The ethics of using AI in healthcare
AI in healthcare demands ethical vigilance, balancing powerful innovation with medicine's core promise: Do no harm. Learn what it takes to deploy AI in healthcare responsibly.
The Greek physician and philosopher Hippocrates ushered in the concepts of modern medicine, establishing medicine as a science based on careful observation of patient symptoms and empirical data rather than superstition. But Hippocrates did more than simply separate medicine from magic; he is credited with adding ethics to the science of medicine, as laid out in the Hippocratic Oath, which states, in part, "First, do no harm." Roughly 2,500 years later, physicians take the Hippocratic Oath as a pledge to care for patients, ease suffering, wisely use their knowledge and judgment, and avoid causing harm.
Modern physicians now have an extraordinary array of tools and technologies at their disposal, and the dramatic rise of artificial intelligence (AI) promises powerful new analytical and diagnostic capabilities. AI is an advanced computing technology that can process vast amounts of medical data, drawing on the collective knowledge of countless physicians and practitioners across continents and centuries. AI technologies used in healthcare have the potential to render earlier, faster, more accurate diagnoses; perform real-time patient monitoring; and predict pathways to the most effective drug and procedural treatments to achieve superior patient care.
But the rush to embrace technology and bring AI systems into the healthcare mainstream carries serious ethical concerns. Sensitive user data in other industries (such as social media) is routinely collected and used to shape advertising, affect public opinion and generate revenue -- often without permission from the users involved and with little regard for how these disclosures might affect them.
Consequently, the healthcare industry faces critical ethical considerations when using AI systems. First, it is responsible for ensuring that sensitive patient data is protected in accordance with prevailing regulatory obligations, such as patient data privacy standards. Second, it is responsible for ensuring that patient data is used appropriately so that healthcare providers and AI systems "do no harm."
This article is part of
AI in healthcare: A guide to improving patient care with AI
- Which also includes:
- Health IT (health information technology)
- precision medicine (PM)
- telehealth (telemedicine)
Why do ethics matter when using AI in healthcare?
Ethical issues related to the use of AI in healthcare carry both technical and moral implications. To do their jobs, professional healthcare practitioners use extensive knowledge, which in turn must be applied fairly and equitably for the benefit of all patients. AI is far from perfect, so providers must take care to apply ethical constructs to AI use. Ethical matters in the AI application of medical knowledge focus on three principal areas.
1. Accuracy
AI systems can sift enormous amounts of data to find trends and proffer diagnoses much faster than human practitioners, but they must be trained using examples of known conditions, as AI can only know what it's taught. Further, the correctness of AI conclusions must be reinforced and optimized by feedback from practitioners. AI makes mistakes and has the potential to make up false conclusions -- a phenomenon called AI hallucination. This means AI assistance in healthcare should never be taken at face value. Its information can save lives, but its conclusions should always be examined and validated by human experts.
2. Fairness
AI conclusions are only as good as the underlying data. Unfortunately, data is often imperfect, with flaws that include incomplete, inaccurate and biased data. These flaws can negatively affect AI decision-making, lowering the accuracy of its conclusions -- particularly if the data underrepresents patients based on social class, race, gender, religion, sexual orientation or disabilities. This makes data quality and bias mitigation central issues in AI development and training.
3. Security
AI is noted for its ability to access and process enormous amounts of data and to act upon its conclusions with a startling amount of autonomy. This puts tremendous pressure on data security needed to safeguard sensitive patient data from illegitimate or inappropriate access -- by the AI as well as AI users -- and to ensure that data used to train AI systems is anonymized properly so that conditions and outcomes cannot be coupled to specific patients. Security issues are partly the domain of IT professionals, but dealing with AI security requires a holistic approach that embraces practitioners, administrators and researchers. Also, it must be reinforced with ongoing awareness training and policy development.
Ethical considerations when using AI in healthcare
There are four key ethical considerations for ensuring AI-driven healthcare businesses use AI tools wisely and for the benefit of patients. Here's how to address them.
