics by 2024, though it should be noted that the guide will focus on addressing AI's use in creating online misinformation.
In the long run, given the global reach of most generative AI systems, we need an international regulatory framework for AI that promotes consistency and inclusivity in the development of the models. This is a tall task, however, because each regulation needs to be customized to suit the local needs of the country or region.
Existing laws and regulations struggle to keep pace with the rapid advancements in generative AI technology. While some legal frameworks touch upon intellectual property rights and privacy concerns, they have notable gaps and limitations in addressing the specific issues posed by generative AI content. Furthermore, enforcing these laws is challenging because it's difficult to identify the origin of AI-generated content and jurisdictional complexities.
Implications of generative AI on digital trust
It could arguably blur the lines to implicate any human actor for any losses or damages caused by misinformation spread online or any malware or cyber attacks generated by AI systems that did not have sufficient safety controls in place. Generative AI content can be used for fraudulent purposes, including creating counterfeit products or manipulating financial markets and public opinion. These pose legal risks related to fraud and can have far-reaching consequences for businesses and society.
The potential compromise of digital identity and authentication systems also raises data security and privacy concerns. For example, biometric security systems could face new threat levels due to generative AI's ability to replicate images in formats that could be used to unlock systems. Would cyber insurance cover losses due to attacks generated by deepfake attacks?
Addressing the legal implications and cybersecurity risks associated with generative AI content requires a multifaceted approach. Besides regulation, technology can help establish the authenticity and origin of generative AI content -- for example, AI algorithms for content verification and digital watermarking. Enhanced cybersecurity measures can also be used to safeguard AI systems from exploitation and prevent unauthorized access.
Generative AI and risk management
Before legislation and regulatory frameworks can fully be enforced, organizations should consider other guardrails. A guidance framework, such as the NIST AI Risk Management Framework (RMF), can help promote a common language across development of all generative AI systems and demonstrate the commitment from the system owners to deploy ethical and safe generative AI systems.
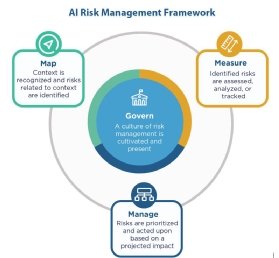
The Govern function of the AI RMF relies on commitment of people, including the senior management team, to cultivate a culture of risk management into the full AI product lifecycle. This includes addressing legal issues around using third-party software or hardware and data.
Responsible leaders of generative AI systems should designate personnel to curate data sets to ensure more diversified perspectives. Commitment from big corporations promoting generative AI products is lacking, however. For example, Microsoft laid off its ethical AI team early this year.
The Map function of the framework establishes context to identify risks related to an AI system. Risks could include reputational damage that may arise due to misuse of the AI systems and that would jeopardize the digital trust of organizations.
The Measure and Map functions require organizations to identify sufficient metrics to demonstrate that AI systems have been tested from all possible angles and mapped to the risks and context of the AI systems. These promote transparency and increase confidence of a commitment from senior management to provide resources to put in place strategies and controls that maximize the benefits from AI systems, while minimizing the risks.
Organizations must act with urgency
Generative AI content has revolutionized the digital landscape. It is accompanied by legal implications and cybersecurity risks, however. The legality and liability challenges from use of generative AI's content is arguably the reason why an increasing number of countries are rushing to draw up guardrails in the form of regulations to govern generative AI use.
Based on the current landscape, however, most countries are moving at a slow pace to draw up such legislation. Some are only starting the journey, while others have in place a mix of voluntary codes of practice or industry-specific rules, which are not sufficient given the potential damage that could result due to violations of intellectual property rights, misuse of AI systems to perform major cybersecurity attacks and other unprecedented adversarial uses.
Similar to how regulations and compliance requirements were considered, drafted and passed in industries such as banking, AI regulations will see delays as well. It could take years. In the meantime, organizations must swiftly enable and safeguard their digital trust with their stakeholders through their own respective means.
In terms of legal and policy measures, due to the evolving landscape of generative AI -- and as part of the governance and AI RMF proposed by NIST -- organizations need to know the context and risks AI systems pose, while continuously reviewing existing and applicable laws and regulations.
Organizations also need to identify touchpoints where generative AI could be used internally or by external stakeholders. This can be done through collaborative efforts, such as the AI RMF working group. Such assessments are crucial for the next step in which organizations need to assess whether generative AI introduces new risks or regulatory obligations.
If there is going to be a delay in communications from relevant authorities, it is crucial for organizations to establish campaigns and educational initiatives to raise awareness internally, as well as within their stakeholder communities, about the opportunities, risks and responsibilities associated with generative AI content.
About the author
Goh Ser Yoong is an IT and cybersecurity professional with various years of experience, comprising both commercial and consulting perspective in information security, compliance and risk management, as well as fraud. Prior to that, Ser Yoong held positions in Standard Chartered, British American Tobacco and PwC with a strong focus on being a small to medium-sized enterprise on fraud and cybersecurity, as well as information security risk and compliance.
Ser Yoong graduated from Putra Business School with an MBA and holds a B.S. in information systems and management with First Class Honours from University of London (London School of Economics). He is a CISA, CISM, CISSP, CGEIT and CDPSE.
He has both spoken and organized conferences, as well as participated in security roundtables on the areas of cybersecurity, information security, IT auditing and governance. Besides serving as one of the student ambassadors for the University of London since graduation and on various boards, such as ISACA and Cloud Security Alliance, Ser Yoong is also actively mentoring on various platforms and with communities.