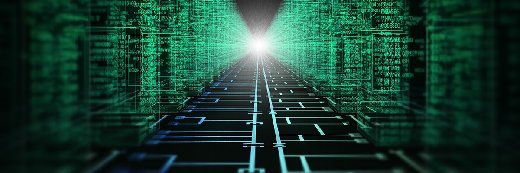
michelangelus - Fotolia
Choosing a modern data warehouse to fit your data needs
Choosing the right modern data warehouse for your enterprise data is a large task. Here are the key evaluation points to consider when choosing your platform.
Data warehouses are special-purpose platforms designed to ingest, store and facilitate the processing of large amounts of information. When I began designing data warehouses 30 years ago, the initial driver was to improve operational system performance by offloading reporting workloads to a separate platform.
As the reporting systems matured, users realized that they were able to use the new environments to transform raw data into actionable insights that business personnel could use to make better decisions. From front-line units to the executive team, the opportunities for all levels of business personnel to use information to improve organizational efficiency were -- and are now -- endless.
Now, big data platform usage ranges from shop floor robots that access modern data warehouses to improve their robotic actions to business personnel using increasingly intelligent analysis tools during their daily activities.
Why consider a data warehouse?
Modern data warehouses improve business intelligence by enhancing data quality and consistency, allowing users to better understand the meaning of the data, promoting a data-driven culture and facilitating historical intelligence and forecasting capabilities.
Before you begin evaluating the different platforms, it's important to learn about the different types of big data implementations. A modern data warehouse is only one of many platform options that may fit your organization's needs.
Choosing a data warehouse that fits your needs
Vendors of all sizes are capitalizing on the interest in business intelligence by offering a wealth of big data products to the IT community. A highly competitive market arena compels all modern data warehouse platform vendors to accelerate the release of new products and enhancements to existing offerings.
As a result, competitors frequently release features that expand their products' administration, data integration, metadata management, analytics and information governance capabilities. The latest trend is AI and machine learning augmented tools that assist human workers to collect, prepare and analyze big data and share business insights.
To correctly select and implement the most appropriate big data platform for their organizations, IT shops must create and execute a well-thought-out, detailed analysis of competing offerings. Because of the wide variety of cloud and on-premises architecture and data infrastructure combinations available, evaluation teams need to expand the scope of their analysis to include the modern data warehouse ecosystem.
Not only does the evaluation team need to understand the modern data warehouse product, team members must also learn the intricacies of the offering's underlying architecture. Choosing the correct large data store ecosystem -- which includes the type, platform, server, storage architecture, on-site or cloud infrastructure, data store and ancillary tool sets -- is critical to the success of any application that stores and processes large data volumes.
Data warehouse evaluation tips
- Follow a standardized product evaluation method to facilitate the selection process, which includes selecting the appropriate evaluation team, performing a thorough needs analysis and creating a set of weighted evaluation metrics. Use the evaluation metrics to create a vendor shortlist and execute a deep-dive comparison of those vendors.
- Understand your business needs. Are you looking for a purpose-built data warehouse that focuses on meeting a unique set of application requirements or a general-purpose platform that provides a much wider scope of features and functionality?
- What types of users will access your modern data warehouse -- citizen data scientists, executive management, sales teams, shop floor personnel or a combination of users? End user needs will play a significant role in platform and analysis tool selections.
- Are you looking for a vendor offering that supports the entire big data processing lifecycle from initial data collection to display or a multi-vendor, best-of-breed solution?
- What data cleansing and advanced data analytics capabilities does the product inherently provide?
- Prevent budgetary surprises by thoroughly evaluating how the vendor will charge you. Big data platform cost models range from simple software purchases to cloud-based systems that charge by query, storage and compute or elapsed processing time.
- Estimate initial and future data storage and workload volumes. How easy is it to scale the platform to accommodate increased data volumes and workloads?
- Visit vendor, peer review and big data discussion forum websites. Gartner's Peer Reviews website is an excellent place to learn how the IT community rates various vendor products. Vendors also often purchase distribution rights for Gartner's Magic Quadrants for data warehouses and make them available for the public to download.
- Depending on your preference, you will need to identify if the vendor supports cloud, on-premises or both environments.
- Does the platform provide a strong interface for system and user administration and platform monitoring?
- Is the platform able ingest a wide variety of data types?
- Does the platform support both batch and real-time data loads?
- What workload management capabilities does it provide?
- Is the product able to conform to organizational, industry-specific or governmental regulatory requirements?