AI existential risk: Is AI a threat to humanity?
What should enterprises make of the recent warnings about AI's threat to humanity? AI experts and ethicists offer opinions and practical advice for managing AI risk.
Rapid innovation in AI has fueled debate among industry experts about the existential threat posed by machines that can perform tasks previously done by humans.
Doomsayers argue that artificial general intelligence -- a machine that's able to think and experience the world like a human -- will happen sooner than expected and will outwit us. Shorter term, they warn our overreliance on AI systems could spell disaster: Disinformation will flood the internet, terrorists will craft new dangerous and cheap weapons, and killer drones could run rampant.
Even Geoffrey Hinton, widely seen as the "godfather of AI" for his seminal work on neural networks, has expressed growing concerns over AI's threat to humanity, issuing a warning in May about the rapidly advancing abilities of generative AI chatbots, like ChatGPT. "Right now, they're not more intelligent than us, as far as I can tell. But I think they soon may be," he told the BBC, forecasting a span of five to 10 years before this happens, as opposed to his previous timeline of 30 to 50 years.
Rising concerns about AI's existential risks have led to a call for a six-month moratorium on AI research -- an AI pause -- promulgated through an open letter signed by many industry and academic experts, including executives at many companies fueling AI innovation.
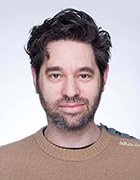
Others argue, however, that this AI doomerism narrative distracts from more likely AI dangers enterprises urgently need to heed: AI bias, inequity, inequality, hallucinations, new failure modes, privacy risks and security breaches. A big concern among people in this group is that a pause might create a protective moat for major AI companies, like OpenAI, maker of ChatGPT and an AI pause advocate.
"Releasing ChatGPT to the public while calling it dangerous seems little more than a cynical ploy by those planning to capitalize on fears without solving them," said Davi Ottenheimer, vice president of trust and digital ethics at Inrupt. He noted that a bigger risk may lie in enabling AI doomsayers to profit by abusing our trust.
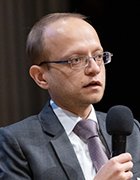
Responsible AI or virtue signaling?
Signed letters calling for an AI pause get a lot of media play, agreed Abhishek Gupta, founder and principal researcher at the Montreal AI Ethics Institute, but they beg the question of what happens next.
"I find it difficult to sign letters that primarily serve as virtue signaling without any tangible action or the necessary clarity to back them up," he said. "In my opinion, such letters are counterproductive as they consume attention cycles without leading to any real change."
Doomsday narratives confuse the discourse and potentially put a lid on the kind of levelheaded conversation required to make sound policy decisions, he said. Additionally, these media-fueled debates consume valuable time and resources that could instead be used to gain deeper understanding of AI use cases.
"For executives seeking to manage risks associated with AI effectively, they must first and foremost educate themselves on actual risks versus falsely presented existential threats," Gupta said. They also need to collaborate with technical experts who have practical experience in developing production-grade AI systems, as well as with academic professionals who work on the theoretical foundations of AI.
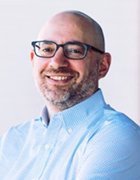
Building consensus on what needs to be tackled to ensure responsible AI programs should not be that hard, according to Eliott Behar, technology and human rights lawyer at Eliott Behar Law and former security counsel at Apple.
"Most people seem to agree that the existing state of big tech is problematic and that the way these companies are using data is somewhere at the heart of the problem," Behar said. What's required, he added, is a greater focus on how to let users see, understand and exercise control over how their data gets processed.
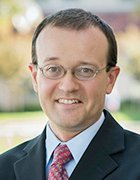
What are realistic AI risks?
If AI doomerism is not likely to prove useful in controlling AI risks, how should enterprises be thinking about the problem? Brian Green, director of technology ethics at the Markkula Center for Applied Ethics at Santa Clara University, said it's helpful to frame AI risks as those that come from the AI itself and risks that come from the use of AI by humans.
Risks from the AI itself range from simple errors in computation that lead to bad outcomes to AI gaining a will of its own and deciding to attack humankind, said Green, author of Ethics in the Age of Disruptive Technologies: An Operational Roadmap, a newly published handbook that lays out what he considers practical steps organizations can take to make ethical decisions. (He stressed that, at present, there is no clear way by which the latter could happen.)
The human use of AI covers everything humans can imagine could be automated and made more efficiently evil with AI: more centralized or hair-trigger control of nuclear weapons, more powerful disinformation campaigns, deadlier biological weapons, more effective planning for social control and so on.
"Everything horrible that human intelligence can do, artificial intelligence might be programmed to do as well as or better than humans," Green said. "There are vastly more chances that humans might use AI for existentially risky purposes than there are chances that AI would just pursue these goals on its own."
Green believes that the realistic AI existential risks we face are more mundane: AI-generated content trained to catch our attention that inadvertently blinds us to important issues or AI-based marketing apps trained to lure us into buying products and services that are detrimental to our well-being.
"I would argue that both of these things are already happening, so this possible existential AI risk is already upon us and is, therefore, 100% real," Green said.
It's important to keep on top of known problems, such as AI bias and misalignment with organizational objectives, he said. "Immediate problems that are ignored can turn into big problems later, and conversely, it is easier to solve big problems later if you first get some practice solving problems now."