Creating and enforcing ethics policies
Detailed policy frameworks are needed to guide the use of AI systems, translating AI recommendations into clinical practice and ensuring AI transparency and explainability. Policies emphasize the ethical use of AI technology to benefit patients while preventing patient harm or malfeasance. Such policies often include discussions of factors such as patient consent, equal access to care, AI errors, AI bias and outright AI system misuse. Policies sometimes include discussions of patient contact to ensure that AI use does not dehumanize patients and that individual AI recommendations are both accurate and reliable before they are enacted.
Maintaining data security and patient privacy
AI can access and process bewildering amounts of data, which must be stored and identified. With strong regulatory and legislative statutes already in place to safeguard patients' personally identifiable information, ethical requirements will include factors such as getting patient consent regarding how data is collected and used, collecting minimal data, following data storage and security protocols (such as end-to-end encryption), using identity and access management (IAM) tools and implementing data backup and disaster recovery measures. Ethical considerations should also explore ways to prevent and mitigate events such as unauthorized AI system use.
Applying human oversight to AI recommendations
It's easy to simply trust the AI system and implement its outcomes, but blind trust in automation -- especially in systems where errors and biases are present -- can have devastating consequences for patients and healthcare organizations. Ethical considerations will detail the need and application of human oversight at several levels. For example, practitioners should review AI recommendations for accuracy and validate the AI decisions by double-checking the explainability of the outcome and considering subjective issues such as patient preferences and values. Further, there should be careful consideration of liability and other legal concerns that affect the entire AI system chain, including AI system developers, AI trainers, clinicians, administrators and other staff. Regular training in the proper and acceptable use of AI tools is part of this consideration.
Ensuring a positive patient experience
Ethics also extends to patient treatment at several levels, including having empathy and sensitivity in information-gathering, recognizing and respecting patient preferences and values, and following up on patient outcomes as well as perceived quality of care. Part of patient involvement also includes clear and concise patient consent, which delineates the information collected, why it's needed, and how it's used -- including further AI training, if needed -- and allowing patients to opt out of certain data uses.
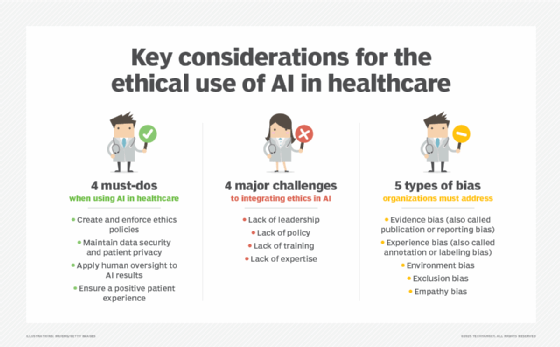
Challenges of ethical integrations
Ethics are central to all types of healthcare practices, but integrating ethical concerns into AI systems can present several broad challenges for organizations, including four major obstacles outlined below.
1. Lack of leadership
A core problem with AI and ethics is the notion that ethical issues are addressed as a native part of the AI platform -- in other words, that simply using an AI platform will correlate to ethical practices. This is not the case. AI systems have no automatic ethical or moral direction and will perform in any way they are utilized. Consequently, the ethical use of AI must start at the top of the healthcare organization, with senior leadership recognizing the need for adherence to ethical standards and issuing the mandate for ethical use of AI systems.
Healthcare leadership seeking a successful integration of ethics and AI will typically focus on understanding the risks of AI related to factors including data security, data ownership, data quality, data bias, informed patient consent, accountability and liability. Once the salient risks are understood, the leadership team can craft policies to guide the implementation and use of AI systems.
2. Lack of policies
Ethical use of AI in healthcare relies on carefully considered policies that address issues such as data security and patient data protection, data retention, clinical validation standards (i.e., ensuring that the AI is correct in its conclusions), data quality and bias mitigation. Policies lay out the rules for using AI systems and their underlying data in accordance with ethical healthcare standards. Policies also help ensure compliance with regulatory and liability obligations.