Could AI stir up social unrest by displacing workers?
How AI technology is changing the nature of work is one of those issues companies should be focusing on now, according to Andrew Pery, AI ethics evangelist at Abbyy, an intelligent automation company.
"With the commercialization of generative AI, the magnitude of labor disruption could be unprecedented," he said, referring to a Goldman Sachs report predicting that generative AI could expose the equivalent of 300 million full-time jobs to automation.
"Such a dramatic displacement of labor is a recipe for growing social tensions by shifting millions of people to the margins of society with unsustainable unemployment levels and without the dignity of work that gives us meaning," Pery said. This may, in turn, give rise to more nefarious and dangerous uses of generative AI technology that subvert the foundations of a rule-based order, he added.
Fostering digital upskilling for new jobs and rethinking social safety net programs will play a pivotal role in safely transitioning into an age of AI, he said.
How enterprises can manage AI risks
A key component of responsible AI is identifying and mitigating risks that could arise from AI systems, Gupta said. These risks can manifest in various forms, including but not limited to data privacy breaches, biased outputs, AI hallucinations, deliberate attacks on AI systems, and concentration of power in compute and data.
Gupta recommended enterprises and stakeholders take a holistic and proactive approach that considers the potential impact of each AI risk across different domains and stakeholders to prioritize these risk scenarios effectively. This requires a deep understanding of AI systems and their algorithmic biases, the data inputs used to train and test the models, and the potential vulnerabilities and attack vectors that hackers or malicious actors may exploit.
AI risk heat map
A practical approach may be to apply the same methods used in cybersecurity, that is, evaluating various risks according to their probability and severity of impact. Many risks have not been identified yet, so Gupta recommended distinguishing between areas of uncertainty and risk. Uncertainty considers the unknown unknowns, while risk refers to assessment based on known unknowns.
Trustworthy AI pledge
Pery suggested that enterprises make a top-down organizational commitment to trustworthy AI principles and guidelines. Trustworthy AI includes human-centered values of fairness of AI outcomes, accuracy, integrity, confidentiality, security, accountability and transparency associated with the use of AI.
Organizations that offer frameworks for trustworthy AI include the following:
- Organization for Economic Cooperation and Development.
- Berkman Klein Center for Internet & Society.
- Stanford Center for Human-Centered Artificial Intelligence.
- AI Now Institute.
In addition, the NIST AI Risk Management Framework provides a comprehensive roadmap for implementing responsible AI best practices and a model for mitigating potential AI harms. Other standards that organizations might consider include the ISO/IEC 23894 framework and the EU-sponsored AI governance framework by the European Committee for Standardization and the European Committee for Electrotechnical Standardization.
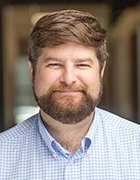
Checklist of questions for monitoring AI
Enterprises should institute measures for human oversight that ensure continuous monitoring of AI system performance. Measures can include identifying potential deviations from expected outcomes, taking remediation steps to correct adverse outcomes and including processes for overriding automated decisions by AI systems.
Nick Amabile, CEO at DAS42, a data and analytics consultancy, said putting the right people and processes around data governance, data literacy, training and enablement is important. It's helpful to consider how existing tools for governing the security and privacy of data could be extended to manage the AI algorithms trained on this data.
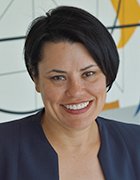
Kimberly Nevala, strategic advisor at SAS, recommended companies spend quality time considering questions such as the following:
- How will and could this solution go astray or make errors?
- Is intentional misuse probable and in what circumstances?
- How might the system be inadvertently misunderstood or misapplied?
- What are the impacts, and how do they scale?
- Does the system's design exacerbate or attenuate the potential for misuse and misunderstanding?
- How might this system be integrated into or influence others within and beyond our scope of control? What might be the second- or third-order effects thereof?
Will AI regulation help or harm?
Governments worldwide are starting to draft new AI regulations that may prevent some of the worst AI risks. Poorly drafted regulations may also slow the helpful adoption of AI applications that solve some of our most pressing problems in healthcare and sustainable development or create new problems.
"In many cases, I think regulation will stifle innovation and create a regulatory moat around incumbent companies and limit competition, innovation and disruption from startups," Amabile said.
Behar said that effective AI regulation requires being specific about the processes and requirements to make AI transparent, understandable and safe. "Drafting broad laws that focus essentially on whether a process is ultimately 'harmful' or not won't take us nearly far enough," he said.
According to Behar, discussions about regulating AI could take a cue from the regulation that helped us transition through the Industrial Revolution, including specifics like minimum safety standards for work conditions, minimum pay requirements, child labor restrictions and environmental standards. The AI equivalent would address how processes should be regulated, including how they use data; whether and how they drive decisions; and how we can ensure that their processes remain transparent, understandable and accountable.
Tackling existential AI risks will require identifying and addressing the present dangers of the AI systems we are deploying today, Nevala said.
"This is an issue that will only be addressed through a combination of public literacy and pressure, regulation and law and -- history sadly suggests-- after a yet-to-be-determined critical threshold of actual harm has occurred," Nevala said.