However, healthcare organizations often fall short when developing, maintaining and educating practitioners on those policies. Absent or incomplete policies lead to unacceptable AI use, put sensitive patient data at risk and potentially expose the organization to regulatory or legal jeopardy.
3. Lack of training
Establishing governance policies around ethical AI use has little value if those policies are not communicated and reinforced across the healthcare organization. Just as everyday businesses establish data security or acceptable use policies and provide regular training to staff, so healthcare organizations must translate AI ethics policies into practical training that can serve new and veteran employees.
Simply providing employees with a copy of the policy isn't enough -- the risks to the healthcare organization (and its patients) are simply too great. The organization must design and implement meaningful AI ethics training and make that training mandatory for all clinical and administrative staff.
4. Lack of expertise
Implementing AI ethical standards in a healthcare setting can be daunting, requiring extensive knowledge of AI systems and detailed insight into the organization's computing infrastructure. For example, IT must implement the mechanisms needed to protect stored data (such as IAM or data encryption); data scientists must work diligently to ensure data quality and mitigate bias for both training and practical usage; and practitioners must recognize and protect sensitive patient data. In addition, AI system experts must ensure transparency and explainability in the AI system to demonstrate comprehensive understanding of AI behavior.
All of this demands an expert staff that understands and supports ethical integration efforts with AI systems. A gap in expertise, such as a lack of explainability in AI system behavior, can compromise AI ethics initiatives and put the healthcare organization at risk.
The risks of AI bias in healthcare
AI operates by processing data against a series of algorithms that have been trained in advance using example data. Unfortunately, the same types of bias experienced by human decision-makers can also be conveyed to an AI system through the design of the algorithms and the data sets used to prepare those algorithms for production. While bias can be detrimental in any AI platform, bias in AI can have profound effects on entire patient demographics by under-representing potential conditions or offering suboptimal patient outcomes.
A primary focus of any AI system development is the identification and mitigation of system bias by measures such as collecting comprehensive data from diverse and highly inclusive sources. Common types of AI bias include the following:
- Evidence bias (also known as publication or reporting bias). Ideally, AI data is objective and transparent, but evidence bias occurs when external factors work to skew data that eventually feeds AI systems. For example, funded research can produce bias as outcomes might skew in favor of funding sources. The same impact occurs when research seeks positive results, sometimes resulting in data skew that favors positive results.
- Experience bias (also known as annotation or labeling bias). Data science and clinical professionals can introduce inconsistent or incorrect data labeling and classifications, essentially introducing undesirable skew into training data as well as algorithm training and tuning tasks. Even clinicians responsible for patient data classification can inadvertently cause such bias.
- Environment bias. This is a variation of exclusion bias where social, physical and environmental factors related to the data sets are not collected and included to add vital context to patient data. For example, environmental bias can occur when details such as residence location, living conditions, income and education are overlooked in the total data set.
- Exclusion bias. Data collection and processing are systematically ignored or underrepresented. For example, if data that pertains to a specific patient demographic is omitted from the total data set, the AI will provide poorer performance for those patients. This can lead to outcomes such as missed or incorrect diagnoses and erroneous treatment recommendations.
- Empathy bias. Empathy bias can occur when subjective, emotional considerations -- often qualitative information that is almost impossible to quantify -- are omitted from data sets and AI training. This prevents the AI from bringing context and nuance to its decision-making and its consideration of the unique needs of patients and groups. For example, not including patient preferences, such as end-of-life wishes, and moral or cultural values can drive inappropriate recommendations.
There are three strategies used to address AI bias. First, ensure that data sets represent diverse and inclusive sources with extensive examples and variabilities. Second, ensure explainability so that AI decision-making is well understood and trustworthy. And third, use comprehensive monitoring to gauge AI outcomes and look for bias over time as the AI system continues to receive new data and learn.
Stephen J. Bigelow, senior technology editor at Informa TechTarget, has more than 30 years of technical writing experience in the PC and technology industry.